Abstract
field:vision-language intelligence
the development in this field :
task-specifific methods
vision-language pre-training (VLP) methods
larger models
empowered by large-scale weakly-labeled data.
paper logic:
take some common VL tasks as examples to introduce the development
of task-specifific methods
focus on VLP methods and comprehensively review key components of the model structures
and training methods
show how recent work utilizes large-scale raw image-text data to learn language-aligned
visual representations that generalize better on zero or few shot learning tasks
discuss some potential future trends
towards modality cooperation, unifified representation, and knowl
edge incorporation
I. I
NTRODUCTION
three eras:
specialized models are designed for different task from 2014 to
2018
joint representations of vision and language are learned by pre-training on well-labeled
VL datasets from 2019 to 2021
seek to pre-train VL models on larger weakly-labeled datasets and to obtain a strong zero/few-shot vision model with VL pre-training from 2021 till now
the general goal:
to learn good visual representations
A good visual representation should have three attributes:
object-level:
granularity of vision and language features should be as fine
as in object and word-level, respectively.
language-aligned:
the vision feature aligned with language can
help in vision tasks.
semantic-rich:
the representation
should be learned from large-scale data without domain
restriction.
II. TASK SPECIFIC PROBLEMS
the development of task-specifific methods is from global representations to fine-grained object-centric representations
Most VL tasks experience three stages:
gloabl vector representation and simple fusion
grid feature representation and cross-modal attention
object-centric feature representation and bottom-up top-down attention
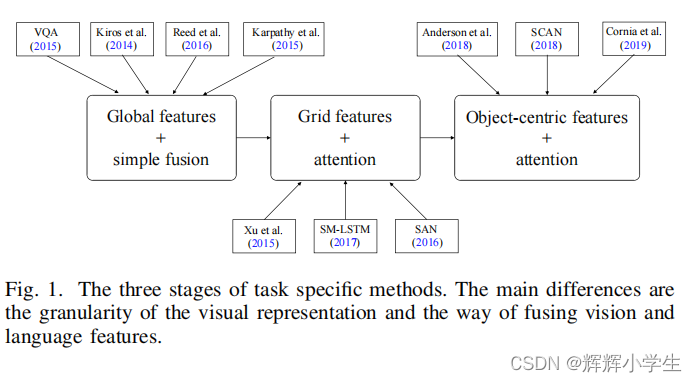
A. Image Captioning(
to generate
a caption for a given image
)
Visual representation develops from image-level global features to fifine-grained and object-level
region features.
language decoding develops from LSTM to attention-based models.
B. VQA (
Given an image-question pair, VQA re
quires answering a question based on the image.
)
to fuse image and language features, attention is the most widely used one.
C. Image Text Matching (
Given
