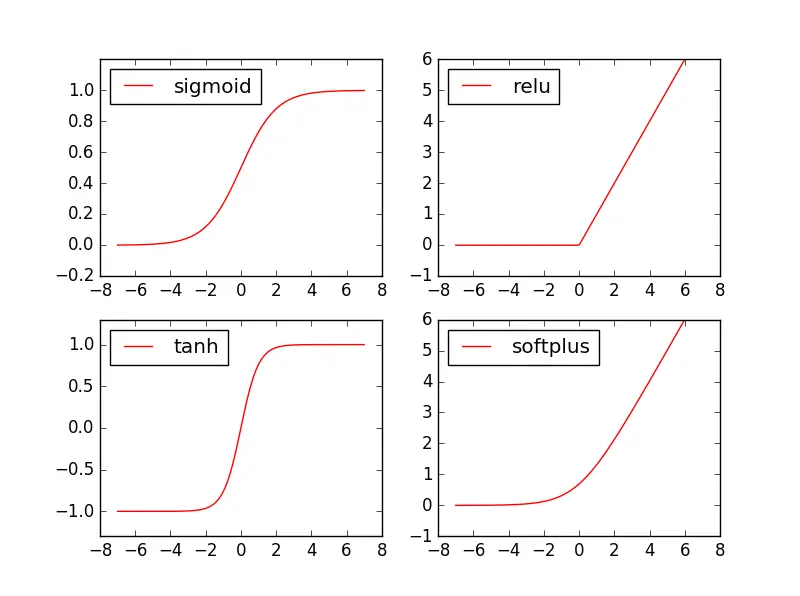
激活函数.png
import numpy as np
import tensorflow as tf
import matplotlib.pyplot as plt
#创建输入数据
x = np.linspace(-7,7,180) #(-7,7,)等间隔的180个点
def sigmoid(inputs):
y= [1/float(1+np.exp(-x)) for x in inputs]
return y
def relu(inputs):
y = [x* (x>0) for x in inputs]
return y
def tanh(inputs):
y = [(np.exp(x) - np.exp(-x)) / float(np.exp(x)+ np.exp(-x)) for x in inputs ]
return y
def softplus(inputs):
y= [np.log(1+np.exp(x)) for x in inputs]
return y
#经过tensorflow的激活函数的各个Y值
y_sigmoid = tf.nn.sigmoid(x)
y_relu = tf.nn.relu(x)
y_tanh = tf.nn.tanh(x)
y_softplus = tf.nn.softplus(x)
#创建会话
sess = tf.Session()
#运行
y_sigmoid, y_relu, y_tanh ,y_softplus = sess.run([y_sigmoid,y_relu,y_tanh,y_softplus])
#创建各个激活函数图像
plt.subplot(221)#二行二列第一个图像
plt.plot(x,y_sigmoid, c= "red",label = "sigmoid")
plt.ylim(-0.2,1.2)
plt.legend(loc = "best")
plt.subplot(222)#二行二列第二个图像
plt.plot(x,y_relu, c= "red",label = "relu")
plt.ylim(-1,6)
plt.legend(loc = "best")
plt.subplot(223)#二行二列第三个图像
plt.plot(x,y_tanh, c= "red",label = "tanh")
plt.ylim(-1.3,1.3)
plt.legend(loc = "best")
plt.subplot(224)#二行二列第四个图像
plt.plot(x,y_softplus, c= "red",label = "softplus")
plt.ylim(-1,6)
plt.legend(loc = "best")
plt.show()
sess.close()