模型概述
U-Net 模型是2015年为解决图像语义分割问题而被提出来的,模型作者认为它能够更高效的利用标注样本,因此可以在样本量不大的情况下,得到更好的结果。
U-Net原论文摘要翻译:人们普遍认为,深度网络的成功训练需要成千上万个带注释的训练样本。在本文中,我们提出了一种网络和训练策略,该策略依赖于大量使用数据增强来更有效地利用可用的标注样本。该架构由捕获上下文的收缩路径(contracting path)和对称的支持精确本地化的扩展路径(expanding path)组成。我们表明,这样的网络可以用非常少的图像端到端训练,并且在ISBI竞赛(电子显微镜堆栈神经元结构分割任务)中性能优于先前最好的方法(滑动窗口卷积网络)。在透射式光学显微镜图像(相位对比度和DIC)上训练的相同网络,让我们以较大优势赢得了ISBI cell tracking challenge 2015。此外,模型速度很快。在最新的GPU上,分割512x512图像只需不到一秒的时间。模型实现(基于Caffe)和训练好的网络模型均可在http://lmb.informatik.uni-freiburg.de/people/ronneber/u-net 上找到
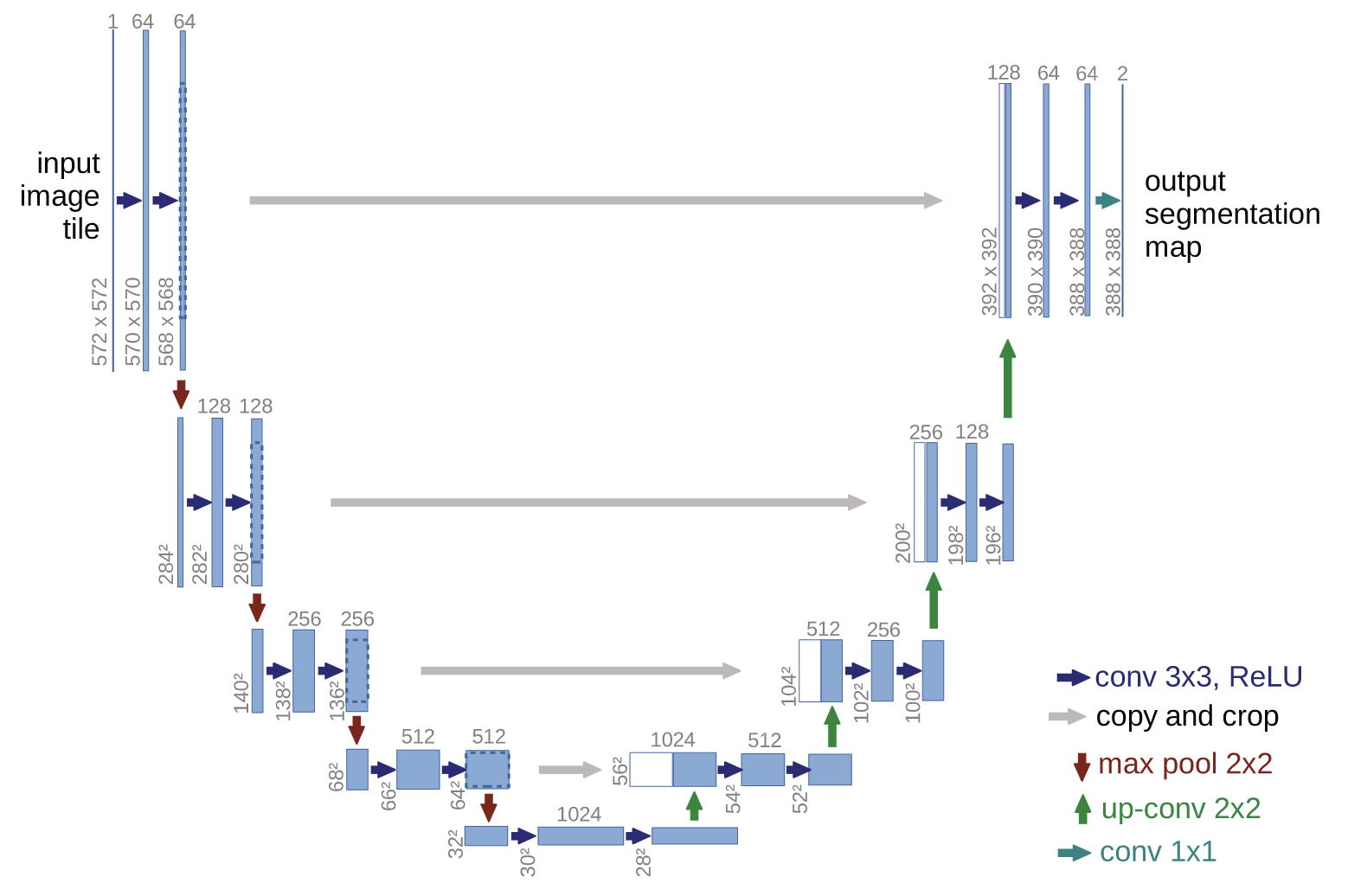
图1 U-Net框架
模型本身看起来比较简单,图1中左边是论文中说的收缩路径(contracting path), 每一个组之间的卷积层的通道数相同,随着网络的变深,卷积层和池化层使图像的大小变小,但是通道数成倍增加(由64-128-256-512变化)。 图1右边是论文中说的扩展路径(expanding path),扩展路径的卷积层的通道数逐步减少(512-256-128-64),并且与之前的网络之间有skip connection(因为图像的大小不一致,所以有crop的操作)。
虽然论文作者没有明确指出,其实U-Net 模型也可以看做encode-decode结构,左边的是encode, 右边的是decode。
相关模型
Unet 之后有很多相关的模型,下面列了一些,以后有需要再看
UNet Ensembled (UNetE) : UNet++: Redesigning Skip Connections to Exploit Multiscale Features in Image Segmentation
UNet+ (UNetP) :UNet++: Redesigning Skip Connections to Exploit Multiscale Features in Image Segmentation
UNet++ (UNetPP): UNet++: A Nested U-Net Architecture for Medical Image Segmentation
UNet3+ :UNet 3+: A full-scale connected unet for medical image segmentation
MultiResUNet :Multiresunet : Rethinking the u-Net Architecture for Multimodal Biomedical Image Segmentation
BCDUNet :Bi-directional CONVLSTM U-Net with densley connected convolutions
IBAUNet :Iba-U-Net: Attentive BCONVLSTM U-net with redesigned inception for Medical Image segmentation
SEDUNet or MCGUNet :Multi-level context gating of embedded collective knowledge for medical image segmentation
NABNet :NABNet: A nested attention-guided BICONVLSTM network for a robust prediction of blood pressure components from reconstructed arterial blood pressure waveforms using PPG and ECG signals
参考资料
Olaf Ronneberger, Philipp Fischer and Thomas Brox. “U-Net: Convolutional Networks for Biomedical Image Segmentation” Lecture Notes in Computer Science(2015): n. pag.