格式:
@staticmethod
from_tensor_slices(
tensors
)
迭代
我们可以调用iter
函数来生成迭代器.
格式:
iter(object[, sentinel])
参数:
-object: 支持迭代的集合对象
- sentinel: 如果传递了第二个参数, 则参数 object 必须是一个可调用的对象 (如, 函数). 此时, iter 创建了一个迭代器对象, 每次调用这个迭代器对象的
__next__()
方法时, 都会调用 object
例子:
list = [1, 2, 3]
i = iter(list)
print(next(i))
print(next(i))
print(next(i))
输出结果:
1
2
3
截断正态分布
truncated_normal
可以帮助我们生成一个截断的正态分布. 生成的正态分布值会在两倍的标准差的范围之内.
格式:
tf.random.truncated_normal(
shape, mean=0.0, stddev=1.0, dtype=tf.dtypes.float32, seed=None, name=None
)
参数:
- shape: 张量的形状
- mean: 正态分布的均值, 默认 0.0
- stddev: 正态分布的标准差, 默认为 1.0
- dtype: 数据类型, 默认为 float32
- seed: 随机数种子
- name: 数据名称
relu 激活函数
激活函数有 sigmoid, maxout, relu 等等函数. 通过激活函数我们可以使得各个层之间达成非线性关系.
激活函数可以帮助我们提高模型健壮性, 提高非线性表达能力, 缓解梯度消失问题.
one_hot
tf.one_hot
函数是讲 input 准换为 one_hot 类型数据输出. 相当于将多个数值联合放在一起作为多个相同类型的向量.
格式:
tf.one_hot(
indices, depth, on_value=None, off_value=None, axis=None, dtype=None, name=None
)
参数:
- indices: 索引的张量
- depth: 指定独热编码维度的标量
- on_value: 索引 indices[j] = i 位置处填充的标量,默认为 1
- off_value: 索引 indices[j] != i 所有位置处填充的标量, 默认为 0
- axis: 填充的轴, 默认为 -1 (最里面的新轴)
- dtype: 输出张量的数据格式
- name:数据名称
assign_sub
assign_sub
可以帮助我们实现张量自减.
格式:
tf.compat.v1.assign_sub(
ref, value, use_locking=None, name=None
)
参数:
- ref: 多重张量
- value: 张量
- use_locking: 锁
- name: 数据名称
准备工作
import tensorflow as tf
# 定义超参数
batch_size = 256 # 一次训练的样本数目
learning_rate = 0.001 # 学习率
iteration_num = 20 # 迭代次数
# 读取mnist数据集
(x, y), _ = tf.keras.datasets.mnist.load_data() # 读取训练集的特征值和目标值
print(x[:5]) # 调试输出前5个图
print(y[:5]) # 调试输出前5个目标值数字
print(x.shape) # (60000, 28, 28) 单通道
print(y.shape) # (60000,)
# 转换成常量tensor
x = tf.convert_to_tensor(x, dtype=tf.float32) / 255 # 转换为0~1的形式
y = tf.convert_to_tensor(y, dtype=tf.int32) # 转换为整数形式
# 调试输出范围
print(tf.reduce_min(x), tf.reduce_max(x)) # 0~1
print(tf.reduce_min(y), tf.reduce_max(y)) # 0~9
# 分割数据集
train_db = tf.data.Dataset.from_tensor_slices((x, y)).batch(batch_size) # 256为一个batch
train_iter = iter(train_db) # 生成迭代对象
# 定义权重和bias [256, 784] => [256, 256] => [256, 128] => [128, 10]
w1 = tf.Variable(tf.random.truncated_normal([784, 256], stddev=0.1)) # 标准差为0.1的截断正态分布
b1 = tf.Variable(tf.zeros([256])) # 初始化为0
w2 = tf.Variable(tf.random.truncated_normal([256, 128], stddev=0.1)) # 标准差为0.1的截断正态分布
b2 = tf.Variable(tf.zeros([128])) # 初始化为0
w3 = tf.Variable(tf.random.truncated_normal([128, 10], stddev=0.1)) # 标准差为0.1的截断正态分布
b3 = tf.Variable(tf.zeros([10])) # 初始化为0
输出结果:
[[[0 0 0 ... 0 0 0]
[0 0 0 ... 0 0 0]
[0 0 0 ... 0 0 0]
...
