#6.位置做smooth L1 loss
取正样本的位置上的值pos_idx做L1 loss
loc_p = loc_data[pos_idx].view(-1, 4)
loc_t = loc_t[pos_idx].view(-1, 4)
#loss_l tensor(14.0165, grad_fn=<SmoothL1LossBackward>)
loss_l = F.smooth_l1_loss(loc_p, loc_t, size_average=False)
#7. Compute max conf across batch for hard negative mining
这部分挺复杂的。简单来说就是难例挖掘
根据交叉熵计算出来的loss_c,把正样本位置填0,然后对loss_c从高到低排序,loss高的就是难样本,取3倍正样本的数量。
最后正样本和难例挖掘到的难样本做交叉熵
conf_p = conf_data[(pos_idx+neg_idx).gt(0)].view(-1, self.num_classes)
# pos [3,8732]
# neg [3,8732]
# conf_t [3,8732]
# targets_weighted [144]
targets_weighted = conf_t[(pos+neg).gt(0)] #只取True位置上的
#loss_c tensor(58.0656, grad_fn=<NllLossBackward>)
loss_c = F.cross_entropy(conf_p, targets_weighted, size_average=False)
~~~~下面是源码分析~~~~~~
~~~~下面是源码分析~~~~~~
~~~~下面是源码分析~~~~~~
def encode(matched, priors, variances):
"""Encode the variances from the priorbox layers into the ground truth boxes
we have matched (based on jaccard overlap) with the prior boxes.
Args:
matched: (tensor) Coords of ground truth for each prior in point-form
Shape: [num_priors, 4].
priors: (tensor) Prior boxes in center-offset form
Shape: [num_priors,4].
variances: (list[float]) Variances of priorboxes
Return:
encoded boxes (tensor), Shape: [num_priors, 4]
"""
# dist b/t match center and prior's center
g_cxcy = (matched[:, :2] + matched[:, 2:])/2 - priors[:, :2] ## [8732,2] 中心点偏移
# encode variance
g_cxcy /= (variances[0] * priors[:, 2:]) ## [8732,2] 中心点偏移 除以anchor的wh
# match wh / prior wh
g_wh = (matched[:, 2:] - matched[:, :2]) / priors[:, 2:] # [8732,2] gt的wh除以anchor的wh
g_wh = torch.log(g_wh) / variances[1]
# return target for smooth_l1_loss
return torch.cat([g_cxcy, g_wh], 1) # [num_priors,4]
matched的尺寸是[8732,4]
matcheds其实是groundtruth,格式是xmin,ymin,xmax,ymax。一般而言,一张图片比如有2个目标,为啥这里变8732个了呢?这个是match函数里面得到的。
具体的match函数里面讲解。大体就是每个预设的anchor(8732个)需要绑定一个gt。
以上,就是encode过程。就是把绑定的gt与anchor中心点和wh做偏移
def match(threshold, truths, priors, variances, labels, loc_t, conf_t, idx):
"""Match each prior box with the ground truth box of the highest jaccard
overlap, encode the bounding boxes, then return the matched indices
corresponding to both confidence and location preds.
Args:
threshold: (float) The overlap threshold used when mathing boxes.
truths: (tensor) Ground truth boxes, Shape: [num_obj, num_priors].
priors: (tensor) Prior boxes from priorbox layers, Shape: [n_priors,4].
variances: (tensor) Variances corresponding to each prior coord,
Shape: [num_priors, 4].
labels: (tensor) All the class labels for the image, Shape: [num_obj].
loc_t: (tensor) Tensor to be filled w/ endcoded location targets.
conf_t: (tensor) Tensor to be filled w/ matched indices for conf preds.
idx: (int) current batch index
Return:
The matched indices corresponding to 1)location and 2)confidence preds.
