Softmax与SVM都是用来对数据进行分类的。Softmax常用于神经网络的输出层,SVM常常直接与SGD配合实现物体分类。无论是Softmax还是SVM在工作时都需要计算出loss和gradient,学习使用中发现两者有很多相似之处,特拿来对比学习。
公式
图解
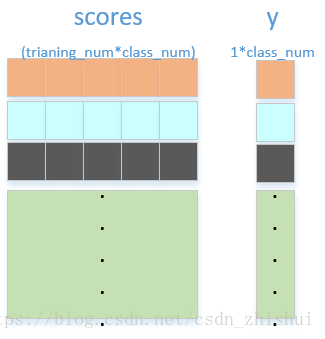
python代码实现
"""
Structured softmax and SVM loss function.
Inputs have dimension D, there are C classes, and we operate on minibatches
of N examples.
Inputs:
- W: A numpy array of shape (D, C) containing weights.
- X: A numpy array of shape (N, D) containing a minibatch of data.
- y: A numpy array of shape (N,) containing training labels; y[i] = c means
that X[i] has label c, where 0 <= c < C.
Returns a tuple of:
- loss as single float
- gradient with respect to weights W; an array of same shape as W
"""
def softmax_loss_vectorized(W, X, y):
loss = 0.0
dW = np.zeros_like(W)
num_train = X.shape[0]
score = X.dot(W)
shift_score = score - np.max(score, axis=1, keepdims=True) # 对数据做了一个平移
shift_score_exp = np.exp(shift_score)
shift_score_exp_sum = np.sum(shift_score_exp, axis=1, keepdims=True)
score_norm = shift_score_exp / shift_score_exp_sum
loss = np.sum(-np.log(score_norm[range(score_norm.shape[0]), y])) / num_train
# dW
d_score = score_norm
d_score[range(d_score.shape[0]), y] -= 1
dW = X.T.dot(score_norm) / num_train
return loss, dW
def svm_loss_vectorized(W, X, y):
delta = 1
num_training = X.shape[0]
scores = X.dot(W)
scores_gt_cls = scores[range(num_training), y][..., np.newaxis]
scores_dis = scores - scores_gt_cls + delta
scores_dis[range(num_training), y] -= delta
scores_norm = np.maximum(0, scores_dis)
loss = np.sum(scores_norm) / num_training
d_scores = scores_norm
d_scores[d_scores > 0] = 1 # 出现错误得分的地方统统设为1
row_sum=np.sum(d_scores, axis=1)
d_scores[range(num_training), y] -= row_sum
dW = X.T.dot(d_scores)/num_training
return loss, dW