首先将caffe的根目录作为当前目录,然后加载caffe程序自带的小猫图片,并显示。
图片大小为360x480,三通道
import numpy as np
import matplotlib.pyplot as plt #matplotlib inline
import caffe
caffe_root='/caffe/'
import os,sys
os.chdir(caffe_root)
sys.path.insert(0,caffe_root+'python')
im = caffe.io.load_image('examples/images/cat.jpg')
print(im.shape)
plt.imshow(im)
plt.axis('off')
plt.show()
打开examples/net_surgery/conv.prototxt文件,修改两个地方
一是将input_shape由原来的是(1,1,100,100)修改为(1,3,100,100),即由单通道灰度图变为三通道彩色图。
二是将过滤器个数(num_output)由3修改为16,多增加一些filter, 当然保持原来的数不变也行。
其它地方不变,修改后的prototxt如下:只有一个卷积层
# Simple single-layer network to showcase editing model parameters.
name: "convolution"
input: "data"
input_shape {
dim: 1
dim: 3
dim: 100
dim: 100
}
layer {
name: "conv"
type: "Convolution"
bottom: "data"
top: "conv"
convolution_param {
num_output: 16
kernel_size: 5
stride: 1
weight_filler {
type: "gaussian"
std: 0.01
}
bias_filler {
type: "constant"
value: 0
}
}
}
将图片数据加载到blobs,但反过来,我们也可以反过来从blob中提取出原始数据,并进行显示。
显示的时候要注意各维的顺序,如blobs的顺序是(1,3,360,480),从前往后分别表示1张图片,3三个通道,
图片大小为360x480,需要调用transpose改变为(360,480,3)才能正常显示。
其中用data[0]表示第一张图片,下标从0开始,此例只有一张图片,因此只能是data[0].
分别用data[0,0],data[0,1]和data[0,2]表示该图片的三个通道。
import numpy as np
import matplotlib.pyplot as plt #matplotlib inline
import caffe
caffe_root='/caffe/'
import os,sys
os.chdir(caffe_root)
sys.path.insert(0,caffe_root+'python')
im = caffe.io.load_image('examples/images/cat.jpg')
net = caffe.Net('examples/net_surgery/conv.prototxt', caffe.TEST)
im_input=im[np.newaxis,:,:,:].transpose(0,3,1,2)
print("data-blobs:",im_input.shape)
net.blobs['data'].reshape(*im_input.shape)
net.blobs['data'].data[...] = im_input
plt.imshow(net.blobs['data'].data[0].transpose(1,2,0))
plt.axis('off')
plt.show()
结果如下:
WARNING: Logging before InitGoogleLogging() is written to STDERR
I0608 17:05:22.288508 12163 net.cpp:51] Initializing net from parameters:
name: "convolution"
state {
phase: TEST
level: 0
}
layer {
name: "data"
type: "Input"
top: "data"
input_param {
shape {
dim: 1
dim: 3
dim: 100
dim: 100
}
}
data-blobs: (1, 3, 360, 480)
}
layer {
name: "conv"
type: "Convolution"
bottom: "data"
top: "conv"
convolution_param {
num_output: 16
kernel_size: 5
stride: 1
weight_filler {
type: "gaussian"
std: 0.01
}
bias_filler {
type: "constant"
value: 0
}
}
}
I0608 17:05:22.288563 12163 layer_factory.hpp:77] Creating layer data
I0608 17:05:22.288576 12163 net.cpp:84] Creating Layer data
I0608 17:05:22.288585 12163 net.cpp:380] data -> data
I0608 17:05:22.288609 12163 net.cpp:122] Setting up data
I0608 17:05:22.288622 12163 net.cpp:129] Top shape: 1 3 100 100 (30000)
I0608 17:05:22.288630 12163 net.cpp:137] Memory required for data: 120000
I0608 17:05:22.288635 12163 layer_factory.hpp:77] Creating layer conv
I0608 17:05:22.288820 12163 net.cpp:84] Creating Layer conv
I0608 17:05:22.288830 12163 net.cpp:406] conv <- data
I0608 17:05:22.288841 12163 net.cpp:380] conv -> conv
I0608 17:05:22.289319 12163 net.cpp:122] Setting up conv
I0608 17:05:22.289333 12163 net.cpp:129] Top shape: 1 16 96 96 (147456)
I0608 17:05:22.289340 12163 net.cpp:137] Memory required for data: 709824
I0608 17:05:22.289360 12163 net.cpp:200] conv does not need backward computation.