"""
# jaccard index
overlaps = jaccard(
truths, #[2,4]
point_form(priors) #[8732,4]
)#overlaps [2,8732] 计算每个gt与anchor的交并比
# (Bipartite Matching)
# [1,num_objects] best prior for each ground truth
best_prior_overlap, best_prior_idx = overlaps.max(1, keepdim=True)
# best_prior_overlap [2,1]
# best_prior_idx [2,1]
#best_prior_idx表示的是anchor的坐标索引,例如tensor([[8444],[5084]])
#意义就是每个gt与anchor的最大交并比和对应的anchor的位置。
#这个是以gt为主。每行取一个最大的。
# [1,num_priors] best ground truth for each prior
best_truth_overlap, best_truth_idx = overlaps.max(0, keepdim=True)
#best_truth_overlap [1,8732]
#best_truth_idx [1,8732]
#best_truth_idx 表示的是,如果有2个gt,那么这个里面的值取值范围是0-1.
#以anchor为主。每列取最大。
#意义就是每个anchor(8732)和gt最大交并比和每个anchor对应的gt。
best_truth_idx.squeeze_(0) # [1,8732] --> [8732]
best_truth_overlap.squeeze_(0) # [1,8732] --> [8732]
best_prior_idx.squeeze_(1) #[2,1] -->[2]
best_prior_overlap.squeeze_(1)#[2,1] -->[2]
best_truth_overlap.index_fill_(0, best_prior_idx, 2) # ensure best prior
#best_prior_idx表示的是gt与哪个anchor交并比最大。
# best_truth_overlap.index_fill_(0, best_prior_idx, 2)在与gt最大的anchor,赋值为2
# TODO refactor: index best_prior_idx with long tensor
# ensure every gt matches with its prior of max overlap
for j in range(best_prior_idx.size(0)):
best_truth_idx[best_prior_idx[j]] = j
#best_truth_idx 对应的位置赋值为gt的索引
matches = truths[best_truth_idx] # Shape: [num_priors,4]
conf = labels[best_truth_idx] + 1 # Shape: [num_priors]
conf[best_truth_overlap < threshold] = 0 # label as background threshold是0.5
loc = encode(matches, priors, variances) #loc [8732,4]
loc_t[idx] = loc # [num_priors,4] encoded offsets to learn
conf_t[idx] = conf # [num_priors] top class label for each prior | conf[8732]
#loc_t = torch.Tensor(num, num_priors, 4) 函数外面申请的,num是batchsize
#conf_t = torch.LongTensor(num, num_priors)
这里用图来表示。就是gt0最大的交并比是0.89,对应的anchor是2.
但是对于anchor2,其最大的交并比是0.9,对应的gt是1.
这种情况优先级最大的是分配gt与最大的anchor。确保gt与anchor交并比最大的留下。
那么,就
best_truth_overlap.index_fill_(0, best_prior_idx, 2) #确保gt与anchor最大的留下,并强制交并比为2
for j in range(best_prior_idx.size(0)):
best_truth_idx[best_prior_idx[j]] = j #对应anchor强制赋值对应的gt
意义就在于确保gt分配某个anchor,并且使得交并比最大为2。同时best_truth_idx强制赋值对应的gt。
总结下match这个函数功能就是把truth绑定到anchor上,相交的truth与anchor绑定,填充loc_t,conf_t传出。
下面给出整个ssd loss计算部分:
multibox_loss.py
class MultiBoxLoss(nn.Module):
"""SSD Weighted Loss Function
Compute Targets:
1) Produce Confidence Target Indices by matching ground truth boxes
with (default) 'priorboxes' that have jaccard index > threshold parameter
(default threshold: 0.5).
2) Produce localization target by 'encoding' variance into offsets of ground
truth boxes and their matched 'priorboxes'.
3) Hard negative mining to filter the excessive number of negative examples
that comes with using a large number of default bounding boxes.
(default negative:positive ratio 3:1)
Objective Loss:
L(x,c,l,g) = (Lconf(x, c) + αLloc(x,l,g)) / N
Where, Lconf is the CrossEntropy Loss and Lloc is the SmoothL1 Loss
weighted by α which is set to 1 by cross val.
