在使用tensorflow搭建模型时会有特征工程的工作,今天介绍一下tensorflow做特征工程的api:tf.feature_column。
feature_column 输入输出类型
1.深度模型的输入必须是Dense类型,所有输出是categorical类型需要经过indicator或者embedding的转换才可以
2.indicator, embedding, bucketized的输入不能是原始特征,前两者只能是categorical类型的feature_column, 后者只能是numeric_column
feature_column | 输入 | 输出 | 输出是否为dense |
---|---|---|---|
categorical_column_with_identity | 数值型离散 | categorical | N |
categorical_column_with_vocabulary_list | 字符型/数值型离散 | categorical | N |
categorical_column_with_hash_bucket | 类别太多的离散值 | categorical | N |
crossed_column | categorical/离散值 | categorical | N |
indicator_column | categorical | one/multi-hot | Y |
embedding_column | categorical | dense vector | Y |
numeric_column | 数值型连续值 | numeric | Y |
bucketzied_column | numeric_column | one-hot | Y |
首先介绍一下tf.feature_column.input_layer,使用 input_layer 作为model的一个输入。
tf.feature_column.input_layer(
features,
feature_columns,
weight_collections=None,
trainable=True,
cols_to_vars=None,
cols_to_output_tensors=None
)
参数:
features:字典,最主要的是dict的key一定要与 feature_columns的key一致,后续才能 才能根据key进行匹配。
feature_columns:该参数必须是继承于DenseColumn的numeric_column, embedding_column, bucketized_column, indicator_column,
如果feature是类别的,那么必须先 用embedding_column or indicator_column封装一下使用。
输入连续值
1、tf.feature_column.numeric_column
numeric_column(
key,
shape=(1,),
default_value=None,
dtype=tf.float32,
normalizer_fn=None
)
参数:
key: 特征的名字。也就是对应的列名称。
shape: 该key所对应的特征的shape. 默认是1,但是比如one-hot类型的,shape就不是1,而是实际的维度。总之,这里是key所对应的维度,不一定是1.
default_value: 如果不存在使用的默认值
normalizer_fn: 对该特征下的所有数据进行转换。如果需要进行normalize,那么就是使用normalize的函数.这里不仅仅局限于normalize,也可以是任何的转换方法,比如取对数,取指数,这仅仅是一种变换方法.
def norm(x):
return x**2
features = {'age': [[50], [18], [13],[29],[43]]}
age = tf.feature_column.numeric_column('age',normalizer_fn= norm)
sess.run(tf.feature_column.input_layer(features,[age]))
array([[2500.],
[ 324.],
[ 169.],
[ 841.],
[1849.]], dtype=float32)
不适用转换函数时:
age = tf.feature_column.numeric_column('age')
sess.run(tf.feature_column.input_layer(features,[age]))
array([[50.],
[18.],
[13.],
[29.],
[43.]], dtype=float32)
2、tf.feature_column.bucketized_column
bucketized_column(
source_column,
boundaries
)
参数:
source_column: 必须是numeric_column
boundaries: 不同的桶。boundaries=[0., 1., 2.],产生的bucket就是, (-inf, 0.), [0., 1.), [1., 2.), and [2., +inf), 每一个区间分别表示0, 1, 2, 3,所以相当于分桶分了4个.
features = {'age': [[50], [18], [13],[29],[43]]}
age_bin = tf.feature_column.bucketized_column(age,boundaries = [10.,15.,25.,35.,45.,55.])
sess.run(tf.feature_column.input_layer(features,[age_bin]))
array([[0., 0., 0., 0., 0., 1., 0.],
[0., 0., 1., 0., 0., 0., 0.],
[0., 1., 0., 0., 0., 0., 0.],
[0., 0., 0., 1., 0., 0., 0.],
[0., 0., 0., 0., 1., 0., 0.]], dtype=float32)
输入离散值
3、tf.feature_column.categorical_column_with_vocabulary_list
categorical_column_with_vocabulary_list(
key,
vocabulary_list,
dtype=None,
default_value=-1,
num_oov_buckets=0
)
作用:将类别特征进行hash映射。根据单词的序列顺序,把单词根据index转换成one hot encoding。
参数:
key: feature名字
vocabulary_list: 对于category来说,进行转换的list.也就是category列表.
dtype: 仅仅string和int被支持,其他的类型是无法进行这个操作的.
default_value: 当不在vocabulary_list中的默认值,这时候num_oov_buckets必须是0.
num_oov_buckets: 用来处理那些不在vocabulary_list中的值,如果是0,那么使用default_value进行填充;如果大于0,则会在[len(vocabulary_list), len(vocabulary_list)+num_oov_buckets]这个区间上重新计算当前特征的值.与前面numeric 不同的是,这里返回的是稀疏tensor.
cat_job = tf.feature_column.categorical_column_with_vocabulary_list('gender',['male','female'])
cat_job
_VocabularyListCategoricalColumn(key='gender', vocabulary_list=('male', 'female'), dtype=tf.string, default_value=-1, num_oov_buckets=0)
类别较多是使用tf.feature_column.categorical_column_with_hash_bucket
4、tf.feature_column.categorical_column_with_identity
作用:把numerical data转成one hot encoding,只适用于值为整数的类别型变量
参数:
key:features是一个字典,key是特征名字,value是特征值。features[key]或者是Tensor或SparseTensor 。如果Tensor ,
缺失值,可以表示为-1为int和''字符串,这将通过此功能列被删除。dict的key一定要与 feature_columns的key一致,后续才能根据key进行匹配。
num_buckets: 分桶的个数。
default_value:当你输入的范围内的整数使用此[0, num_buckets)并且要使用的输入值本身作为分类ID。
超出此范围的值将导致default_value如果指定,否则就会失败。下面的例子中,在输入的文字0将导致相同的默认ID。
feature_columns:必须是继承于DenseColumn的numeric_column, embedding_column, bucketized_column, indicator_column。如果feature是类别的,那么必须先用embedding_column或者indicator_column封装一下使用。
features = {'birthplace': [[1],[1],[3],[4]]}
#特征列
birthplace = tf.feature_column.categorical_column_with_identity("birthplace", num_buckets=5, default_value=0)
birthplace = tf.feature_column.indicator_column(birthplace)
#组合特征列
columns = [birthplace]
#输入层(数据,特征列)
inputs = tf.feature_column.input_layer(features, columns)
v=sess.run(inputs)
print(v)
[[0. 1. 0. 0. 0.]