I0608 17:05:22.289366 12163 net.cpp:200] data does not need backward computation.
I0608 17:05:22.289372 12163 net.cpp:242] This network produces output conv
I0608 17:05:22.289381 12163 net.cpp:255] Network initialization done.
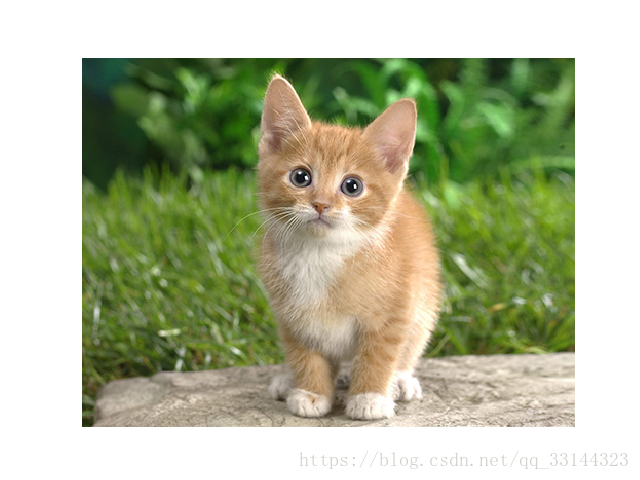
编写一个show_data函数来显示数据,从blobs数据中将原始图片提取出来,并分别显示不同的通道图
import numpy as np
import matplotlib.pyplot as plt #matplotlib inline
import caffe
caffe_root='/caffe/'
import os,sys
def show_data(data, head, padsize=1, padval=0):
data -= data.min()
data /= data.max()
# force the number of filters to be square
n = int(np.ceil(np.sqrt(data.shape[0])))
padding = ((0, n ** 2 - data.shape[0]), (0, padsize), (0, padsize)) + ((0, 0),) * (data.ndim - 3)
data = np.pad(data, padding, mode='constant', constant_values=(padval, padval))
# tile the filters into an image
data = data.reshape((n, n) + data.shape[1:]).transpose((0, 2, 1, 3) + tuple(range(4, data.ndim + 1)))
data = data.reshape((n * data.shape[1], n * data.shape[3]) + data.shape[4:])
plt.figure()
plt.title(head)
plt.imshow(data)
plt.axis('off')
if __name__ == '__main__':
os.chdir(caffe_root)
sys.path.insert(0, caffe_root + 'python')
im = caffe.io.load_image('examples/images/cat.jpg')
net = caffe.Net('examples/net_surgery/conv.prototxt', caffe.TEST)
im_input = im[np.newaxis, :, :, :].transpose(0, 3, 1, 2)
net.blobs['data'].reshape(*im_input.shape)
net.blobs['data'].data[...] = im_input
plt.rcParams['image.cmap'] = 'gray'
print("data-blobs:", net.blobs['data'].data.shape)
show_data(net.blobs['data'].data[0], 'origin images')
plt.show()
结果如下:
data-blobs: (1, 3, 360, 480)
WARNING: Logging before InitGoogleLogging() is written to STDERR
I0608 17:15:34.040977 12859 net.cpp:51] Initializing net from parameters:
name: "convolution"
state {
phase: TEST
level: 0
}
layer {
name: "data"
type: "Input"
top: "data"
input_param {
shape {
dim: 1
dim: 3
dim: 100
dim: 100
}
}
}
layer {
name: "conv"
type: "Convolution"
bottom: "data"
top: "conv"
convolution_param {
num_output: 16
kernel_size: 5
stride: 1
weight_filler {
type: "gaussian"
std: 0.01
}
bias_filler {
type: "constant"
value: 0
}
}
}
I0608 17:15:34.041028 12859 layer_factory.hpp:77] Creating layer data
I0608 17:15:34.041043 12859 net.cpp:84] Creating Layer data
I0608 17:15:34.041051 12859 net.cpp:380] data -> data
I0608 17:15:34.041075 12859 net.cpp:122] Setting up data
I0608 17:15:34.041090 12859 net.cpp:129] Top shape: 1 3 100 100 (30000)
I0608 17:15:34.041095 12859 net.cpp:137] Memory required for data: 120000
I0608 17:15:34.041102 12859 layer_factory.hpp:77] Creating layer conv
I0608 17:15:34.041116 12859 net.cpp:84] Creating Layer conv
I0608 17:15:34.041123 12859 net.cpp:406] conv <- data
I0608 17:15:34.041134 12859 net.cpp:380] conv -> conv
I0608 17:15:34.041601 12859 net.cpp:122] Setting up conv
I0608 17:15:34.041615 12859 net.cpp:129] Top shape: 1 16 96 96 (147456)
I0608 17:15:34.041620 12859 net.cpp:137] Memory required for data: 709824
I0608 17:15:34.041640 12859 net.cpp:200] conv does not need backward computation.
