本文使用的剪枝库是torch-pruning ,实验了该库的三个剪枝算法GroupNormPruner、BNScalePruner和GrowingRegPruner。
安装使用
- 安装依赖库
pip install torch-pruning
- 把 https://github.com/VainF/Torch-Pruning/blob/master/examples/yolov8/yolov8_pruning.py,文件拷贝到yolov8的根目录下。或者使用我的剪枝代码,在原有的基础上稍作修改,保存了不同剪枝阶段的模型。注意:稀疏化训练这个参数is_regularize不要开启,开启了会报错。这个需要读者把pruner传进源代码里才可以正常进行稀疏训练
import argparse
import math
import os
os.environ["CUDA_VISIBLE_DEVICES"] = "4"
from copy import deepcopy
from datetime import datetime
from pathlib import Path
from typing import List, Union
import numpy as np
import torch
import torch.nn as nn
from matplotlib import pyplot as plt
from ultralytics import YOLO, __version__
from ultralytics.nn.modules import Detect, C2f, Conv, Bottleneck
from ultralytics.nn.tasks import attempt_load_one_weight
from ultralytics.yolo.engine.model import TASK_MAP
from ultralytics.yolo.engine.trainer import BaseTrainer
from ultralytics.yolo.utils import yaml_load, LOGGER, RANK, DEFAULT_CFG_DICT, DEFAULT_CFG_KEYS
from ultralytics.yolo.utils.checks import check_yaml
from ultralytics.yolo.utils.torch_utils import initialize_weights, de_parallel
import torch_pruning as tp
def save_pruning_performance_graph(x, y1, y2, y3):
"""
Draw performance change graph
Parameters
----------
x : List
Parameter numbers of all pruning steps
y1 : List
mAPs after fine-tuning of all pruning steps
y2 : List
MACs of all pruning steps
y3 : List
mAPs after pruning (not fine-tuned) of all pruning steps
Returns
-------
"""
try:
plt.style.use("ggplot")
except:
pass
x, y1, y2, y3 = np.array(x), np.array(y1), np.array(y2), np.array(y3)
y2_ratio = y2 / y2[0]
fig, ax = plt.subplots(figsize=(8, 6))
ax.set_xlabel('Pruning Ratio')
ax.set_ylabel('mAP')
ax.plot(x, y1, label='recovered mAP')
ax.scatter(x, y1)
ax.plot(x, y3, color='tab:gray', label='pruned mAP')
ax.scatter(x, y3, color='tab:gray')
ax2 = ax.twinx()
ax2.set_ylabel('MACs')
ax2.plot(x, y2_ratio, color='tab:orange', label='MACs')
ax2.scatter(x, y2_ratio, color='tab:orange')
lines, labels = ax.get_legend_handles_labels()
lines2, labels2 = ax2.get_legend_handles_labels()
ax2.legend(lines + lines2, labels + labels2, loc='best')
ax.set_xlim(105, -5)
ax.set_ylim(0, max(y1) + 0.05)
ax2.set_ylim(0.05, 1.05)
max_y1_idx = np.argmax(y1)
min_y1_idx = np.argmin(y1)
max_y2_idx = np.argmax(y2)
min_y2_idx = np.argmin(y2)
max_y1 = y1[max_y1_idx]
min_y1 = y1[min_y1_idx]
max_y2 = y2_ratio[max_y2_idx]
min_y2 = y2_ratio[min_y2_idx]
ax.text(x[max_y1_idx], max_y1 - 0.05, f'max mAP = {max_y1:.2f}', fontsize=10)
ax.text(x[min_y1_idx], min_y1 + 0.02, f'min mAP = {min_y1:.2f}', fontsize=10)
ax2.text(x[max_y2_idx], max_y2 - 0.05, f'max MACs = {max_y2 * y2[0] / 1e9:.2f}G', fontsize=10)
ax2.text(x[min_y2_idx], min_y2 + 0.02, f'min MACs = {min_y2 * y2[0] / 1e9:.2f}G', fontsize=10)
plt.title('Comparison of mAP and MACs with Pruning Ratio')
plt.savefig('pruning_perf_change.png')