Args:
c: class confidences,
l: predicted boxes,
g: ground truth boxes
N: number of matched default boxes
See: https://arxiv.org/pdf/1512.02325.pdf for more details.
"""
def __init__(self, num_classes, overlap_thresh, prior_for_matching,
bkg_label, neg_mining, neg_pos, neg_overlap, encode_target,
use_gpu=True):
super(MultiBoxLoss, self).__init__()
self.use_gpu = use_gpu
self.num_classes = num_classes
self.threshold = overlap_thresh
self.background_label = bkg_label
self.encode_target = encode_target
self.use_prior_for_matching = prior_for_matching
self.do_neg_mining = neg_mining
self.negpos_ratio = neg_pos
self.neg_overlap = neg_overlap
self.variance = cfg['variance']
def forward(self, predictions, targets):
"""Multibox Loss
Args:
predictions (tuple): A tuple containing loc preds, conf preds,
and prior boxes from SSD net.
conf shape: torch.size(batch_size,num_priors,num_classes)
loc shape: torch.size(batch_size,num_priors,4)
priors shape: torch.size(num_priors,4)
targets (tensor): Ground truth boxes and labels for a batch,
shape: [batch_size,num_objs,5] (last idx is the label).
loc_data [3,8732,4]
conf_data [3,8732,21]
priors [8732,4]
"""
