def objective(space):
model = xgb.XGBRegressor(
max_depth = int(space['max_depth']),
n_estimators = int(space['n_estimators']),
subsample = space['subsample'],
colsample_bytree = space['colsample_bytree'],
learning_rate = space['learning_rate'],
reg_alpha = space['reg_alpha']
)
X_train, X_test, y_train, y_test = train_test_split(train, 'count')
eval_set = [( X_train, y_train), ( X_test, y_test)]
(_, registered_pred) = fit_and_predict(train, model, 'registered_log')
(_, casual_pred) = fit_and_predict(train, model, 'casual_log')
y_test = train[train.is_test == True]['count']
y_pred = (np.exp2(registered_pred) - 1) + (np.exp2(casual_pred) -1)
score = rmsle(y_test, y_pred)
print ("SCORE:
使用 Hyperopt 进行参数调优
最新推荐文章于 2023-09-14 09:35:31 发布
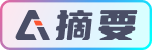