【CVPR2020】Non-local neural networks with grouped bilinear attention transforms
代码:https://github.com/BA-Transform/BAT-Image-Classification
作者提出了一种名为 bilinear attention 的 non-local 的建模方法,目标是把输入特征 X ∈ R c × h × w X\in\mathbb{R}^{c\times h \times w} X∈Rc×h×w 变成 Y ∈ R c × h × w Y\in\mathbb{R}^{c\times h \times w} Y∈Rc×h×w ,是通过矩阵相乘来实现的(如下图所示,即bilinear)。关键难题是,如何构建 P ∈ R h × h P\in\mathbb{R}^{h\times h} P∈Rh×h 矩阵和 Q ∈ R w × w Q \in \mathbb{R}^{w\times w} Q∈Rw×w 矩阵 ?
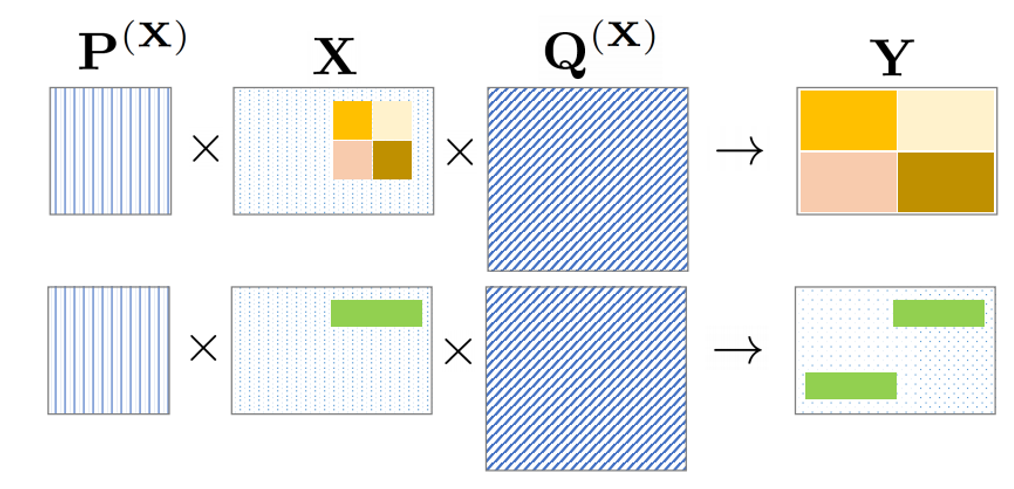
如下图所示, P P P 和 Q Q Q 矩阵的生成,是通过一维卷积来实现的,实际操作中,作者对 P , Q , X P,Q,X P,Q,X 矩阵都进行了降维处理,进一步减少了计算量。
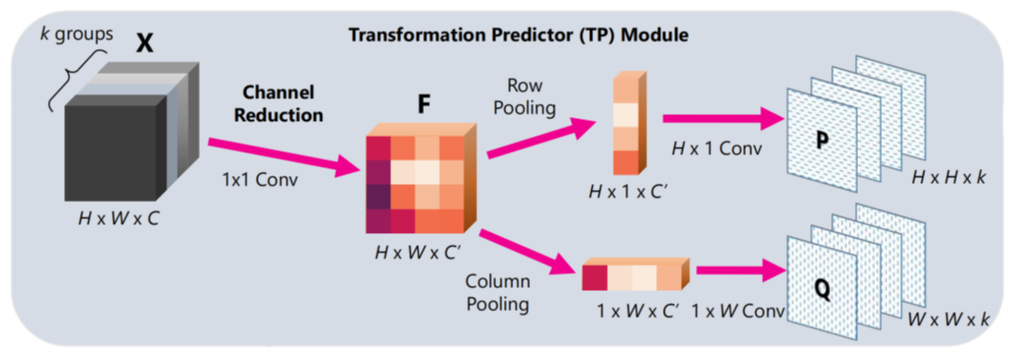
模块的整体架构如下图所示,可以看出是经过BA变换,得到 P P P矩阵和 Q Q Q矩阵,然后再和输入的 X X X相乘,就可以得到 Y Y Y矩阵。
论文有趣的地方是 Motivation 的阐述,作者说,研究动机来自于人类视觉系统,视觉系统有bottom-up的机制(Marr的视觉理论,由边缘抽象成整体概念),了有 top-down 机制(Gestalt的视觉理论,由整体快速关注到感兴趣区域),论文方法与两个机制的对应,作者这样描述的:
- Our proposed BA-Transform supports a large variety of operations on the attended image or video parts albeit its simplicity, including numerous affine transformation (selective scaling, shift, rotation, cropping etc.), suppressing / strengthening local structure or even global reasoning. 这就对应了human are remarkably capable of capturing attention patterns(Bottom-up机制)
- Bilinear matrix multiplication is amenable to efficient differential calculation. Top-down supervision can be gradually back-propagated to shallow layers and enforce the consistency between learned attentions and top-down supervision.
这就对应了 top-down 机制