Review of old Collaborative filtering
In the last few classes, we know that:
- If you're given features for movies, you can use that to learn parameters
for users
figure-1 - If you're given parameters for the users, you can use that to learn features for movies
figure-2
One thing you could do is go back and forth. Maybe randomly initialize parameter , then solve for
. Then solve for better
, then better
, and so on.
Optimized Collaborative filtering algorithm objective
I want to point out that the two square error terms in figure-1 and figure-2 are actually the same, that is:

- The left hand is sum over all users
, and then sum over all movies rated by that user.
- The right hand just does things in the opposite order. For every movie
, sum over all the users
that have rated that movie.
So both summations on the left and right hands are just summations over all pairs for which
.
And thus, we have:

So we can define the following cost function by putting above two cost functions in figure-1 and figure-2 together:

It actually has an interesting property:
- If you were to hold the
constant, then you'll be solving exactly the problem of figure-1
- If you were to hold the
constant, then you'll be solving exactly the problem of figure-2
With the new cost function, instead of sequentially going back and forth between the two sets of parameters and
, we'll just minimize with respect to both sets of parameters simultaneously.
Note:
With the new Collaborative filtering algorithm, we have by doing away with the intercept term
which was set to 1 by convention. And similarly,
. The reason is we're now learning all the features automatically. There is no need to hard code the feature that is always equal to 1. If the algorithm really wants a feature that is always equal to 1, it can choose to learn one for itself. The algorithm has the flexibility to just learn it by itself.
Optimized Collaborative filtering algorithm
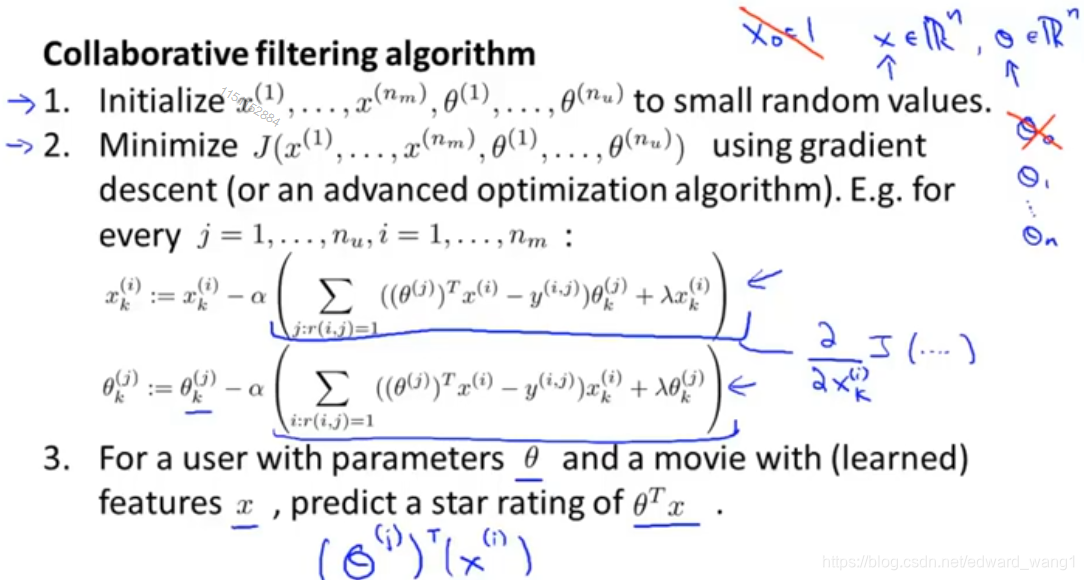
Figure-6 shows the steps of Collaborative filtering algorithm. Firstly, we randomly initialize the feature and parameter
to some small values. Then minimize the features and parameters with GD or other advanced optimization algorithm. Then, suppose user
has not rated movie
yet, then the rating can be calculated as
.
<end>