本文作者系360奇舞团前端开发工程师
随着各种大型语言模型 (LLM
)的开源, 开发人员也意识到向量数据库越来越重要,因为它可以进一步增强这些模型。像 GPT
这种LLM
大模型,通常使用大规模的嵌入向量来表示文本数据,而 Vector DB
提供了高效存储和检索向量数据的能力,使得在应用中存储和访问这些向量变得更加高效和快速。
向量数据库
向量数据库可以索引、存储结构化或非结构化数据(例如文本或图像)及其向量嵌入(数据的数字表示)并提供对它们的访问。它允许用户在生产环境中快速大规模地查找和检索相似的对象。由于其高效的搜索功能,向量数据库有时也称为向量搜索引擎。
向量嵌入
现实生活中的数据有结构化的数据,如电子表格这种组织整齐的数据,也有非结构化的数据如文档、图像、音视频等,且绝大多数都是非结构化数据。这类数据很难以有组织的方式存储它们,以便我们可以轻松的找到所需的内容。如何对这些数据进行量化存储,并能够快速查找出来呢?
向量嵌入是一种将非结构化数据转换为数学向量的方法,使得数据可以被量化存储并且可以进行快速查找。通过向量嵌入,非结构化数据(如文档、图像、音视频等)被映射到一个多维的向量空间中,每个数据项都用一个向量来表示。这些向量通常被设计成能够捕捉数据的语义信息,使得相似的数据在向量空间中距离较近,不相似的数据在向量空间中距离较远。
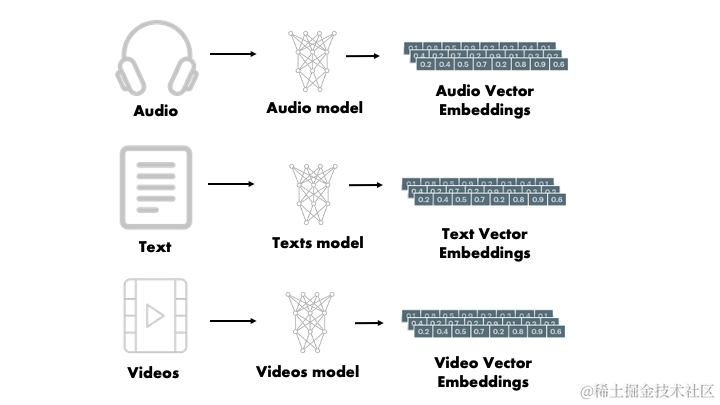
现代机器学习模型可以经过训练将原始数据转换为嵌入,表示为固定维度的浮点数数组(或向量)。向量数据库便是用于存储这些嵌入向量的专用数据库
向量搜索
使用向量嵌入,我们可以在向量空间中搜索彼此接近的对象,以找到并检索相似的对象。这种搜索方式被称为向量搜索、相似性搜索或语义搜索。
具体向量搜索是如何进行的,举个例子来讲:我们基于人格五因素测试对多人进行性格测试,在5
个维度上收集了他们的评分,并将这些评分作为向量嵌入;
为方便在平面坐标系中展示向量之间的关系,我们先只取2
个维度的数据,将评分范围调到 -1 ~ 1
,并将这些点表示为从坐标原点到它们的向量:
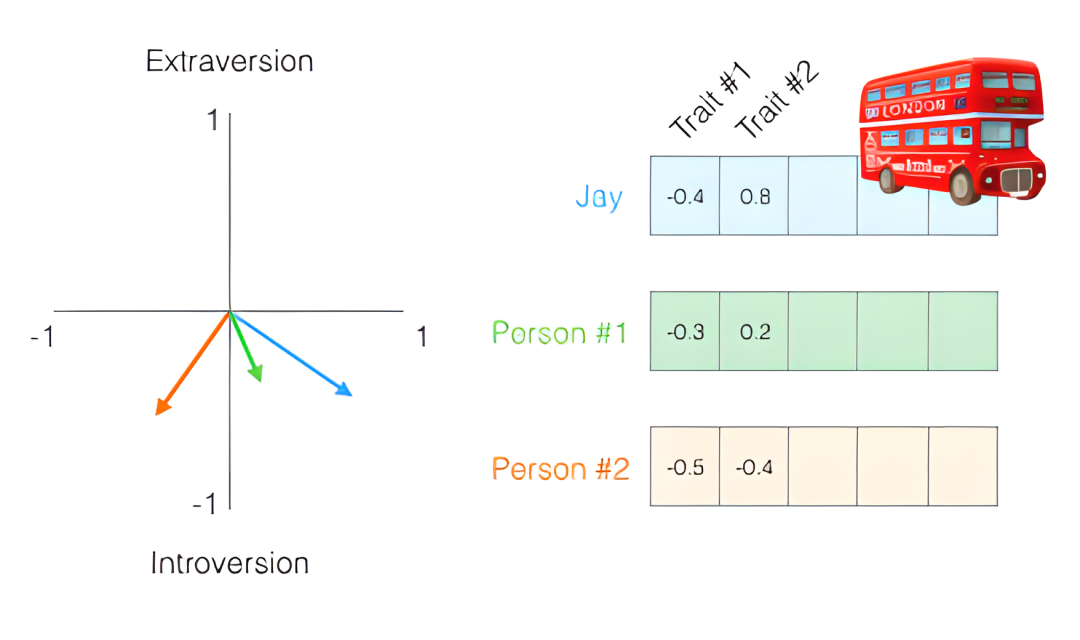
如果Jay
被车撞了,我们需要通过向量搜索找到一个与其性格相似的人去代替,那么上图中哪个人与Jay
相似?机器如何识别呢?这时向量的表示法就可以让我们通过数学方式计算向量的相似性,来找到了性格相似的人。