def infer_shortcut(bottleneck):
c1 = bottleneck.cv1.conv.in_channels
c2 = bottleneck.cv2.conv.out_channels
return c1 == c2 and hasattr(bottleneck, 'add') and bottleneck.add
class C2f_v2(nn.Module):
def __init__(self, c1, c2, n=1, shortcut=False, g=1, e=0.5):
super().__init__()
self.c = int(c2 * e)
self.cv0 = Conv(c1, self.c, 1, 1)
self.cv1 = Conv(c1, self.c, 1, 1)
self.cv2 = Conv((2 + n) * self.c, c2, 1)
self.m = nn.ModuleList(Bottleneck(self.c, self.c, shortcut, g, k=((3, 3), (3, 3)), e=1.0) for _ in range(n))
def forward(self, x):
y = [self.cv0(x), self.cv1(x)]
y.extend(m(y[-1]) for m in self.m)
return self.cv2(torch.cat(y, 1))
def transfer_weights(c2f, c2f_v2):
c2f_v2.cv2 = c2f.cv2
c2f_v2.m = c2f.m
state_dict = c2f.state_dict()
state_dict_v2 = c2f_v2.state_dict()
old_weight = state_dict['cv1.conv.weight']
half_channels = old_weight.shape[0] // 2
state_dict_v2['cv0.conv.weight'] = old_weight[:half_channels]
state_dict_v2['cv1.conv.weight'] = old_weight[half_channels:]
for bn_key in ['weight', 'bias', 'running_mean', 'running_var']:
old_bn = state_dict[f'cv1.bn.{bn_key}']
state_dict_v2[f'cv0.bn.{bn_key}'] = old_bn[:half_channels]
state_dict_v2[f'cv1.bn.{bn_key}'] = old_bn[half_channels:]
for key in state_dict:
if not key.startswith('cv1.'):
state_dict_v2[key] = state_dict[key]
for attr_name in dir(c2f):
attr_value = getattr(c2f, attr_name)
if not callable(attr_value) and '_' not in attr_name:
setattr(c2f_v2, attr_name, attr_value)
c2f_v2.load_state_dict(state_dict_v2)
def replace_c2f_with_c2f_v2(module):
for name, child_module in module.named_children():
if isinstance(child_module, C2f):
shortcut = infer_shortcut(child_module.m[0])
c2f_v2 = C2f_v2(child_module.cv1.conv.in_channels, child_module.cv2.conv.out_channels,
n=len(child_module.m), shortcut=shortcut,
g=child_module.m[0].cv2.conv.groups,
e=child_module.c / child_module.cv2.conv.out_channels)
transfer_weights(child_module, c2f_v2)
setattr(module, name, c2f_v2)
else:
replace_c2f_with_c2f_v2(child_module)
def save_model_v2(self: BaseTrainer):
"""
Disabled half precision saving. originated from ultralytics/yolo/engine/trainer.py
"""
ckpt = {
'epoch': self.epoch,
'best_fitness': self.best_fitness,
'model': deepcopy(de_parallel(self.model)),
'ema': deepcopy(self.ema.ema),
'updates': self.ema.updates,
'optimizer': self.optimizer.state_dict(),
'train_args': vars(self.args),
'date': datetime.now().isoformat(),
'version': __version__}
torch.save(ckpt, self.last)
if self.best_fitness == self.fitness:
torch.save(ckpt, self.best)
if (self.epoch > 0) and (self.save_period > 0) and (self.epoch % self.save_period == 0):
torch.save(ckpt, self.wdir / f'epoch{self.epoch}.pt')
del ckpt
def final_eval_v2(self: BaseTrainer):
"""
originated from ultralytics/yolo/engine/trainer.py
"""
for f in self.last, self.best:
if f.exists():
strip_optimizer_v2(f)
if f is self.best:
LOGGER.info(f'\nValidating {f}...')