loc_data, conf_data, priors = predictions
num = loc_data.size(0)
priors = priors[:loc_data.size(1), :]
num_priors = (priors.size(0))
num_classes = self.num_classes
# match priors (default boxes) and ground truth boxes
loc_t = torch.Tensor(num, num_priors, 4) #[3,8732,4]
conf_t = torch.LongTensor(num, num_priors) #[3,8732]
for idx in range(num):
truths = targets[idx][:, :-1].data
labels = targets[idx][:, -1].data
defaults = priors.data
match(self.threshold, truths, defaults, self.variance, labels,
loc_t, conf_t, idx)
**自我介绍一下,小编13年上海交大毕业,曾经在小公司待过,也去过华为、OPPO等大厂,18年进入阿里一直到现在。**
**深知大多数Linux运维工程师,想要提升技能,往往是自己摸索成长或者是报班学习,但对于培训机构动则几千的学费,着实压力不小。自己不成体系的自学效果低效又漫长,而且极易碰到天花板技术停滞不前!**
**因此收集整理了一份《2024年Linux运维全套学习资料》,初衷也很简单,就是希望能够帮助到想自学提升又不知道该从何学起的朋友,同时减轻大家的负担。**
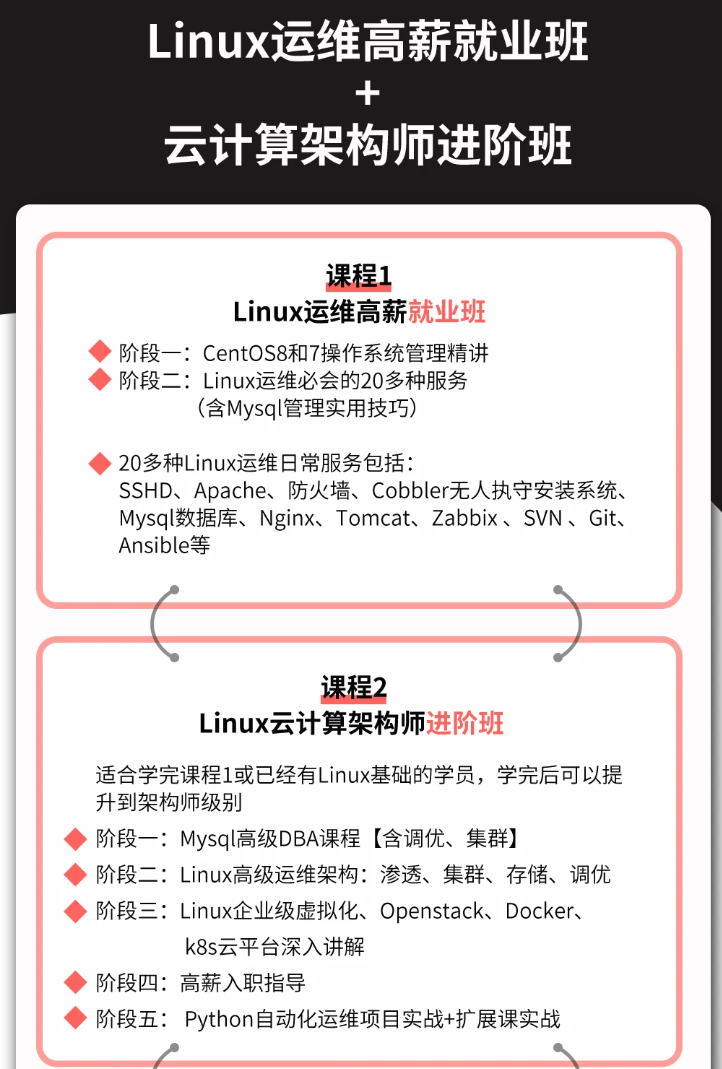
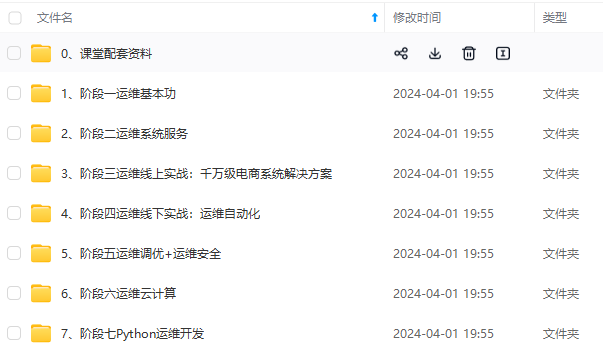
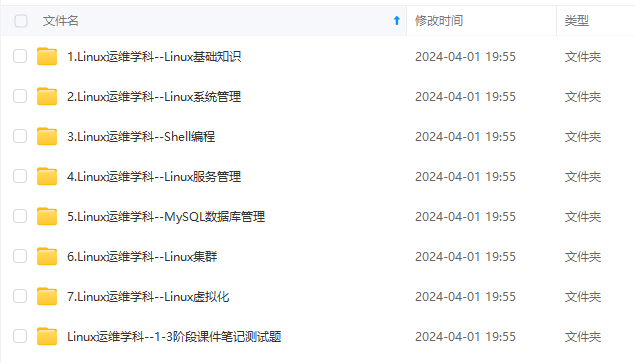
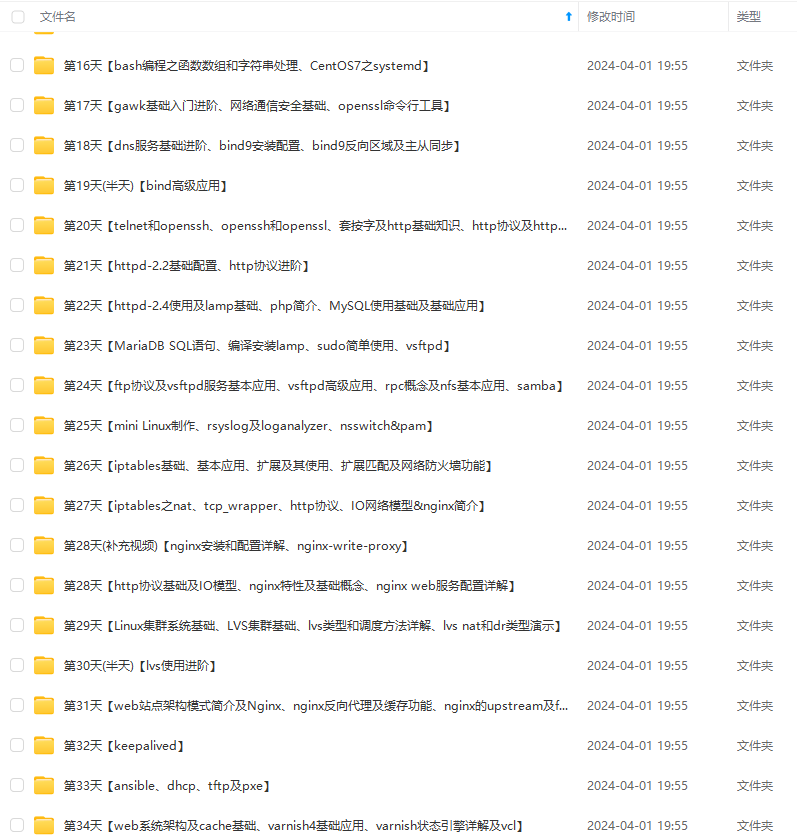
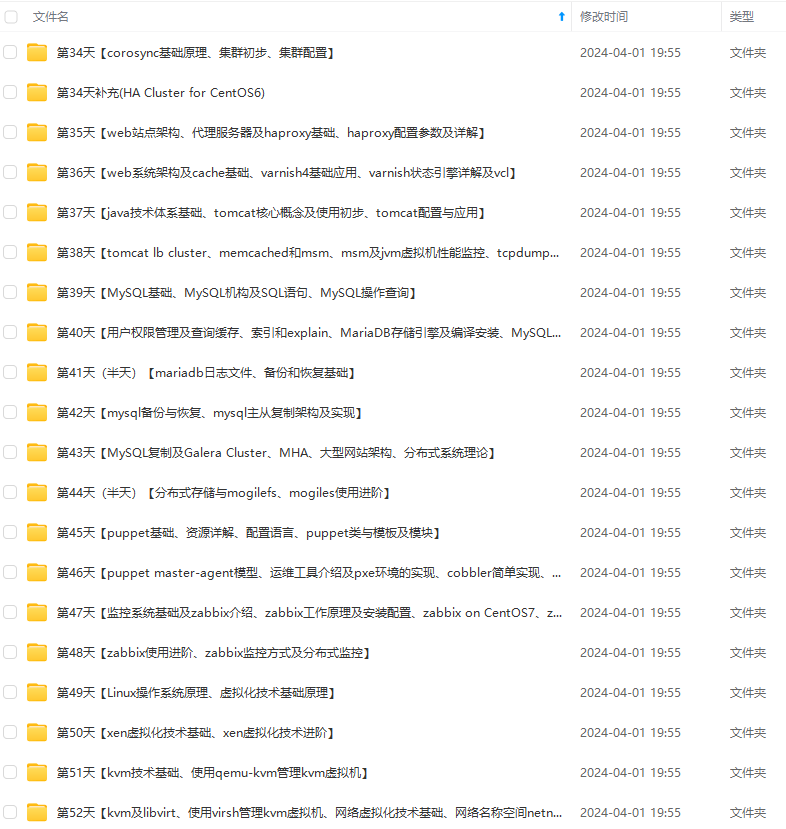
**既有适合小白学习的零基础资料,也有适合3年以上经验的小伙伴深入学习提升的进阶课程,基本涵盖了95%以上Linux运维知识点,真正体系化!**
**由于文件比较大,这里只是将部分目录大纲截图出来,每个节点里面都包含大厂面经、学习笔记、源码讲义、实战项目、讲解视频,并且后续会持续更新**
**如果你觉得这些内容对你有帮助,可以添加VX:vip1024b (备注Linux运维获取)**
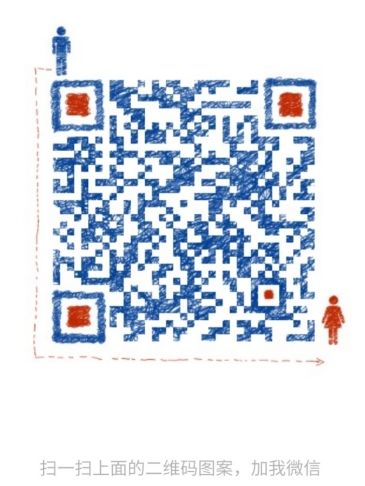