I0608 17:15:34.041647 12859 net.cpp:200] data does not need backward computation.
I0608 17:15:34.041653 12859 net.cpp:242] This network produces output conv
I0608 17:15:34.041661 12859 net.cpp:255] Network initialization done.
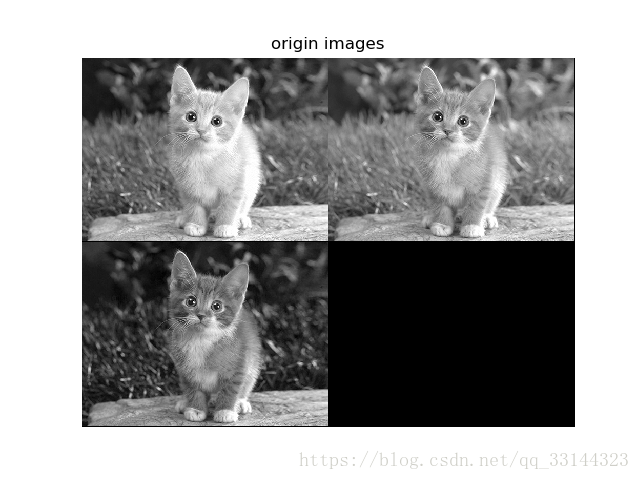
调用forward()执行卷积操作,blobs数据发生改变。由原来的(1,3,360,480)变为(1,16,356,476)。
并初始化生成了相应的权值,权值数据为(16,3,5,5)。
最后调用两次show_data来分别显示权值和卷积过滤后的16通道图片。
import numpy as np
import matplotlib.pyplot as plt #matplotlib inline
import caffe
caffe_root='/caffe/'
import os,sys
def show_data(data, head, padsize=1, padval=0):
data -= data.min()
data /= data.max()
# force the number of filters to be square
n = int(np.ceil(np.sqrt(data.shape[0])))
padding = ((0, n ** 2 - data.shape[0]), (0, padsize), (0, padsize)) + ((0, 0),) * (data.ndim - 3)
data = np.pad(data, padding, mode='constant', constant_values=(padval, padval))
# tile the filters into an image
data = data.reshape((n, n) + data.shape[1:]).transpose((0, 2, 1, 3) + tuple(range(4, data.ndim + 1)))
data = data.reshape((n * data.shape[1], n * data.shape[3]) + data.shape[4:])
plt.figure()
plt.title(head)
plt.imshow(data)
plt.axis('off')
if __name__ == '__main__':
os.chdir(caffe_root)
sys.path.insert(0, caffe_root + 'python')
im = caffe.io.load_image('examples/images/cat.jpg')
net = caffe.Net('examples/net_surgery/conv.prototxt', caffe.TEST)
im_input = im[np.newaxis, :, :, :].transpose(0, 3, 1, 2)
net.blobs['data'].reshape(*im_input.shape)
net.blobs['data'].data[...] = im_input
plt.rcParams['image.cmap'] = 'gray'
net.forward()
print("data-blobs:", net.blobs['data'].data.shape)
print("conv-blobs:", net.blobs['conv'].data.shape)
print("weight-blobs:", net.params['conv'][0].data.shape)
show_data(net.params['conv'][0].data[:, 0], 'conv weights(filter)')
show_data(net.blobs['conv'].data[0], 'post-conv images')
plt.show()