[0. 1. 0. 0. 0.]
[0. 0. 0. 1. 0.]
[0. 0. 0. 0. 1.]]
5、tf.feature_column.indicator_column(categorical_column)
作用:将 categorical_column表示成 multi-hot形式的 dense tensor,同一个元素在一行出现多次, 计数会超过1。
参数:
必须是categorical_column,只有 categorical_column_with_* ,crossed_column 以及bucketized_column 类型的column 才可以使用该函数。
import tensorflow as tf
sess=tf.Session()
#特征数据
features = {'sex': [['male','other'], ['male','female'], ['female','female'],['other','female']]}
#特征列
sex_column = tf.feature_column.categorical_column_with_vocabulary_list('sex', ['male', 'female','other'],num_oov_buckets=2)
sex_column = tf.feature_column.indicator_column(sex_column)
#组合特征列
columns = [sex_column]
#输入层(数据,特征列)
inputs = tf.feature_column.input_layer(features, columns)
#初始化并运行
init = tf.global_variables_initializer()
sess.run(tf.tables_initializer())
sess.run(init)
v=sess.run(inputs)
print(v)
[[1. 0. 1. 0. 0.]
[1. 1. 0. 0. 0.]
[0. 2. 0. 0. 0.]
[0. 1. 1. 0. 0.]]
如果你已经来到这里,不妨关注如下公众号一起探索这个奇妙世界:
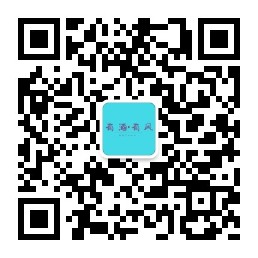
6、tf.feature_column.embedding_column
tf.feature_column.embedding_column(
categorical_column,
dimension,
combiner='mean',
initializer=None,
ckpt_to_load_from=None,
tensor_name_in_ckpt=None,
max_norm=None,
trainable=True
)
该方法和indicator_column一样,只接受 categorical_column,目的是将 稀疏矩阵转换为稠密矩阵。
参数:
categorical_column:入参categorical_column_with_*的返回
dimension: embedding 的维度,一般计算规则是 类别开4次方,但是也可以根据需要自行设置
combiner: 多个 vector的 组合方式,有 mean(default),sqrtn以及sum
initializer:embedding matrix的初始化值,默认 均值0,标准差 1/sqrt(dimension)的tf.truncated_normal_initializer
ckpt_to_load_from以及tensor_name_in_ckpt 主要是为了使用pre-trained embedding matrix
max_norm: if not 'None',则使用l2 归一化
trainable: 是否可训练的
features = {'aa': [[2], [1], [3]]}
# 特征列 feature_column
aa_fc = tf.feature_column.categorical_column_with_identity('aa', num_buckets=9, default_value=0)
# aa_fc = tf.feature_column.indicator_column(aa_fc)
'''对于维度特别大的feature_column, 使用 embedding_column, 过于稀疏的特征对模型影响比较大 '''
aa_fc = tf.feature_column.embedding_column(aa_fc, dimension=4)
# 组合特征列 feature_columns
columns = [aa_fc]
# 输入层
inputs = tf.feature_column.input_layer(features=features, feature_columns=columns)
# 初始化并运行
sess = tf.Session()
variables_init = tf.global_variables_initializer()
table_init = tf.tables_initializer()
sess.run(variables_init)
sess.run(table_init)
v = sess.run(inputs)
print(v)
[[-0.08986011 -0.58195084 -0.28713277 -0.10367975]
[ 0.10307329 -0.41133747 -0.2876911 -0.13596815]
[-0.8687555 0.4577404 0.160222 -0.23040968]]
7、tf.feature_column.crossed_column
特征交叉,交叉后的Tensor。输出为cross的one-hot结果,hash_bucket_size代表输出的交叉向量的one-hot维度,按照index进行交叉的,也就是说同一个样本,不同特征进行交叉。
对于缺失的特征,使用前一个index的特征做交叉。
#特征数据
features = {
'sex': [1, 2, 1, 1, 2],
'department': ['sport', 'sport', 'drawing', 'gardening', 'travelling'],
}
#特征列
department = tf.feature_column.categorical_column_with_vocabulary_list('department', ['sport','drawing','gardening','travelling'], dtype=tf.string)
sex = tf.feature_column.categorical_column_with_identity('sex', num_buckets=2, default_value=0)
sex_department = tf.feature_column.crossed_column([department,sex], 10)
# sex_department = tf.feature_column.crossed_column([features['department'],features['sex']], 16)
sex_department = tf.feature_column.indicator_column(sex_department)
#组合特征列
columns = [sex_department]
#输入层(数据,特征列)
inputs = tf.feature_column.input_layer(features, columns)
#初始化并运行
init = tf.global_variables_initializer()
sess.run(tf.tables_initializer())
sess.run(init)
v=sess.run(inputs)
print(v)