相关文章:
1 理论
AlexNet是一个8层的深度卷积网络,主要应用于图像。
文章创新点:
- 针对数据过少,提出了扩增方法,对图片裁剪/翻转等
- 对于激活函数,采用ReLU代替Sigmoid,克服Sigmoid函数在接近0和1时难以训练的问题
- 针对网络过拟合,提出Dropout
- 对于模型结构,改变了卷积核大小/网络层数/步长等
模型结构:5层卷积,第一层卷积核为 11 ∗ 11 11*11 11∗11、第二层为 5 ∗ 5 5*5 5∗5之外,其余三层均为 3 ∗ 3 3*3 3∗3。
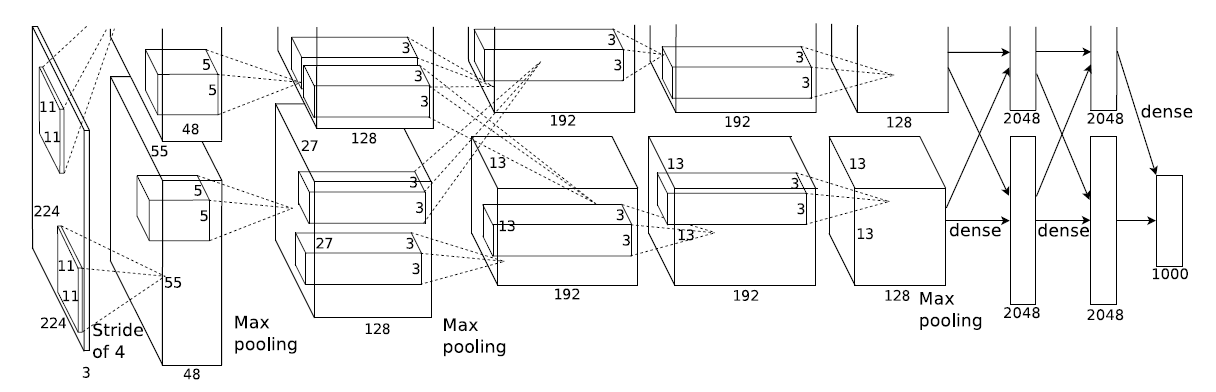
具体结构:
第一层:卷积层
卷积核大小 11 ∗ 11 11*11 11∗11,输入通道数根据输入图像而定,输出通道数为96,步长为4。
池化层窗口大小为 3 ∗ 3 3*3 3∗3,步长为2。
第二层:卷积层
卷积核大小 5 ∗ 5 5*5 5∗5,输入通道数为96,输出通道数为256,步长为2。
池化层窗口大小为 3 ∗ 3 3*3 3∗3,步长为2。
第三层:卷积层
卷积核大小 3 ∗ 3 3*3 3∗3,输入通道数为256,输出通道数为384,步长为1。
第四层:卷积层
卷积核大小 3 ∗ 3 3*3 3∗3,输入通道数为384,输出通道数为384,步长为1。
第五层:卷积层
卷积核大小 3 ∗ 3 3*3 3∗3,输入通道数为384,输出通道数为256,步长为1。
池化层窗口大小为 3 ∗ 3 3*3 3∗3,步长为2。
第六层:全连接层
输入大小为上一层的输出,输出大小为4096。
Dropout概率为0.5。
第七层:全连接层
输入大小为4096,输出大小为4096。
Dropout概率为0.5。
第八层:全连接层
输入大小为4096,输出大小为分类数。
注意:需要注意一点,5个卷积层中前2个卷积层后面都会紧跟一个池化层,而第3、4层卷积层后面没有池化层,而是连续3、4、5层三个卷积层后才加入一个池化层。
2 实践
# ImageNet Classification with Deep Convolutional Neural Networks
import torch.nn as nn
import torch
class AlexNet(nn.Module):
def __init__(self, input_channel, n_classes):
super(AlexNet, self).__init__()
self.conv1 = nn.Sequential(
# transforming (bsize x 1 x 224 x 224) to (bsize x 96 x 54 x 54)
# From floor((n_h - k_s + p + s)/s), floor((224 - 11 + 3 + 4) / 4) => floor(219/4) => floor(55.5) => 55
nn.Conv2d(input_channel, 96, kernel_size=11, stride=4, padding=3), # (batch_size * 96 * 55 * 55)
nn.ReLU(inplace=True),
nn.MaxPool2d(kernel_size=3, stride=2)) # (batch_size * 96 * 27 * 27)
self.conv2 = nn.Sequential(
nn.Conv2d(96, 256, kernel_size=5, padding=2), # (batch_size * 256 * 27 * 27)
nn.ReLU(inplace=True),
nn.MaxPool2d(kernel_size=3, stride=2)) # (batch_size * 256 * 13 * 13)
self.conv3 = nn.Sequential(
nn.Conv2d(256, 384, kernel_size=3, padding=1), # (batch_size * 384 * 13 * 13)
nn.ReLU(inplace=True),
nn.Conv2d(384, 384, kernel_size=3, padding=1), # (batch_size * 384 * 13 * 13)
nn.ReLU(inplace=True),
nn.Conv2d(384, 256, kernel_size=3, padding=1), # (batch_size * 256 * 13 * 13)
nn.ReLU(inplace=True),
nn.MaxPool2d(kernel_size=3, stride=2), # (batch_size * 256 * 6 * 6)
nn.Flatten())
self.fc = nn.Sequential(
nn.Linear(256 * 6 * 6, 4096), # (batch_size * 4096)
nn.ReLU(inplace=True),
nn.Dropout(p=0.5),
nn.Linear(4096, 4096), # (batch_size * 4096)
nn.ReLU(inplace=True),
nn.Dropout(p=0.5),
nn.Linear(4096, n_classes)) # (batch_size * 10)
self.conv1.apply(self.init_weights)
self.conv2.apply(self.init_weights)
self.conv3.apply(self.init_weights)
self.fc.apply(self.init_weights)
def init_weights(self, layer):
if type(layer) == nn.Linear or type(layer) == nn.Conv2d:
nn.init.xavier_uniform_(layer.weight)
def forward(self, x):
out = self.conv1(x)
out = self.conv2(out)
out = self.conv3(out)
out = self.fc(out)
return out
if __name__ == '__main__':
data = torch.randn(size=(16, 3, 224, 224))
model = AlexNet(input_channel=3, n_classes=10)
classes = model(data)
print(classes.size()) # (16,10)
参考:https://zhuanlan.zhihu.com/p/86447716