[0 0 0 ... 0 0 0]
[0 0 0 ... 0 0 0]
[0 0 0 ... 0 0 0]]
[[0 0 0 ... 0 0 0]
[0 0 0 ... 0 0 0]
[0 0 0 ... 0 0 0]
...
[0 0 0 ... 0 0 0]
[0 0 0 ... 0 0 0]
[0 0 0 ... 0 0 0]]
[[0 0 0 ... 0 0 0]
[0 0 0 ... 0 0 0]
[0 0 0 ... 0 0 0]
...
[0 0 0 ... 0 0 0]
[0 0 0 ... 0 0 0]
[0 0 0 ... 0 0 0]]
[[0 0 0 ... 0 0 0]
[0 0 0 ... 0 0 0]
[0 0 0 ... 0 0 0]
...
[0 0 0 ... 0 0 0]
[0 0 0 ... 0 0 0]
[0 0 0 ... 0 0 0]]
[[0 0 0 ... 0 0 0]
[0 0 0 ... 0 0 0]
[0 0 0 ... 0 0 0]
...
[0 0 0 ... 0 0 0]
[0 0 0 ... 0 0 0]
[0 0 0 ... 0 0 0]]]
[5 0 4 1 9]
(60000, 28, 28)
(60000,)
tf.Tensor(0.0, shape=(), dtype=float32) tf.Tensor(1.0, shape=(), dtype=float32)
tf.Tensor(0, shape=(), dtype=int32) tf.Tensor(9, shape=(), dtype=int32)
train 函数
def train(epoch): # 训练
for step, (x, y) in enumerate(train_db): # 每一批样本遍历
# 把x平铺 [256, 28, 28] => [256, 784]
x = tf.reshape(x, [-1, 784])
with tf.GradientTape() as tape: # 自动求解
# 第一个隐层 [256, 784] => [256, 256]
# [256, 784]@[784, 256] + [256] => [256, 256] + [256] => [256, 256] + [256, 256] (广播机制)
h1 = x @ w1 + tf.broadcast_to(b1, [x.shape[0], 256])
h1 = tf.nn.relu(h1) # relu激活
# 第二个隐层 [256, 256] => [256, 128]
h2 = h1 @ w2 + b2
h2 = tf.nn.relu(h2) # relu激活
# 输出层 [256, 128] => [128, 10]
out = h2 @ w3 + b3
# 计算损失MSE(Mean Square Error)
y_onehot = tf.one_hot(y, depth=10) # 转换成one_hot编码
loss = tf.square(y_onehot - out) # 计算总误差
loss = tf.reduce_mean(loss) # 计算平均误差MSE
# 计算梯度
grads = tape.gradient(loss, [w1, b1, w2, b2, w3, b3])
# 更新权重
w1.assign_sub(learning_rate * grads[0]) # 自减梯度*学习率
b1.assign_sub(learning_rate * grads[1]) # 自减梯度*学习率
w2.assign_sub(learning_rate * grads[2]) # 自减梯度*学习率
b2.assign_sub(learning_rate * grads[3]) # 自减梯度*学习率
w3.assign_sub(learning_rate * grads[4]) # 自减梯度*学习率
b3.assign_sub(learning_rate * grads[5]) # 自减梯度*学习率
if step % 100 == 0: # 每运行100个批次, 输出一次