self.metrics = self.validator(model=f)
self.metrics.pop('fitness', None)
self.run_callbacks('on_fit_epoch_end')
def strip_optimizer_v2(f: Union[str, Path] = 'best.pt', s: str = '') -> None:
"""
Disabled half precision saving. originated from ultralytics/yolo/utils/torch_utils.py
"""
x = torch.load(f, map_location=torch.device('cpu'))
args = {**DEFAULT_CFG_DICT, **x['train_args']}
if x.get('ema'):
x['model'] = x['ema']
for k in 'optimizer', 'ema', 'updates':
x[k] = None
for p in x['model'].parameters():
p.requires_grad = False
x['train_args'] = {k: v for k, v in args.items() if k in DEFAULT_CFG_KEYS}
torch.save(x, s or f)
mb = os.path.getsize(s or f) / 1E6
LOGGER.info(f"Optimizer stripped from {f},{f' saved as {s},' if s else ''} {mb:.1f}MB")
def train_v2(self: YOLO,pruner=None, pruning=False, **kwargs):
"""
Disabled loading new model when pruning flag is set. originated from ultralytics/yolo/engine/model.py
"""
self._check_is_pytorch_model()
if self.session:
if any(kwargs):
LOGGER.warning('WARNING ⚠️ using HUB training arguments, ignoring local training arguments.')
kwargs = self.session.train_args
overrides = self.overrides.copy()
overrides.update(kwargs)
if kwargs.get('cfg'):
LOGGER.info(f"cfg file passed. Overriding default params with {kwargs['cfg']}.")
overrides = yaml_load(check_yaml(kwargs['cfg']))
overrides['mode'] = 'train'
if not overrides.get('data'):
raise AttributeError("Dataset required but missing, i.e. pass 'data=coco128.yaml'")
if overrides.get('resume'):
overrides['resume'] = self.ckpt_path
self.task = overrides.get('task') or self.task
self.trainer = TASK_MAP[self.task][1](overrides=overrides, _callbacks=self.callbacks)
if not pruning:
if not overrides.get('resume'):
self.trainer.model = self.trainer.get_model(weights=self.model if self.ckpt else None, cfg=self.model.yaml)
self.model = self.trainer.model
else:
self.trainer.pruning = True
self.trainer.model = self.model
self.trainer.save_model = save_model_v2.__get__(self.trainer)
self.trainer.final_eval = final_eval_v2.__get__(self.trainer)
self.trainer.hub_session = self.session
if pruner is None:
self.trainer.train()
else:
self.trainer.train(pruner)
if RANK in (-1, 0):
self.model, _ = attempt_load_one_weight(str(self.trainer.best))
self.overrides = self.model.args
self.metrics = getattr(self.trainer.validator, 'metrics', None)
def prune(args):
base_name = 'prune/' + str(datetime.now()) + '/'
model = YOLO(args.model)
model.__setattr__("train_v2", train_v2.__get__(model))
pruning_cfg = yaml_load(check_yaml(args.cfg))
batch_size = pruning_cfg['batch']
is_regularize = args.is_regularize
sparse_train_epoch = args.sparse_train_epoch
finetune_epoch = args.finetune_epoch
pruning_cfg['data'] = "./ultralytics/datasets/soccer-big.yaml"
pruning_cfg['epochs'] = finetune_epoch
model.model.train()
replace_c2f_with_c2f_v2(model.model)
initialize_weights(model.model)
for name, param in model.model.named_parameters():
param.requires_grad = True
example_inputs = torch.randn(1, 3, pruning_cfg["imgsz"], pruning_cfg["imgsz"]).to(model.device)
macs_list, nparams_list, map_list, pruned_map_list = [], [], [], []
base_macs, base_nparams = tp.utils.count_ops_and_params(model.model, example_inputs)
pruning_cfg['name'] = base_name+f"baseline_val"
pruning_cfg['batch'] = 64
validation_model = deepcopy(model)
metric = validation_model.val(**pruning_cfg)
init_map = metric.box.map
macs_list.append(base_macs)
nparams_list.append(100)
map_list.append(init_map)
pruned_map_list.append(init_map)
print(f"Before Pruning: MACs={base_macs / 1e9: .5f} G, #Params={base_nparams / 1e6: .5f} M, mAP={init_map: .5f}")
