1、NN
1.1
基础网络的解析
其中,测试和验证集能赋值到 tf.constant() 中,而训练集可以导入 tf.placeholder() 中,训练集只有导入占位符我们才能在随机梯度下降中成批量地进行训练。网络定义的权重矩阵和偏置向量后需要执行初始化,每一层需要一个权重矩阵和一个偏置向量。构建损失函数,并计算训练损失。模型会输出一个预测向量,我们可以比较预测标签和真实标签并使用交叉熵函数和 softmax 回归来确定损失值。训练损失衡量预测值和真实值之间差距,并用于更新权重矩阵。优化器,优化器将使用计算的损失值和反向传播算法更新权重和偏置项参数。
1.2 数据的加载过程
这里有一些定义的函数用来加载数据时候使用:
-
def randomize(dataset, labels):
-
permutation = np.random.permutation(labels.shape[ 0])
-
shuffled_dataset = dataset[permutation, :, :]
-
shuffled_labels = labels[permutation]
-
return shuffled_dataset, shuffled_labels
-
def one_hot_encode(np_array):
-
return (np.arange( 10) == np_array[:, None]).astype(np.float32)
def reformat_data(dataset, labels, image_width, image_height, image_depth):
-
np_dataset_ = np.array([np.array(image_data).reshape(image_width, image_height, image_depth) for image_data in dataset])
-
np_labels_ = one_hot_encode(np.array(labels, dtype=np.float32))
-
np_dataset, np_labels = randomize(np_dataset_, np_labels_)
-
return np_dataset, np_labels
def flatten_tf_array(array):
-
shape = array.get_shape().as_list()
-
return tf.reshape(array, [shape[ 0], shape[ 1] * shape[ 2] * shape[ 3]])
-
def accuracy(predictions, labels):
-
