from numpy import *
import matplotlib.pyplot as plt
1.导入数据
points = genfromtxt('linear_regress_lsm_data.csv', delimiter=',')
length = len(points)
print('point count %d'%length)
x = array(points[:, 0])
y = array(points[:, 1])
plt.scatter(x, y)
plt.show
point count 100
<function matplotlib.pyplot.show(close=None, block=None)>
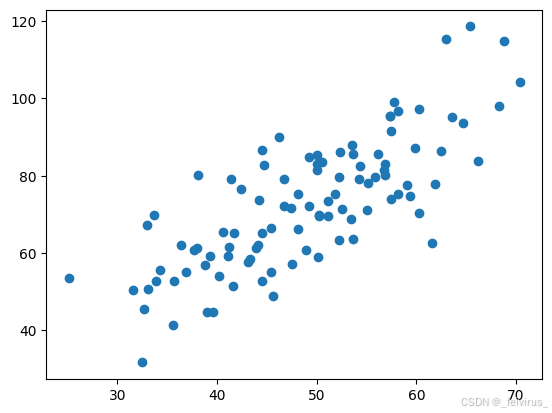
2.损失函数
def compute_cost(points, w, b):
total_cost = 0
length = len(points)
for i in range(length):
x = points[i, 0]
y = points[i, 1]
total_cost = (y - w * x - b) ** 2
return total_cost / float (length)
3.模型超参
learning_rate = 0.0001
init_b = 0
init_w = 0
num_iteration = 10
4.梯度下降
def gradient_descent(points, init_w, init_b, learning_rate, num_iteration):
b = init_b
w = init_w
cost_list = []
for i in range(num_iteration):
cost_list.append(compute_cost(points, w, b))
w, b = step_grad_desc(w, b, array(points), learning_rate)
return [w, b, cost_list]
def step_grad_desc(cur_w, cur_b, points, learning_rate):
w_grad = 0
b_grad = 0
poi_len = len(points)
for i in range(poi_len):
x = points[i, 0]
y = points[i, 1]
w_grad +=(cur_w * x + cur_b - y) * x
b_grad += cur_w * x + cur_b - y
new_w = cur_w - learning_rate * w_grad * (2 / float(poi_len))
new_b = cur_b - learning_rate * b_grad * (2 / float(poi_len))
return new_w, new_b
5.训练
w, b, cost_list = gradient_descent(points, init_w, init_b, learning_rate, num_iteration)
print("w= ", w)
print("b= ", b)
print("cost= ", compute_cost(points, w, b))
w= 1.4774173755483797
b= 0.02963934787473238
cost= 2.656735381402925
6.看损失函数随着迭代下降
plt.plot(cost_list)
plt.show()
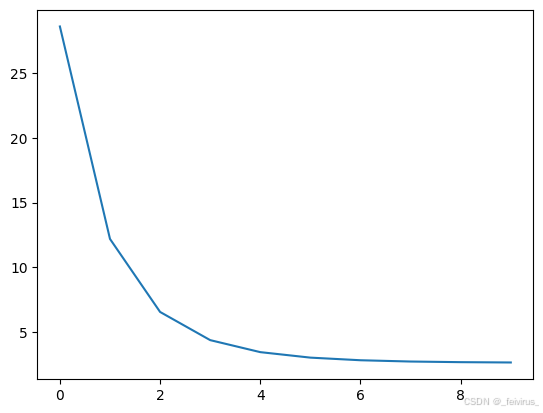
7.画拟合曲线
plt.scatter(x, y)
y_predict = w * x + b
plt.plot(x, y_predict, c = 'y')
plt.show
<function matplotlib.pyplot.show(close=None, block=None)>
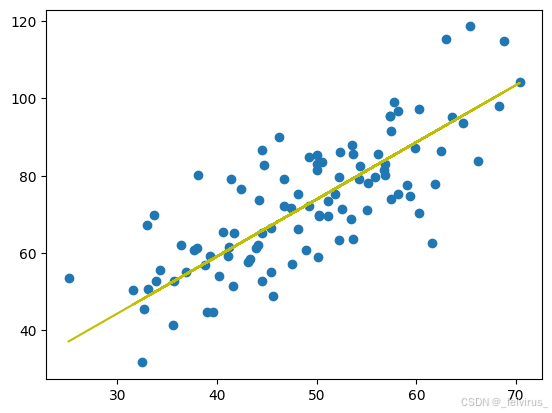