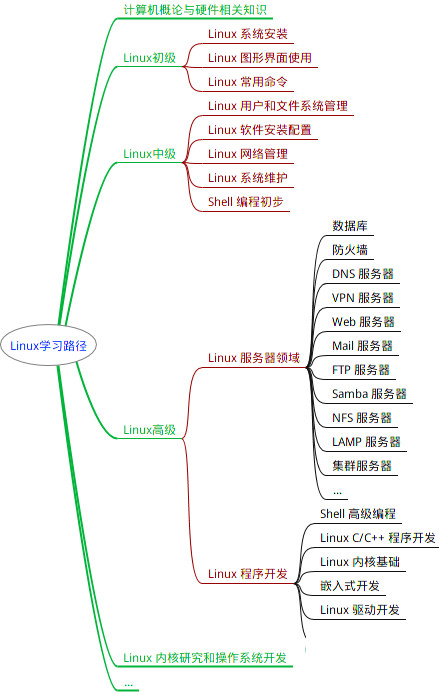
最全的Linux教程,Linux从入门到精通
======================
1. **linux从入门到精通(第2版)**
2. **Linux系统移植**
3. **Linux驱动开发入门与实战**
4. **LINUX 系统移植 第2版**
5. **Linux开源网络全栈详解 从DPDK到OpenFlow**
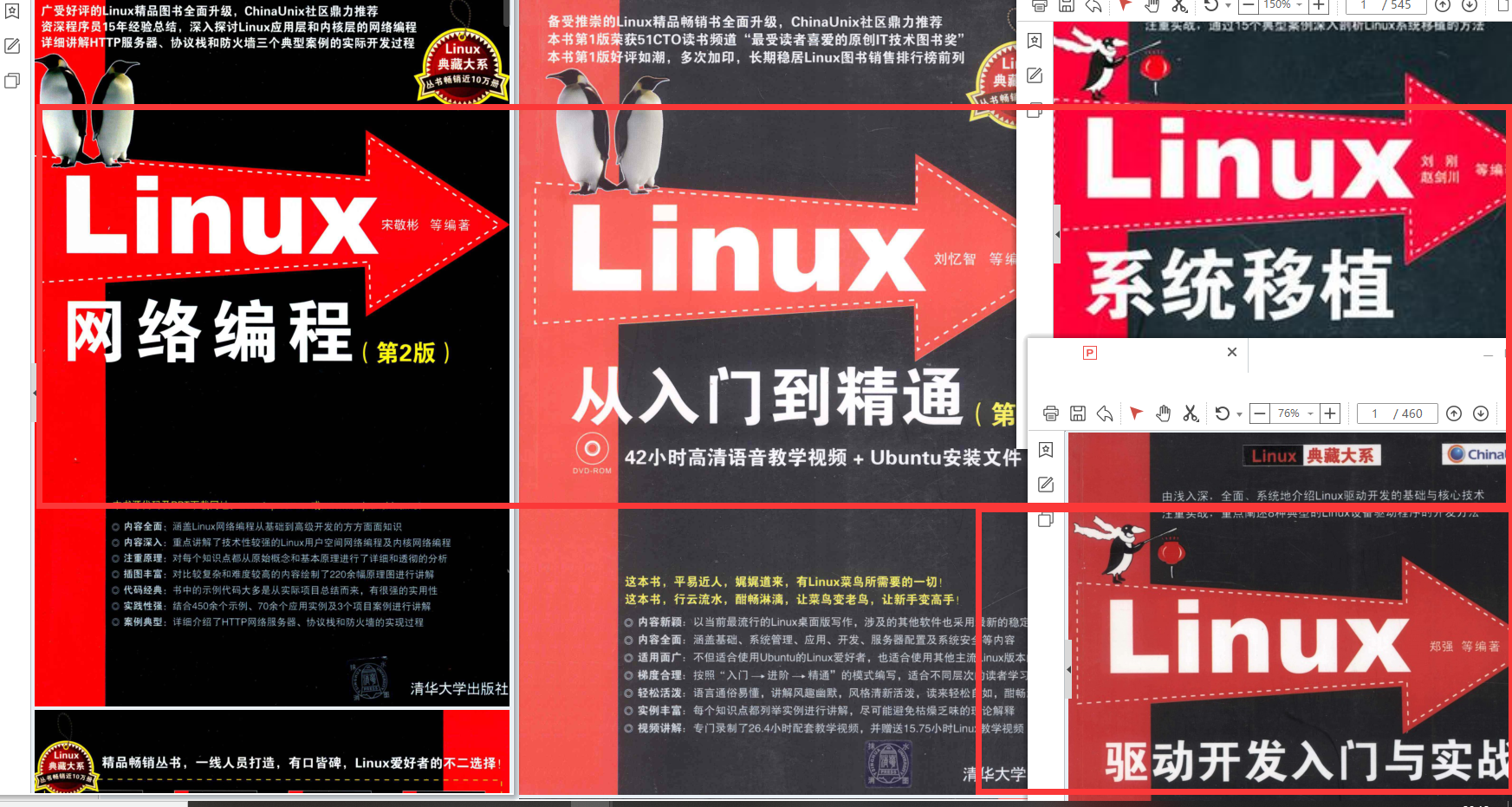
第一份《Linux从入门到精通》466页
====================
内容简介
====
本书是获得了很多读者好评的Linux经典畅销书**《Linux从入门到精通》的第2版**。本书第1版出版后曾经多次印刷,并被51CTO读书频道评为“最受读者喜爱的原创IT技术图书奖”。本书第﹖版以最新的Ubuntu 12.04为版本,循序渐进地向读者介绍了Linux 的基础应用、系统管理、网络应用、娱乐和办公、程序开发、服务器配置、系统安全等。本书附带1张光盘,内容为本书配套多媒体教学视频。另外,本书还为读者提供了大量的Linux学习资料和Ubuntu安装镜像文件,供读者免费下载。
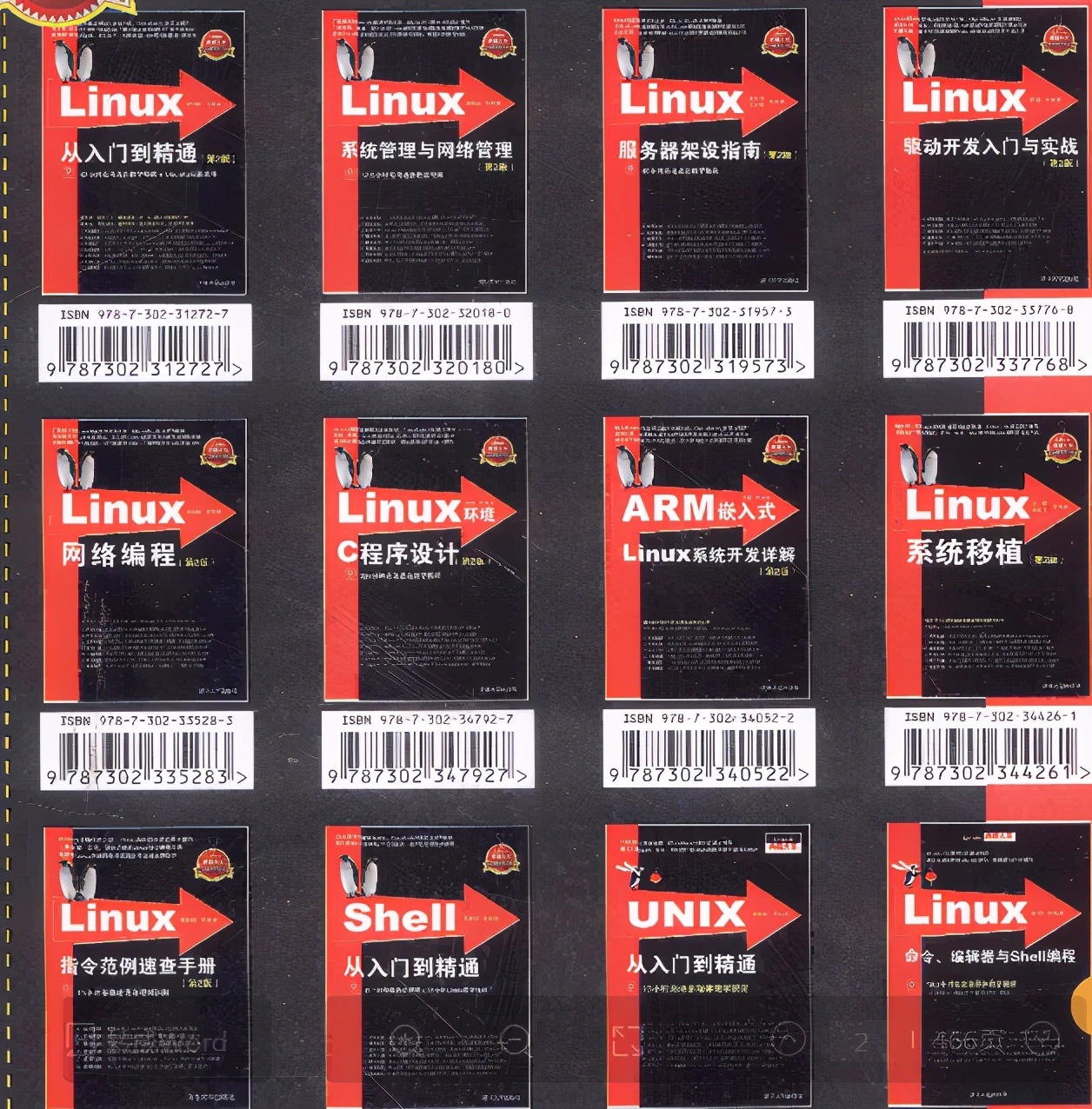