结果如下:
WARNING: Logging before InitGoogleLogging() is written to STDERR
I0608 19:44:21.352882 20892 net.cpp:51] Initializing net from parameters:
name: "convolution"
state {
phase: TEST
level: 0
}
layer {
name: "data"
type: "Input"
top: "data"
input_param {
shape {
dim: 1
dim: 3
dim: 100
dim: 100
}
}
}
layer {
name: "conv"
type: "Convolution"
bottom: "data"
top: "conv"
convolution_param {
num_output: 16
kernel_size: 5
stride: 1
weight_filler {
type: "gaussian"
std: 0.01
}
bias_filler {
type: "constant"
value: 0
}
}
}
I0608 19:44:21.352941 20892 layer_factory.hpp:77] Creating layer data
I0608 19:44:21.352958 20892 net.cpp:84] Creating Layer data
I0608 19:44:21.352969 20892 net.cpp:380] data -> data
I0608 19:44:21.352996 20892 net.cpp:122] Setting up data
I0608 19:44:21.353011 20892 net.cpp:129] Top shape: 1 3 100 100 (30000)
I0608 19:44:21.353018 20892 net.cpp:137] Memory required for data: 120000
I0608 19:44:21.353026 20892 layer_factory.hpp:77] Creating layer conv
I0608 19:44:21.353044 20892 net.cpp:84] Creating Layer conv
I0608 19:44:21.353052 20892 net.cpp:406] conv <- data
I0608 19:44:21.353065 20892 net.cpp:380] conv -> conv
I0608 19:44:21.353521 20892 net.cpp:122] Setting up conv
I0608 19:44:21.353538 20892 net.cpp:129] Top shape: 1 16 96 96 (147456)
I0608 19:44:21.353545 20892 net.cpp:137] Memory required for data: 709824
I0608 19:44:21.353565 20892 net.cpp:200] conv does not need backward computation.
I0608 19:44:21.353574 20892 net.cpp:200] data does not need backward computation.
I0608 19:44:21.353581 20892 net.cpp:242] This network produces output conv
I0608 19:44:21.353590 20892 net.cpp:255] Network initialization done.
data-blobs: (1, 3, 360, 480)
conv-blobs: (1, 16, 356, 476)
weight-blobs: (16, 3, 5, 5)
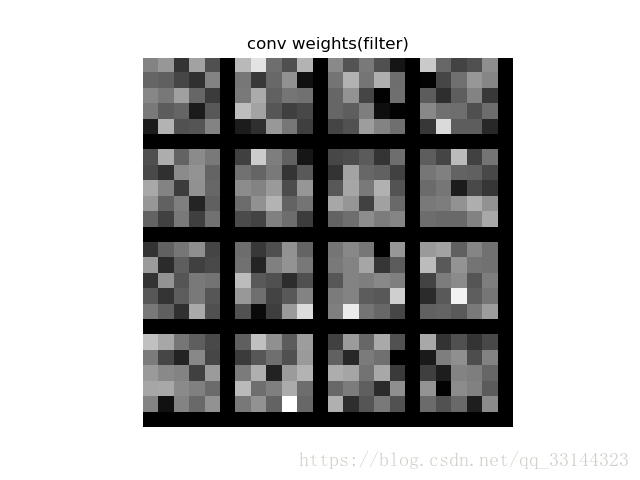
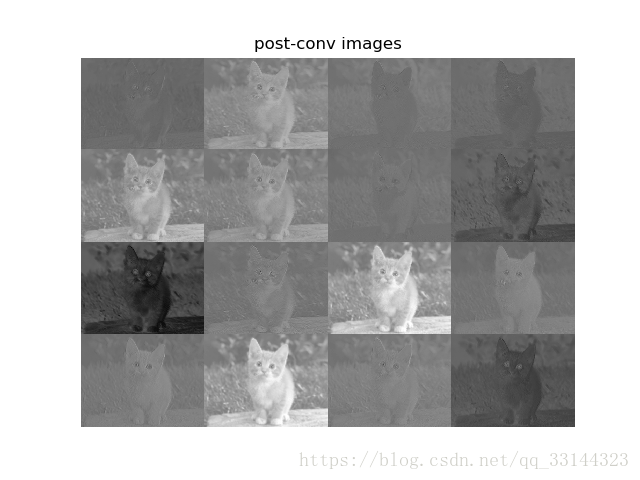