向量的相似性通常通过计算两个向量之间的距离来确定。计算相似度分数的常用方法是 余弦相似度, 其数学定义与公式如下:
给定两个 n
维属性向量 A
和 B
,它们的余弦相似度 cos(θ)
通过使用向量点积和向量长度表示为:

其中,Ai
和 Bi
分别是向量 A
和 B
的第 i
个分量。
按照数学公式我们可以计算出Person #1
和Jay
相似度最高,两个向量的夹角也最小,因此他们更相似:
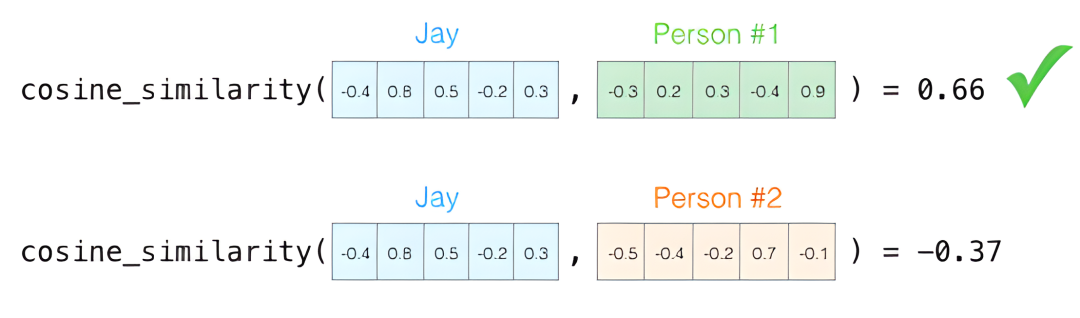
实际上两个维度的特征,不足以判断人格是否相似,因此我们需要用到5
个维度的数据:
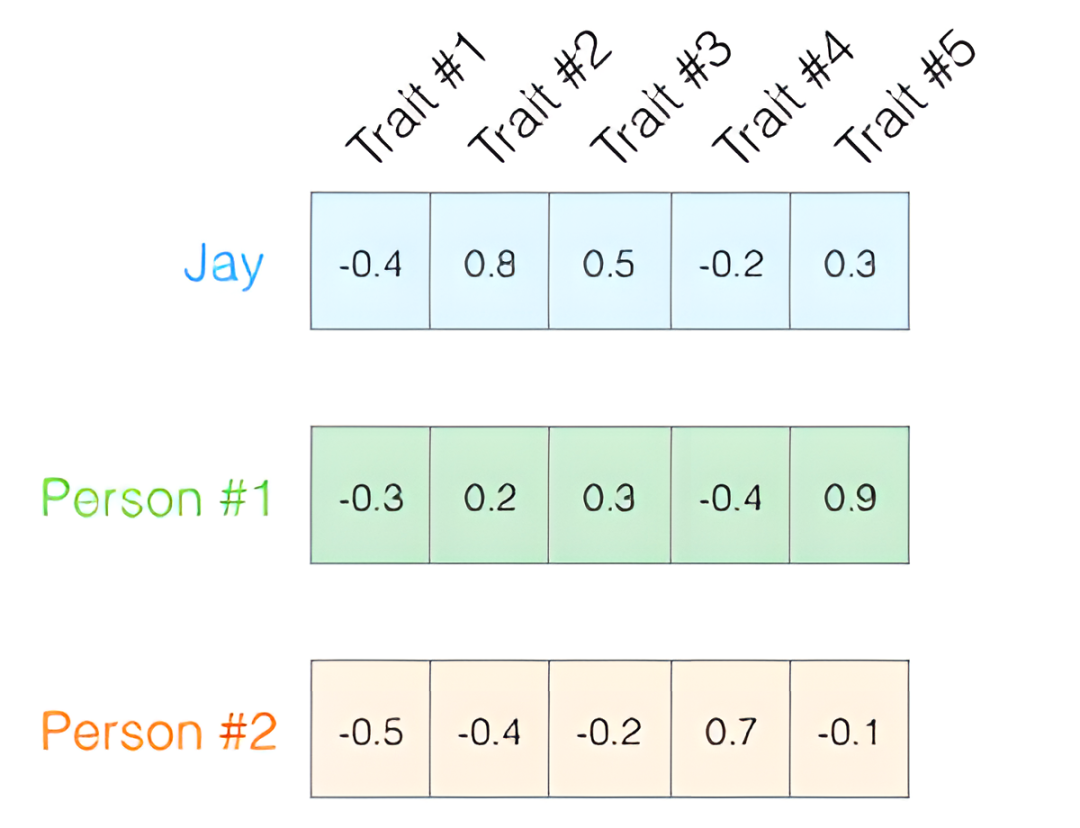
虽然数据维度升高,但这种比较向量相似度的方法,适用于任意维度的向量空间:
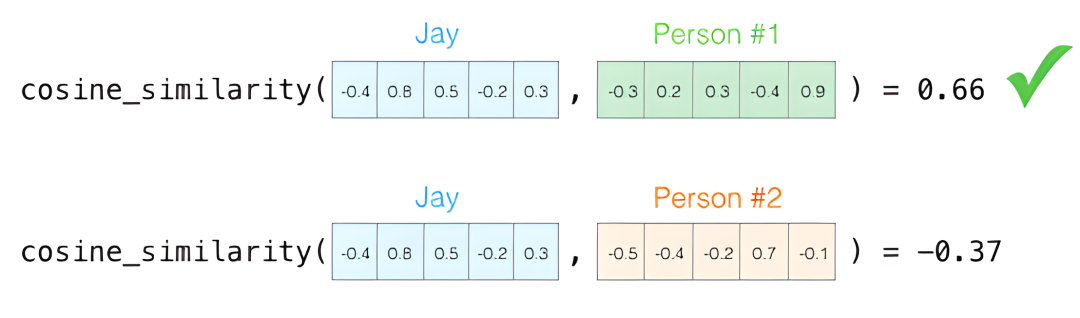
向量索引
向量索引是一种用于在大规模数据集中快速搜索相似向量的技术。通俗地说,就像我们在一堆杂乱的图片中找到与给定图片相似的图片一样。构建向量索引就像是给大量的照片贴上标签并整理成相册一样。
想象一下,你有一个非常大的图像库,每张图片都表示为一个向量。现在,你希望找到与给定图片相似的图片。这时,向量索引就派上了用场。可以根据给定图片的向量,在多维数据空间中快速定位相册,然后快速定位那些与给定图片在向量空间中距离较近的图片,而不必一个一个地比较每张图片。
常见的构建索引的算法:
基于聚类的索引 (如,FAISS)
基于邻近图的索引 (如,HNSW)
基于树的索引 (如,ANNOY)
基于哈希的索引 (如,LSH)
基于压缩的索引 (如,PQ 或 SCANN)
向量索引在实际中有许多应用,比如图像搜索、语义搜索、推荐系统等。它能够帮助我们快速地处理和检索大规模的向量数据,从而在很多领域提高效率和性能。
工作流程
向量数据库的常见的工作管道:

索引:对嵌入的向量是通索引算法对其进行索引并将向量数据和构建好的索引结构存储到数据库中。
查询:向量数据库会根据查询向量在向量索引中进行搜索,并返回与查询向量最相似的向量结果。
后期处理:在某些情况下,向量数据库从数据集中检索出一组与查询向量最相似的向量,并对它们进行后期处理,以返回最终的结果。这个步骤可能包括使用不同的相似度度量重新对查询到的相似向量进行排序。换句话说,就像在购物网站上找到了一些可能的商品,然后根据不同的标准重新排序,以便更好地满足用户的需求。
