既有适合小白学习的零基础资料,也有适合3年以上经验的小伙伴深入学习提升的进阶课程,涵盖了95%以上Go语言开发知识点,真正体系化!
由于文件比较多,这里只是将部分目录截图出来,全套包含大厂面经、学习笔记、源码讲义、实战项目、大纲路线、讲解视频,并且后续会持续更新
}
}
public static void main(String[] args) {
Configuration conf=new Configuration();
try {
//args从控制台获取路径 解析得到域名
String[] paths=new GenericOptionsParser(conf,args).getRemainingArgs();
if(paths.length<2){
throw new RuntimeException("必須輸出 輸入 和输出路径");
}
//得到一个Job 并设置名字
Job job=Job.getInstance(conf,"wordcount");
//设置Jar 使本程序在Hadoop中运行
job.setJarByClass(WordCount.class);
//设置Map处理类
job.setMapperClass(WordCountMapper.class);
//设置map的输出类型,因为不一致,所以要设置
job.setMapOutputKeyClass(Text.class);
job.setOutputValueClass(IntWritable.class);
//设置Reduce处理类
job.setReducerClass(WordCountReducer.class);
//设置输入和输出目录
FileInputFormat.addInputPath(job, new Path(paths[0]));
FileOutputFormat.setOutputPath(job, new Path(paths[1]));
//启动运行
System.exit(job.waitForCompletion(true) ? 0:1);
} catch (IOException e) {
e.printStackTrace();
} catch (ClassNotFoundException e) {
e.printStackTrace();
} catch (InterruptedException e) {
e.printStackTrace();
}
}
}
## 二、通过 Hadoop 建立倒排索引
倒排索引就是根据单词内容来查找文档的方式,由于不是根据文档来确定文档所包含的内容,进行了相反的操作,所以被称为倒排索引, 它是搜索引擎最为核心的数据结构,以及文档检索的关键部分。
下面来看一个例子来理解什么是倒排索引
这里我准备了两个文件 分别为1.txt和2.txt
1.txt的内容如下
I Love Hadoop
I like ZhouSiYuan
I love me
---
2.txt的内容如下
I Love MapReduce
I like NBA
I love Hadoop
我这里使用的是默认的输入格式TextInputFormat,他是一行一行的读的,键是偏移量。
所以在map阶段之前的到结果如下
map阶段从1.txt的得到的输入
0 I Love Hadoop
15 I like ZhouSiYuan
34 I love me
map阶段从2.txt的得到的输入
0 I Love MapReduce
18 I like NBA
30 I love Hadoop
map阶段
把词频作为值
把单词和URI组成key值
比如
key : I+hdfs://192.168.52.140:9000/index/2.txt value:1
为什么要这样设置键和值?
因为这样设计可以使用MapReduce框架自带的map端排序,将同一单词的词频组成列表
经过map阶段1.txt得到的输出如下
I:hdfs://192.168.52.140:9000/index/1.txt 1
Love:hdfs://192.168.52.140:9000/index/1.txt 1
MapReduce:hdfs://192.168.52.140:9000/index/1.txt 1
I:hdfs://192.168.52.140:9000/index/1.txt 1
Like:hdfs://192.168.52.140:9000/index/1.txt 1
ZhouSiYuan:hdfs://192.168.52.140:9000/index/1.txt 1
I:hdfs://192.168.52.140:9000/index/1.txt 1
love:hdfs://192.168.52.140:9000/index/1.txt 1
me:hdfs://192.168.52.140:9000/index/1.txt 1
经过map阶段2.txt得到的输出如下
I:hdfs://192.168.52.140:9000/index/2.txt 1
Love:hdfs://192.168.52.140:9000/index/2.txt 1
MapReduce:hdfs://192.168.52.140:9000/index/2.txt 1
I:hdfs://192.168.52.140:9000/index/2.txt 1
Like:hdfs://192.168.52.140:9000/index/2.txt 1