return ( 100.0 * np.sum(np.argmax(predictions, 1) == np.argmax(labels, 1)) / predictions.shape[ 0]) def randomize(dataset, labels):
-
permutation = np.random.permutation(labels.shape[ 0])
-
shuffled_dataset = dataset[permutation, :, :]
-
shuffled_labels = labels[permutation]
-
return shuffled_dataset, shuffled_labels
-
-
def one_hot_encode(np_array):
-
return (np.arange( 10) == np_array[:, None]).astype(np.float32)
-
def reformat_data(dataset, labels, image_width, image_height, image_depth):
-
np_dataset_ = np.array([np.array(image_data).reshape(image_width, image_height, image_depth) for image_data in dataset])
-
np_labels_ = one_hot_encode(np.array(labels, dtype=np.float32))
-
np_dataset, np_labels = randomize(np_dataset_, np_labels_)
-
return np_dataset, np_labels
-
-
def flatten_tf_array(array):
-
shape = array.get_shape().as_list()
-
return tf.reshape(array, [shape[ 0], shape[ 1] * shape[ 2] * shape[ 3]])
-
-
def accuracy(predictions, labels):
-
return ( 100.0 * np.sum(np.argmax(predictions, 1) == np.argmax(labels, 1)) / predictions.shape[ 0])
实例:
mnist手写数据集的加载方法
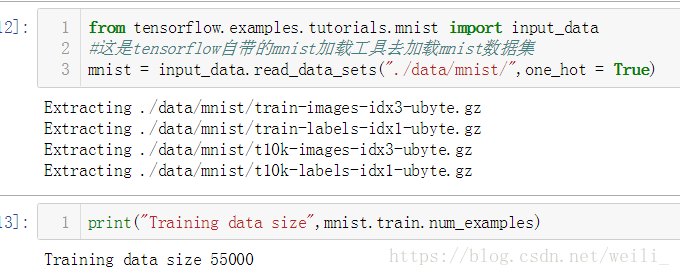
2.cifar10数据集的下载:
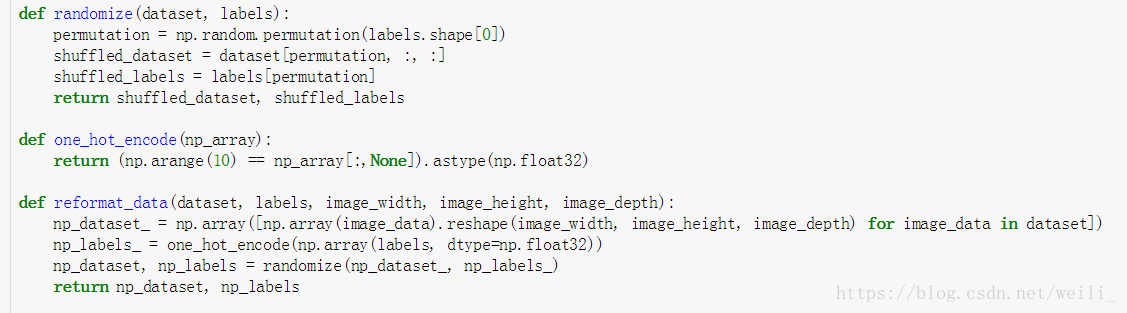
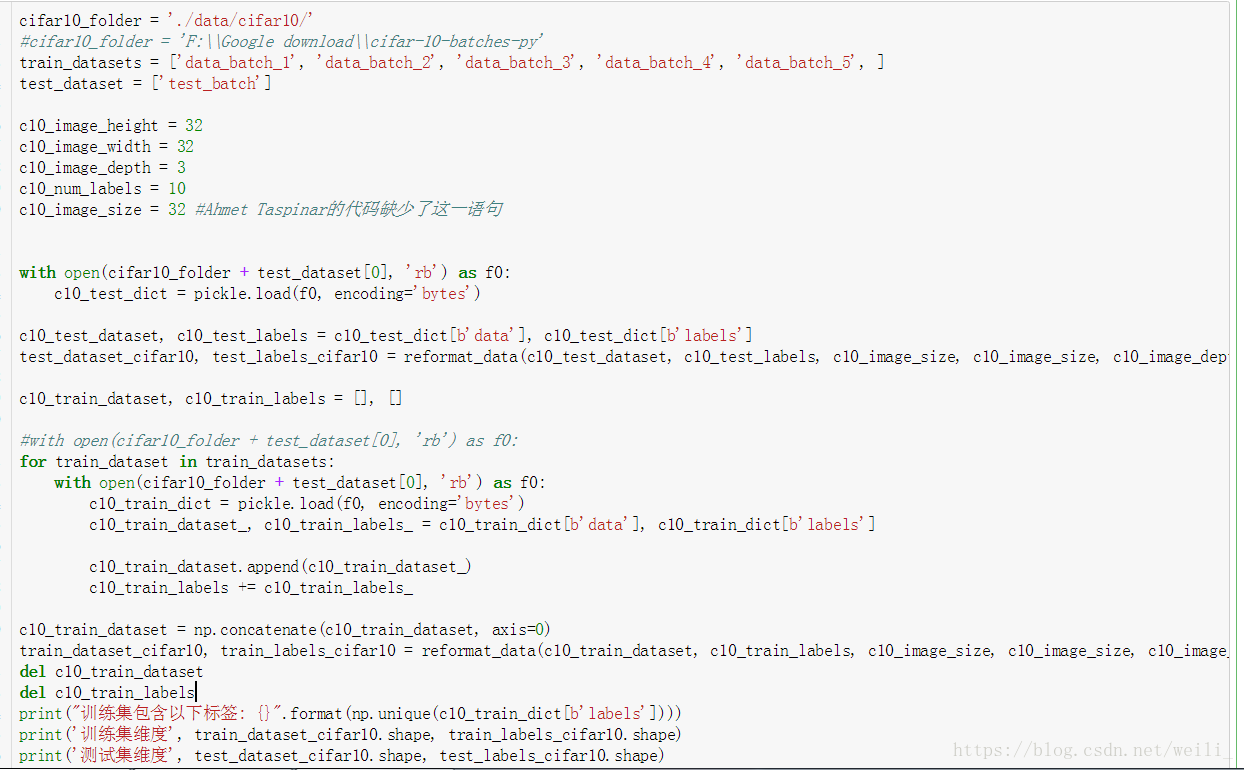