print("epoch:", epoch, "step:", step, "loss:", float(loss))
run 函数
def run():
for i in range(iteration_num): # 迭代20次
train(i)
完整代码
import tensorflow as tf
# 定义超参数
batch_size = 256 # 一次训练的样本数目
learning_rate = 0.001 # 学习率
iteration_num = 20 # 迭代次数
# 读取mnist数据集
(x, y), _ = tf.keras.datasets.mnist.load_data() # 读取训练集的特征值和目标值
print(x[:5]) # 调试输出前5个图
print(y[:5]) # 调试输出前5个目标值数字
print(x.shape) # (60000, 28, 28) 单通道
print(y.shape) # (60000,)
# 转换成常量tensor
x = tf.convert_to_tensor(x, dtype=tf.float32) / 255 # 转换为0~1的形式
y = tf.convert_to_tensor(y, dtype=tf.int32) # 转换为整数形式
# 调试输出范围
print(tf.reduce_min(x), tf.reduce_max(x)) # 0~1
print(tf.reduce_min(y), tf.reduce_max(y)) # 0~9
# 分割数据集
train_db = tf.data.Dataset.from_tensor_slices((x, y)).batch(batch_size) # 256为一个batch
train_iter = iter(train_db) # 生成迭代对象
# 定义权重和bias [256, 784] => [256, 256] => [256, 128] => [128, 10]
w1 = tf.Variable(tf.random.truncated_normal([784, 256], stddev=0.1)) # 标准差为0.1的截断正态分布
b1 = tf.Variable(tf.zeros([256])) # 初始化为0
w2 = tf.Variable(tf.random.truncated_normal([256, 128], stddev=0.1)) # 标准差为0.1的截断正态分布
b2 = tf.Variable(tf.zeros([128])) # 初始化为0
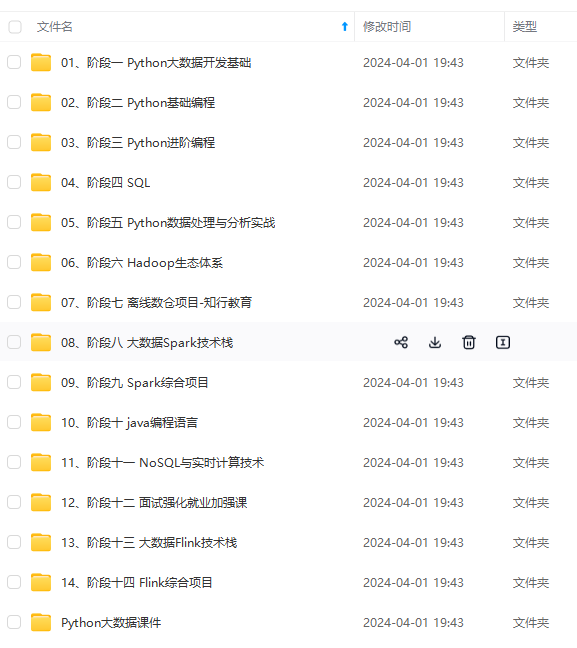
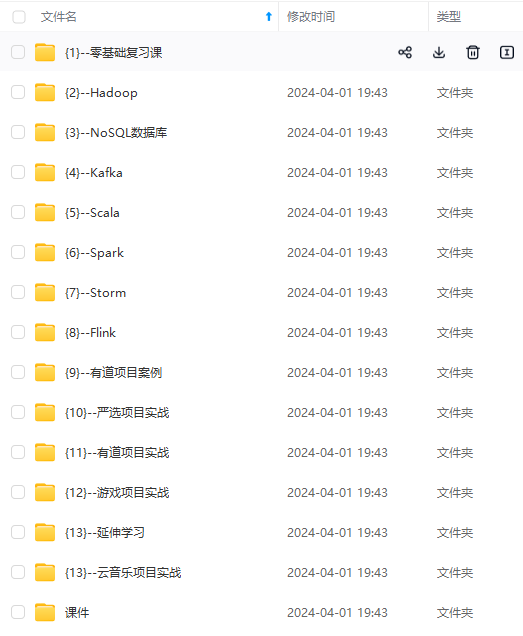
**网上学习资料一大堆,但如果学到的知识不成体系,遇到问题时只是浅尝辄止,不再深入研究,那么很难做到真正的技术提升。**
**[需要这份系统化资料的朋友,可以戳这里获取](https://bbs.csdn.net/topics/618545628)**
**一个人可以走的很快,但一群人才能走的更远!不论你是正从事IT行业的老鸟或是对IT行业感兴趣的新人,都欢迎加入我们的的圈子(技术交流、学习资源、职场吐槽、大厂内推、面试辅导),让我们一起学习成长!**
f.zeros([128])) # 初始化为0
[外链图片转存中...(img-2ot0o8Nx-1714784632401)]
[外链图片转存中...(img-P1jUmyy2-1714784632401)]
**网上学习资料一大堆,但如果学到的知识不成体系,遇到问题时只是浅尝辄止,不再深入研究,那么很难做到真正的技术提升。**
**[需要这份系统化资料的朋友,可以戳这里获取](https://bbs.csdn.net/topics/618545628)**
**一个人可以走的很快,但一群人才能走的更远!不论你是正从事IT行业的老鸟或是对IT行业感兴趣的新人,都欢迎加入我们的的圈子(技术交流、学习资源、职场吐槽、大厂内推、面试辅导),让我们一起学习成长!**