a query in a certain modality (vision or language), it aims
to find the semantically closest target from another modality.
)
two sub-tasks:
image-to-text retrieval and text-to-image retrieval
to calculate the similarity between image and text
D. Other tasks
Text-to-Image Generation:
Given a piece of text, generate
an image containing the content of the text.
Visual Dialog:
Given an image, a dialog history, and a
question about the image, answer the question.
Visual Reasoning:
requires answer
ing a question about an input image
Visual Entailment:
Given an image and a text, decide
whether the image semantically entails the input text.
Phrase Grounding and Reference Expression Comprehension:
require a model to output bounding
boxes corresponding to the text. For phrase grounding, the text
is a set of phrases and for reference expression comprehension,
the text is an expression.
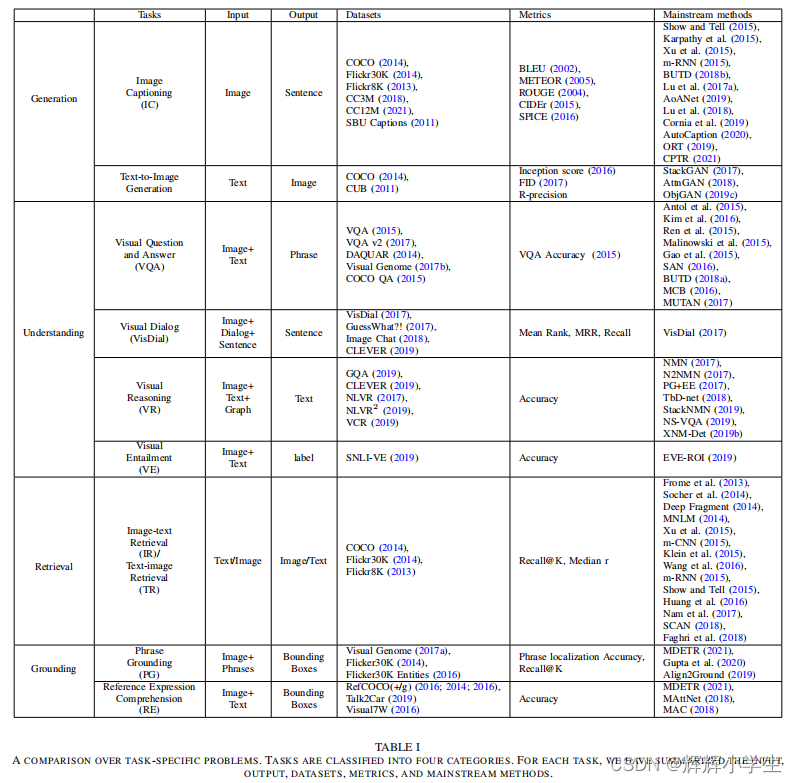
III. VISION LANGUAGE JOINT REPRESENTATION
three components in VLP models:VE,TE,MF
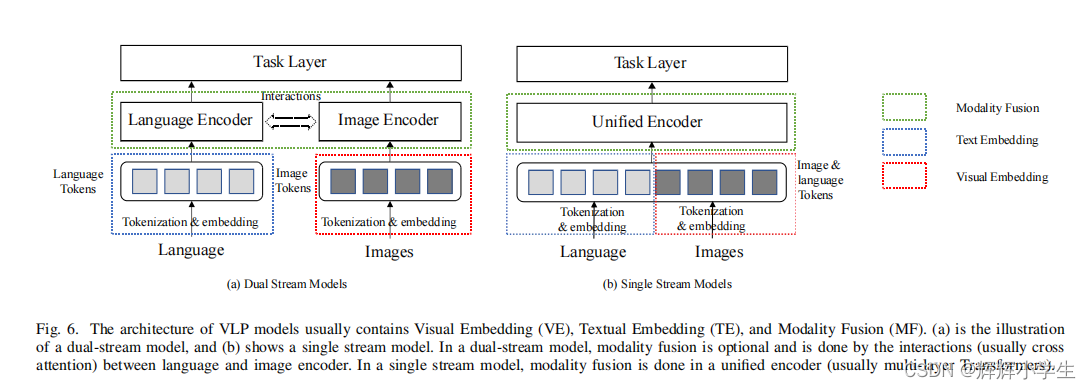
A. Why Pre-training Is Needed? (原文写的特别好,这里略)
B. Modality Embedding
1) Text Tokenization and Embedding:
each word as a token -> a subword tokenization approach
2) Visual Tokenization and Embedding:
1) Grid features:
directly extracted from equally sized
image grids with a convolution feature extractor
advantages:
convenient as it does not require a pre-trained object detector
besides salient objects, grid features also contain background which may be useful for downstream tasks
disadvanteges:not object-level(俺自己加的 嘻嘻)
2) Region features:
extracted by a pre-trained object
detector
three essential components of region features:
bounding boxes, object tags, and RoI features (feature vectors
after RoI pooling)
advantages:
focus on meaningful
regions of the image which might be pretty closely related as well as helpful to downstream tasks.
3) Patch features:
extracted by a linear projection
on evenly divided image patches
The main difference between patch and grid features is that grid features are extracted from
the feature map of a convolutional model while patch features
directly utilize a linear projection.
advanteges:efficiency
C. Modality Fusion
1) Dual stream modeling: 2个encoder
2) Single stream modeling: 单encoder
D. Training
后面略了
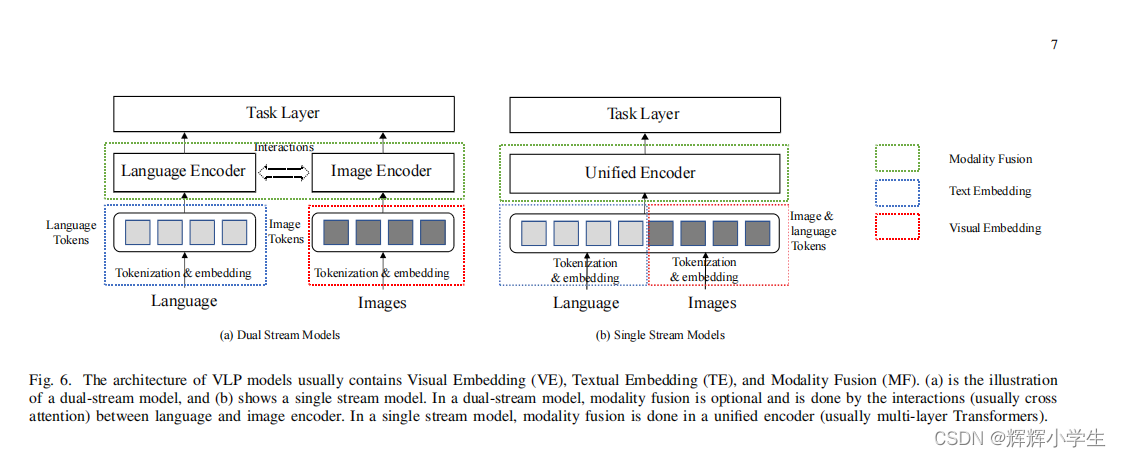