**本书适合广大Linux初中级用户、开源软件爱好者和大专院校的学生阅读,同时也非常适合准备从事Linux平台开发的各类人员。**
> 需要《Linux入门到精通》、《linux系统移植》、《Linux驱动开发入门实战》、《Linux开源网络全栈》电子书籍及教程的工程师朋友们劳烦您转发+评论
**一个人可以走的很快,但一群人才能走的更远。不论你是正从事IT行业的老鸟或是对IT行业感兴趣的新人,都欢迎扫码加入我们的的圈子(技术交流、学习资源、职场吐槽、大厂内推、面试辅导),让我们一起学习成长!**
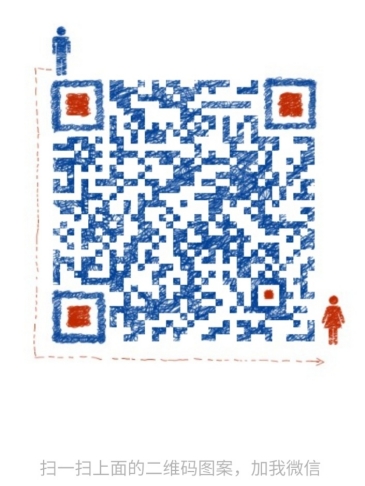
合广大Linux初中级用户、开源软件爱好者和大专院校的学生阅读,同时也非常适合准备从事Linux平台开发的各类人员。**
> 需要《Linux入门到精通》、《linux系统移植》、《Linux驱动开发入门实战》、《Linux开源网络全栈》电子书籍及教程的工程师朋友们劳烦您转发+评论
**一个人可以走的很快,但一群人才能走的更远。不论你是正从事IT行业的老鸟或是对IT行业感兴趣的新人,都欢迎扫码加入我们的的圈子(技术交流、学习资源、职场吐槽、大厂内推、面试辅导),让我们一起学习成长!**
[外链图片转存中...(img-c4yoEFQC-1712718620483)]