1. 数据类型
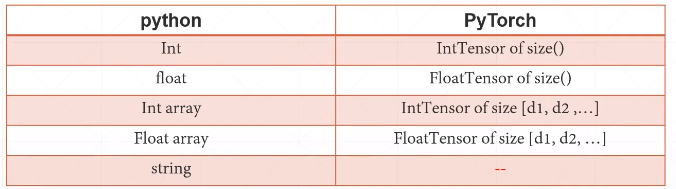
如何表示string?
-
One-hot
- [0, 1, 0, 0, ...]
-
Embedding
-
Word2vec
-
glove
-
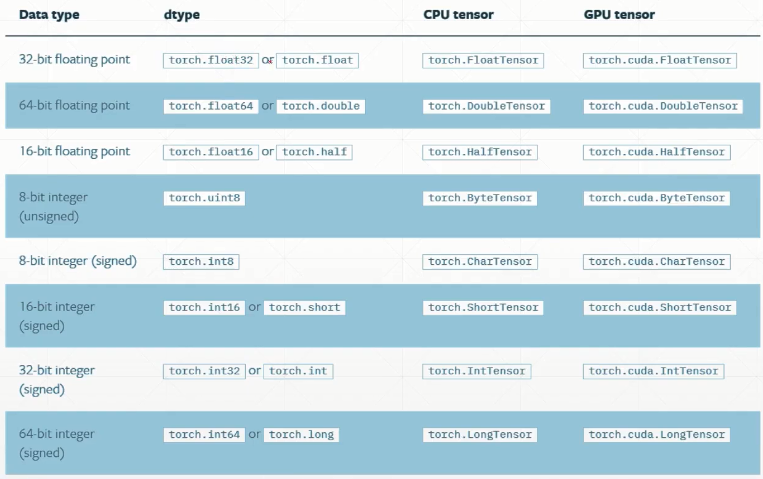
类型推断
import torch
# type check
a = torch.randn(2, 3)
print(a.type()) #torch.FloatTensor
print(type(a)) #<class 'torch.Tensor'> 这种情况比较少
print(isinstance(a, torch.FloatTensor)) #True
标量
- 标量 dimension 0/rank 0(常用于loss)
# 标量 dimension 0/rank 0(常用于loss)
b = torch.tensor(1.3)
print(b) # tensor(1.3000)
print(b.shape) # torch.Size([]) 成员
print(len(b.shape)) # 0
print(b.size()) # torch.Size([]) 成员函数
张量(tensor)
- 张量 dimension 1(常用于bias)
# 张量 dimension 1(常用于bias)
print(torch.tensor([1.1])) # tensor([1.1000]) 指定具体数据,可以是 N 维
print(torch.FloatTensor(1)) # tensor([9.6429e-39]) 指定第一维度的长度,随机初始化
data = np.ones(2) # 长度为2的numpy array
print(data) # array([1., 1.])
print(torch.from_numpy(data)) # tensor([1., 1.], dtype=torch.float64) 从numpy引入
c = torch.ones(2)
print(c.shape) # torch.Size([2]), 第一维的长度为2
- 张量 dimension 2(常用于batch等)
# 张量 dimension 2
d = torch.randn(2, 3)
print(d)
#tensor([[-1.8543, -0.7280, 0.6671],
# [ 1.1492, -0.6379, -0.4835]])
print(d.shape) # torch.Size([2, 3]),第一维长度为2,第二维长度为3(两行3列)
print(d.shape[0]) # 2
print(d.size(1)) # 或者d.shape[1],3
- 张量 dimension 3(常用于RNN等)
# 张量 dimension 3
f = torch.rand(1,2,3) # 理解:1个数据集,2个Tx,每个Tx有3个输出
print(f)
#tensor([[[0.3690, 0.5702, 0.2382],
# [0.3130, 0.5591, 0.3829]]])
print(f.shape) # torch.Size([1, 2, 3])
print(f[0]) # 取第一个维度
#tensor([[0.4535, 0.4307, 0.6469],
# [0.1591, 0.0778, 0.4489]])
print(f[0][1]) # tensor([0.1591, 0.0778, 0.4489])
- 张量 dimension 4(常用于表示图片类型)
eg:a = torch.rand(b,c,h,w) 表示b张 c通道、h*w的图片
a = torch.rand(2,3,28,28) # 2张图片,每张图片3通道,大小为28x28
print(a)
print(a.shape)
print(a.numel()) # 2x3x28x28=4704, 全部元素个数
print(a.dim()) # 4, 维度数量
tensor([[[[0.2607, 0.6929, 0.4447, ..., 0.7346, 0.1117, 0.6536],
...,
[0.4591, 0.7439, 0.0944, ..., 0.0986, 0.9818, 0.9580]],
[[0.2049, 0.2220, 0.6390, ..., 0.7402, 0.0301, 0.1057],
...,
[0.437