NBA:hdfs://192.168.52.140:9000/index/2.txt 1
I:hdfs://192.168.52.140:9000/index/2.txt 1
love:hdfs://192.168.52.140:9000/index/2.txt 1
Hadoop:hdfs://192.168.52.140:9000/index/2.txt 1
1.txt经过MapReduce框架自带的map端排序得到的输出结果如下
I:hdfs://192.168.52.140:9000/index/1.txt list{1,1,1}
Love:hdfs://192.168.52.140:9000/index/1.txt list{1}
MapReduce:hdfs://192.168.52.140:9000/index/1.txt list{1}
Like:hdfs://192.168.52.140:9000/index/1.txt list{1}
ZhouSiYuan:hdfs://192.168.52.140:9000/index/1.txt list{1}
love:hdfs://192.168.52.140:9000/index/1.txt list{1}
me:hdfs://192.168.52.140:9000/index/1.txt list{1}
2.txt经过MapReduce框架自带的map端排序得到的输出结果如下
I:hdfs://192.168.52.140:9000/index/2.txt list{1,1,1}
Love:hdfs://192.168.52.140:9000/index/2.txt list{1}
MapReduce:hdfs://192.168.52.140:9000/index/2.txt list{1}
Like:hdfs://192.168.52.140:9000/index/2.txt list{1}
NBA:hdfs://192.168.52.140:9000/index/2.txt list{1}
love:hdfs://192.168.52.140:9000/index/2.txt list{1}
Hadoop:hdfs://192.168.52.140:9000/index/2.txt list{1}
combine阶段:
key值为单词,
value值由URI和词频组成
value: hdfs://192.168.52.140:9000/index/2.txt:3 key:I
为什么这样设计键值了?
因为在Shuffle过程将面临一个问题,所有具有相同单词的记录(由单词、URL和词频组成)应该交由同一个Reducer处理
所以重新把单词设置为键可以使用MapReduce框架默认的Shuffle过程,将相同单词的所有记录发送给同一个Reducer处理
combine阶段将key相同的value值累加
1.txt得到如下输出
I hdfs://192.168.52.140:9000/index/1.txt:3
Love hdfs://192.168.52.140:9000/index/1.txt:1
MapReduce hdfs://192.168.52.140:9000/index/1.txt:1
Like hdfs://192.168.52.140:9000/index/1.txt:1
ZhouSiYuan hdfs://192.168.52.140:9000/index/1.txt:1
love hdfs://192.168.52.140:9000/index/1.txt:1
me hdfs://192.168.52.140:9000/index/1.txt:1
2.txt得到如下输出
I hdfs://192.168.52.140:9000/index/2.txt:3
Love hdfs://192.168.52.140:9000/index/2.txt:1
MapReduce hdfs://192.168.52.140:9000/index/2.txt:1
Like hdfs://192.168.52.140:9000/index/2.txt:1
NBA hdfs://192.168.52.140:9000/index/2.txt:1
love hdfs://192.168.52.140:9000/index/2.txt:1
Hadoop hdfs://192.168.52.140:9000/index/2.txt:1
这样reducer过程就很简单了,它只用来生成文档列表
比如相同的单词I,这样生成文档列表
I hdfs://192.168.52.140:9000/index/2.txt:3;hdfs://192.168.52.140:9000/index/1.txt:3;
最后所有的输出结果如下
Hadoop hdfs://192.168.52.140:9000/index/1.txt:1;hdfs://192.168.52.140:9000/index/2.txt:1;
I hdfs://192.168.52.140:9000/index/2.txt:3;hdfs://192.168.52.140:9000/index/1.txt:3;
Love hdfs://192.168.52.140:9000/index/1.txt:1;hdfs://192.168.52.140:9000/index/2.txt:1;
MapReduce hdfs://192.168.52.140:9000/index/2.txt:1;