Chroma
示例
Chroma DB 是一个开源矢量存储,用于存储和检索矢量嵌入。它的主要用途是保存嵌入以及元数据,交由LLM模型使用。此外,它还可以用于文本数据的语义搜索引擎。
工作流程:
创建
collection
(类似关系型数据库中的表)使用元数据和唯一的ID将文本添加到集合中。
通过文本或嵌入查询集合。也可以通过元数据过滤结果。
测试数据
employee_info = """
James Smith, a 32-year-old software engineer with 8 years of experience,
is a member of the development and hiking clubs who enjoys cooking, photography, and playing guitar
in his free time. He aspires to lead a tech team after gaining more expertise in his field
at XYZ Corporation.
"""
department_info = """
The development department at XYZ Corporation is responsible for designing, implementing,
and maintaining the software products of the company. It consists of skilled professionals
ranging from software engineers to UX designers, working collaboratively to innovate
and deliver high-quality solutions to clients' needs.
"""
company_info = """
XYZ Corporation, established in 2005 in Silicon Valley, is a leading software development company
specializing in creating cutting-edge solutions for various industries. With over 1000 employees
and offices in multiple countries, XYZ Corp is known for its commitment to excellence and innovation
in the tech sector.
"""
默认情况下Chroma
使用all-MiniLM-L6-v2
模型将文字转换为向量:
import chromadb
chroma_client = chromadb.Client()
# 创建集合
collection = chroma_client.create_collection(name="employee")
# 添加到集合
collection.add(documents=[employee_info, department_info, company_info],
metadatas=[{"source": "employee info"}, {"source": "department info"}, {"source": "company info"}],
ids=["id1", "id2", "id3"]
)
添加数据到集合时便会自动下载all-MiniLM-L6-v2
模型,并将文本转为嵌入到simple_employee_info
集合中。
from chromadb.utils import embedding_functions
def_efn = embedding_functions.DefaultEmbeddingFunction()
embedding_text = def_efn(['早上','好'])
# [[-0.045326605439186096, 0.004748976789414883, 0.02001281827688217, 0.007265775464475155, -0.0672430619597435, ...]]
查询:相似性搜索
# 相似性搜索
results = collection.query(
query_texts=["What is the employee's name?"],
n_results=2
)
