plt.show()
threshold_otsu(gray_image)
函数根据 Otsu
的二值化算法返回阈值。之后,使用此值构建二进制图像( dtype= bool
),最后应将其转换为 8 位无符号整数格式( dtype=uint8
)以进行可视化,使用img_as_ubyte()
函数完成转换过程。
程序输出如下图所示:
接下来,介绍下如何使用 scikit-image
中的一些其它阈值技术。
接下来,将对比 Otsu
、triangle
、Niblack
和 Sauvola
阈值技术进行阈值处理的不同效果。 Otsu
和 triangle
是全局阈值技术,而 Niblack
和 Sauvola
是局部阈值技术。当背景不均匀时,局部阈值技术则是更好的方法阈值处理方法。
同样的,第一步是导入所需的包:
from skimage.filters import threshold_otsu, threshold_triangle, threshold_niblack, threshold_sauvola
from skimage import img_as_ubyte
import cv2
import matplotlib.pyplot as plt
调用每个阈值方法( threshold_otsu()
、threshold_niblack()
、threshold_sauvola()
和 threshold_triangle()
),以使用 scikit-image
对比执行阈值操作:
Otsu
thresh_otsu = threshold_otsu(gray_image)
binary_otsu = gray_image > thresh_otsu
binary_otsu = img_as_ubyte(binary_otsu)
Niblack
thresh_niblack = threshold_niblack(gray_image, window_size=25, k=0.8)
binary_niblack = gray_image > thresh_niblack
binary_niblack = img_as_ubyte(binary_niblack)
Sauvola
thresh_sauvola = threshold_sauvola(gray_image, window_size=25)
binary_sauvola = gray_image > thresh_sauvola
binary_sauvola = img_as_ubyte(binary_sauvola)
triangle
thresh_triangle = threshold_triangle(gray_image)
binary_triangle = gray_image > thresh_triangle
binary_triangle = img_as_ubyte(binary_triangle)
程序输出如下图所示:
如上图所示,当图像不均匀时,局部阈值方法可以提供更好的结果。因此,可以将这些局部阈值方法应用于文本识别。
最后,我们了解一个更加有趣的阈值算法—— Multi-Otsu
阈值技术,其可用于将输入图像的像素分为多个不同的类别,每个类别根据图像内灰度的强度计算获得。
Multi-Otsu
根据所需类别的数量计算多个阈值,默认类数为3,此时将获得三个类别,算法返回两个阈值,由直方图中的红线表示。
import matplotlib
import numpy as np
import cv2
from skimage import data
from skimage.filters import threshold_multiotsu
image = cv2.imread(‘8.png’)
image = cv2.cvtColor(image, cv2.COLOR_BGR2GRAY)
使用默认值调用 threshold_multiotsu()
thresholds = threshold_multiotsu(image)
regions = np.digitize(image, bins=thresholds)
def show_img_with_matplotlib(img, title, pos, cmap):
ax = plt.subplot(1, 3, pos)
plt.imshow(img, cmap=cmap)
plt.title(title, fontsize=8)
plt.axis(‘off’)
fig, ax = plt.subplots(nrows=1, ncols=3, figsize=(10, 3.5))
绘制灰度图像
show_img_with_matplotlib(image, ‘Original’, 1, cmap=‘gray’)
可视化直方图
ax[1].hist(image.ravel(), bins=255)
ax[1].set_title(‘Histogram’)
for thresh in thresholds:
ax[1].axvline(thresh, color=‘r’)
可视化 Multi Otsu 结果
show_img_with_matplotlib(regions, ‘Multi-Otsu result’, 3, cmap=‘jet’)
plt.subplots_adjust()
plt.show()
可以通过修改 threshold_multiotsu
的 classes
参数来改变类别数,以观察不同效果:
将类别数修改为4
thresholds = threshold_multiotsu(image, classes=3)
regions = np.digitize(image, bins=thresholds)
scikit-image
也提供了其他更多的阈值技术,可以参阅 API 文档,以查看所有可用方法。
本文中,我们介绍了如何使用 scikit-image
中的不同阈值算法,包括两种全局阈值技术( Otsu
和 三角形二值算法)和两种局部阈值技术( Niblack
和 Sauvola
算法)。
自我介绍一下,小编13年上海交大毕业,曾经在小公司待过,也去过华为、OPPO等大厂,18年进入阿里一直到现在。
深知大多数Python工程师,想要提升技能,往往是自己摸索成长或者是报班学习,但对于培训机构动则几千的学费,着实压力不小。自己不成体系的自学效果低效又漫长,而且极易碰到天花板技术停滞不前!
因此收集整理了一份《2024年Python开发全套学习资料》,初衷也很简单,就是希望能够帮助到想自学提升又不知道该从何学起的朋友,同时减轻大家的负担。
既有适合小白学习的零基础资料,也有适合3年以上经验的小伙伴深入学习提升的进阶课程,基本涵盖了95%以上前端开发知识点,真正体系化!
由于文件比较大,这里只是将部分目录大纲截图出来,每个节点里面都包含大厂面经、学习笔记、源码讲义、实战项目、讲解视频,并且后续会持续更新
如果你觉得这些内容对你有帮助,可以扫码获取!!!(备注Python)
n/img_convert/9f49b566129f47b8a67243c1008edf79.png)
既有适合小白学习的零基础资料,也有适合3年以上经验的小伙伴深入学习提升的进阶课程,基本涵盖了95%以上前端开发知识点,真正体系化!
由于文件比较大,这里只是将部分目录大纲截图出来,每个节点里面都包含大厂面经、学习笔记、源码讲义、实战项目、讲解视频,并且后续会持续更新
如果你觉得这些内容对你有帮助,可以扫码获取!!!(备注Python)
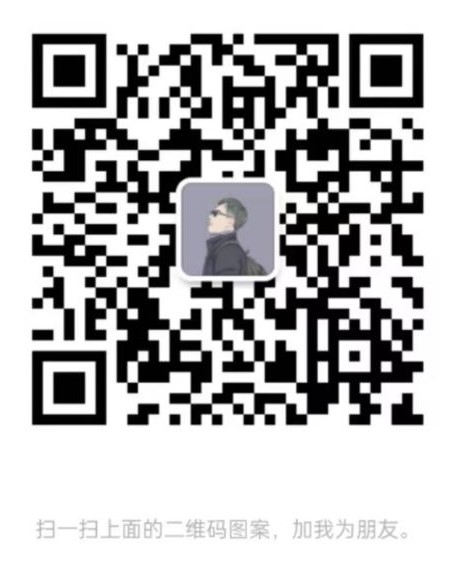