NBA hdfs://192.168.52.140:9000/index/2.txt:1;
ZhouSiYuan hdfs://192.168.52.140:9000/index/1.txt:1;
like hdfs://192.168.52.140:9000/index/1.txt:1;hdfs://192.168.52.140:9000/index/2.txt:1;
love hdfs://192.168.52.140:9000/index/2.txt:1;hdfs://192.168.52.140:9000/index/1.txt:1;
me hdfs://192.168.52.140:9000/index/1.txt:1;
下面是整个源代码
package com.hadoop.mapreduce.test8.invertedindex;
import java.io.IOException;
import java.util.StringTokenizer;
import org.apache.hadoop.conf.Configuration;
import org.apache.hadoop.fs.Path;
import org.apache.hadoop.io.Text;
import org.apache.hadoop.mapreduce.Job;
import org.apache.hadoop.mapreduce.Mapper;
import org.apache.hadoop.mapreduce.Reducer;
import org.apache.hadoop.mapreduce.lib.input.FileInputFormat;
import org.apache.hadoop.mapreduce.lib.input.FileSplit;
import org.apache.hadoop.mapreduce.lib.output.FileOutputFormat;
public class InvertedIndex {
/**
*
* @author 汤高
*
*/
public static class InvertedIndexMapper extends Mapper<Object, Text, Text, Text>{
private Text keyInfo = new Text(); // 存储单词和URI的组合
private Text valueInfo = new Text(); //存储词频
private FileSplit split; // 存储split对象。
@Override
protected void map(Object key, Text value, Mapper<Object, Text, Text, Text>.Context context)
throws IOException, InterruptedException {
//获得<key,value>对所属的FileSplit对象。
split = (FileSplit) context.getInputSplit();
System.out.println("偏移量"+key);
System.out.println("值"+value);
//StringTokenizer是用来把字符串截取成一个个标记或单词的,默认是空格或多个空格(\t\n\r等等)截取
StringTokenizer itr = new StringTokenizer( value.toString());
while( itr.hasMoreTokens() ){
// key值由单词和URI组成。
keyInfo.set( itr.nextToken()+":"+split.getPath().toString());
//词频初始为1
valueInfo.set("1");
context.write(keyInfo, valueInfo);
}
System.out.println("key"+keyInfo);
System.out.println("value"+valueInfo);
}
}
/**
*
* @author 汤高
*
*/
public static class InvertedIndexCombiner extends Reducer<Text, Text, Text, Text>{
private Text info = new Text();
@Override
protected void reduce(Text key, Iterable<Text> values, Reducer<Text, Text, Text, Text>.Context context)
throws IOException, InterruptedException {
//统计词频
int sum = 0;
for (Text value : values) {
sum += Integer.parseInt(value.toString() );
}
int splitIndex = key.toString().indexOf(":");
//重新设置value值由URI和词频组成
info.set( key.toString().substring( splitIndex + 1) +":"+sum );
//重新设置key值为单词