# 输出两个相似的结果
# {
# "ids": [["id1", "id2"]],
# "distances": [[1.6440917253494263, 1.6728706359863281]],
# "metadatas": [[{"source": "department info"}, {"source": "employee info"}]],
# "embeddings": None,
# "documents": [
# [
# "The development department at XYZ Corporation is responsible for designing, implementing...."
# "James Smith, a 32-year-old software engineer with 8 years of experience.......",
# ]
# ],
# "uris": None,
# "data": None
# }
查询过滤器
# 在匹配的元数据中查找
results = collection.query(
query_texts=["What is the employee's name?"],
n_results=2,
where={"source":{"$in":["employee info","company info"]}}
)
# 依据文档的内容进行过滤
# results = collection.query(
# query_texts=["What is the employee's name?"],
# n_results=2,
# where_document={"$contains":"engineer"}
# )
也可以不使用默认的嵌入模型:
from chromadb.utils import embedding_functions
ollama_ef = embedding_functions.OllamaEmbeddingFunction(
url="http://localhost:11434/api/embeddings",
model_name="llama3",
)
employees_embeddings = ollama_ef([employee_info, department_info, company_info])
# 嵌入的向量
# [[0.9733191728591919, -2.4074814319610596, -1.0328993797302246, -0.11499451100826263,
# 2.668761730194092, 0.3950701355934143, 0.8115649223327637, -0.058548394590616226,
# -3.9989635944366455, -2.2536189556121826, -0.9562288522720337, 2.3301892280578613,...]]
collection2 = chroma_client.get_or_create_collection(name="other_employee",
embedding_function=ollama_ef)
#添加嵌入的向量
collection2.add(embeddings=employees_embeddings,
documents=[employee_info, department_info, company_info],
metadatas=[{"source": "employee info"}, {"source": "department info"}, {"source": "company info"}],
ids=["id1", "id2", "id3"]
)
result2 = collection2.query(query_texts=["What is the employee's name?"],
n_results=2)
# 查询输出
# {
# "ids": [["id1", "id3"]],
# "distances": [[27612.076171875, 29216.796875]],
# "metadatas": [[{"source": "employee info"}, {"source": "company info"}]],
# "embeddings": None,
# "documents": [
# [
# "James Smith, a 32-year-old software engineer with 8 years of experience, ....",
# "XYZ Corporation, established in 2005 in Silicon Valley, is a leading software development company specializing...."
# ]
# ],
# "uris": None,
# "data": None
# }
和默认的词嵌入模型不同的是,相似度匹配到了employee_info
和company_info
。不同的词嵌入模型执行查询时会有不一样的效果。
更新数据
# 更新
collection.update(ids=["id1"],
documents=["Bob, a 28-year-old test engineer with 4 years of experience"],
metadatas=[{"source":"employee info"}],
)
# 相似性搜索
results = collection.query(
query_texts=["What is the employee's name?"],
n_results=2
)
# 输出的员工信息变为了 `Bob`
# {
# "ids": [["id1", "id2"]],
# "distances": [[1.4391101598739624, 1.6440917253494263]],
# "metadatas": [[{"source": "employee info"}, {"source": "department info"}]],
# "embeddings": None,
# "documents": [
# [
# "Bob, a 28-year-old test engineer with 4 years of experience",
# "The development department at XYZ Corporation is responsible for designing, implementing,\nand maintaining the software products of the company. It consists of skilled professionals\nranging from software engineers to UX designers, working collaboratively to innovate\nand deliver high-quality solutions to clients' needs.\n"
# ]
# ],
# "uris": None,
# "data": None
# }
删除数据
print(f"删除前集合有 {collection.count()} 条记录") #删除前集合有 3 条记录
collection.delete(ids=["id1"])
print(f"删除后集合有 {collection.count()} 条记录")#删除后集合有 2 条记录
参考
https://partee.io/2022/08/11/vector-embeddings/
https://www.pinecone.io/learn/vector-database/
https://jalammar.github.io/illustrated-word2vec/
https://zilliz.com/blog/similarity-metrics-for-vector-search
https://weaviate.io/blog/what-is-a-vector-database
- END -
如果您关注前端+AI 相关领域可以扫码加群交流
关于奇舞团
奇舞团是 360 集团最大的大前端团队,非常重视人才培养,有工程师、讲师、翻译官、业务接口人、团队 Leader 等多种发展方向供员工选择,并辅以提供相应的技术力、专业力、通用力、领导力等培训课程。奇舞团以开放和求贤的心态欢迎各种优秀人才关注和加入奇舞团。