key.set( key.toString().substring(0,splitIndex));
context.write(key, info);
System.out.println("key"+key);
System.out.println("value"+info);
}
}
/**
*
* @author 汤高
*
*/
public static class InvertedIndexReducer extends Reducer<Text, Text, Text, Text>{
private Text result = new Text();
@Override
protected void reduce(Text key, Iterable<Text> values, Reducer<Text, Text, Text, Text>.Context context)
throws IOException, InterruptedException {
//生成文档列表
String fileList = new String();
for (Text value : values) {
fileList += value.toString()+";";
}
result.set(fileList);
context.write(key, result);
}
}
public static void main(String[] args) {
try {
Configuration conf = new Configuration();
Job job = Job.getInstance(conf,"InvertedIndex");
job.setJarByClass(InvertedIndex.class);
//实现map函数,根据输入的<key,value>对生成中间结果。
job.setMapperClass(InvertedIndexMapper.class);
job.setMapOutputKeyClass(Text.class);
job.setMapOutputValueClass(Text.class);
job.setCombinerClass(InvertedIndexCombiner.class);
job.setReducerClass(InvertedIndexReducer.class);
job.setOutputKeyClass(Text.class);
job.setOutputValueClass(Text.class);
//我把那两个文件上传到这个index目录下了
FileInputFormat.addInputPath(job, new Path("hdfs://192.168.52.140:9000/index/"));
//把结果输出到out_index+时间戳的目录下
FileOutputFormat.setOutputPath(job, new Path("hdfs://192.168.52.140:9000/out_index"+System.currentTimeMillis()+"/"));
System.exit(job.waitForCompletion(true) ? 0 : 1);
} catch (IllegalStateException e) {
e.printStackTrace();
} catch (IllegalArgumentException e) {
e.printStackTrace();
} catch (ClassNotFoundException e) {
e.printStackTrace();
} catch (IOException e) {
e.printStackTrace();
} catch (InterruptedException e) {
e.printStackTrace();
}
}
}
转载自: <https://blog.csdn.net/tanggao1314/article/details/51340672>
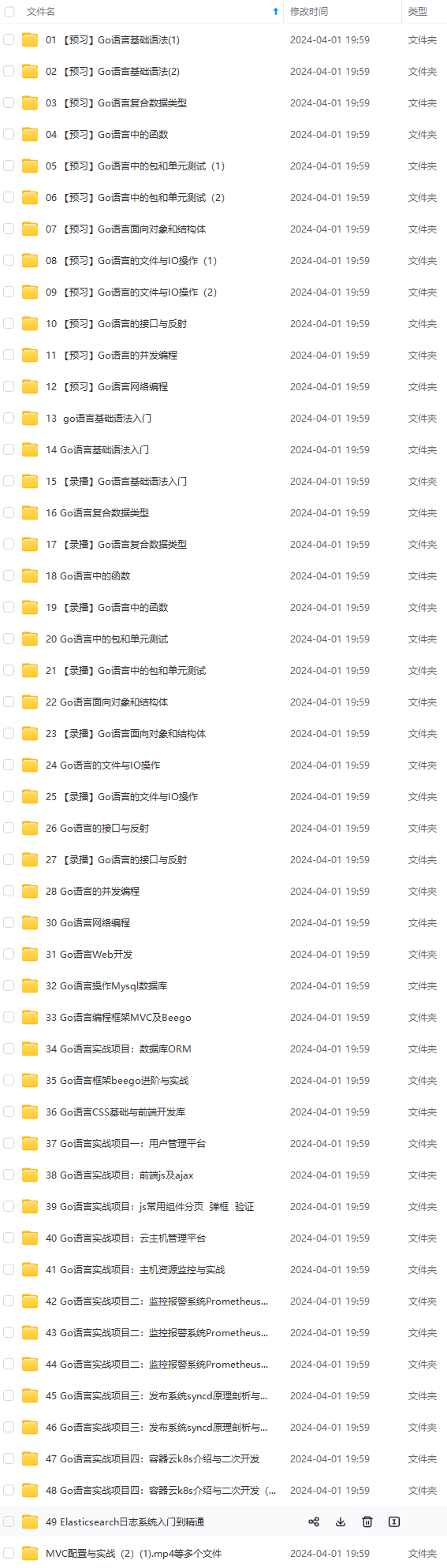
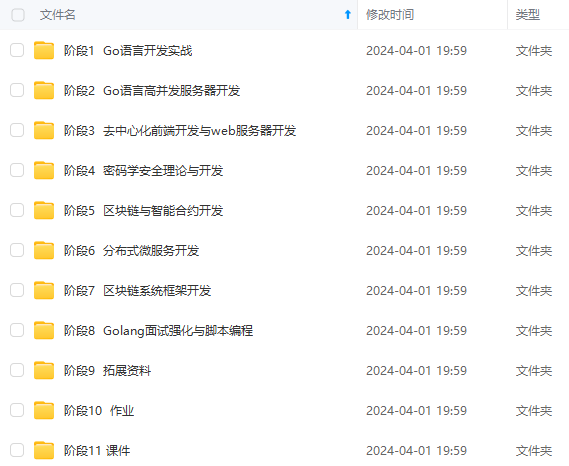
**网上学习资料一大堆,但如果学到的知识不成体系,遇到问题时只是浅尝辄止,不再深入研究,那么很难做到真正的技术提升。**
**[需要这份系统化的资料的朋友,可以添加戳这里获取](https://bbs.csdn.net/topics/618658159)**
**一个人可以走的很快,但一群人才能走的更远!不论你是正从事IT行业的老鸟或是对IT行业感兴趣的新人,都欢迎加入我们的的圈子(技术交流、学习资源、职场吐槽、大厂内推、面试辅导),让我们一起学习成长!**
}
}
}
转载自: https://blog.csdn.net/tanggao1314/article/details/51340672
[外链图片转存中…(img-oIQA0gKb-1715903106846)]
[外链图片转存中…(img-Y7j5UdeR-1715903106846)]
网上学习资料一大堆,但如果学到的知识不成体系,遇到问题时只是浅尝辄止,不再深入研究,那么很难做到真正的技术提升。
一个人可以走的很快,但一群人才能走的更远!不论你是正从事IT行业的老鸟或是对IT行业感兴趣的新人,都欢迎加入我们的的圈子(技术交流、学习资源、职场吐槽、大厂内推、面试辅导),让我们一起学习成长!