ch_sparsity = 1 - math.pow((1 - args.target_prune_rate), 1 / args.iterative_steps)
for i in range(args.iterative_steps):
model.model.train()
for name, param in model.model.named_parameters():
param.requires_grad = True
ignored_layers = []
unwrapped_parameters = []
for m in model.model.modules():
if isinstance(m, (Detect,)):
ignored_layers.append(m)
example_inputs = example_inputs.to(model.device)
pruner = tp.pruner.GroupNormPruner(
model.model,
example_inputs,
importance=tp.importance.GroupNormImportance(),
iterative_steps=1,
ch_sparsity=ch_sparsity,
ignored_layers=ignored_layers,
unwrapped_parameters=unwrapped_parameters
)
if is_regularize:
sparse_pruner = tp.pruner.GroupNormPruner(
model.model,
example_inputs,
importance=tp.importance.GroupNormImportance(),
iterative_steps=args.iterative_steps,
ch_sparsity=args.target_prune_rate,
ignored_layers=ignored_layers,
unwrapped_parameters=unwrapped_parameters
)
pruning_cfg['epochs'] = sparse_train_epoch
pruning_cfg['name'] = base_name+"sparse_training"
pruning_cfg['batch'] = batch_size
model.train_v2(sparse_pruner, pruning=True, **pruning_cfg)
pruner.step()
pruning_cfg['epochs'] = finetune_epoch
pruning_cfg['name'] = base_name+f"step_{i}_pre_val"
pruning_cfg['batch'] = 64
validation_model.model = deepcopy(model.model)
metric = validation_model.val(**pruning_cfg)
pruned_map = metric.box.map
if is_regularize:
is_regularize = False
pruned_macs, pruned_nparams = tp.utils.count_ops_and_params(pruner.model, example_inputs.cuda())
else:
pruned_macs, pruned_nparams = tp.utils.count_ops_and_params(pruner.model, example_inputs.to(model.device))
current_speed_up = float(macs_list[0]) / pruned_macs
print(f"After pruning iter {i + 1}: MACs={pruned_macs / 1e9} G, #Params={pruned_nparams / 1e6} M, "
f"mAP={pruned_map}, speed up={current_speed_up}")
for name, param in model.model.named_parameters():
param.requires_grad = True
pruning_cfg['name'] = base_name+f"step_{i}_finetune"
pruning_cfg['batch'] = batch_size
model.train_v2(pruning=True, **pruning_cfg)
pruning_cfg['name'] = base_name+f"step_{i}_post_val"
pruning_cfg['batch'] = 64
validation_model = YOLO(model.trainer.best)
metric = validation_model.val(**pruning_cfg)
current_map = metric.box.map
print(f"After fine tuning mAP={current_map}")
macs_list.append(pruned_macs)
nparams_list.append(pruned_nparams / base_nparams * 100)
pruned_map_list.append(pruned_map)
map_list.append(current_map)
del pruner
model.model.zero_grad()
save_path = 'runs/detect/'+base_name+f"step_{i}_pruned_model.pth"
torch.save(model.model,save_path)
print('pruned model saved in',save_path)
save_pruning_performance_graph(nparams_list, map_list, macs_list, pruned_map_list)
if __name__ == "__main__":
parser = argparse.ArgumentParser()
parser.add_argument('--model', default='runs/detect/train/weights/last.pt', help='Pretrained pruning target model file')
parser.add_argument('--cfg', default='default.yaml',
help='Pruning config file.'
' This file should have same format with ultralytics/yolo/cfg/default.yaml')
parser.add_argument('--is_regularize', default=False, type=bool, help='Sparse training')
parser.add_argument('--max-map-drop', default=1, type=float, help='Allowed maximum map drop after fine-tuning')
parser.add_argument('--target-prune-rate', default=0.2, type=float, help='Target pruning rate')
parser.add_argument('--iterative-steps', default=4, type=int, help='Total pruning iteration step')
parser.add_argument('--sparse_train_epoch', default=10, type=int)
parser.add_argument('--finetune_epoch', default=4, type=int)
args = parser.parse_args()
prune(args)
- 在代码的这些位置加上一些限制,不然它会经常的验证模型:
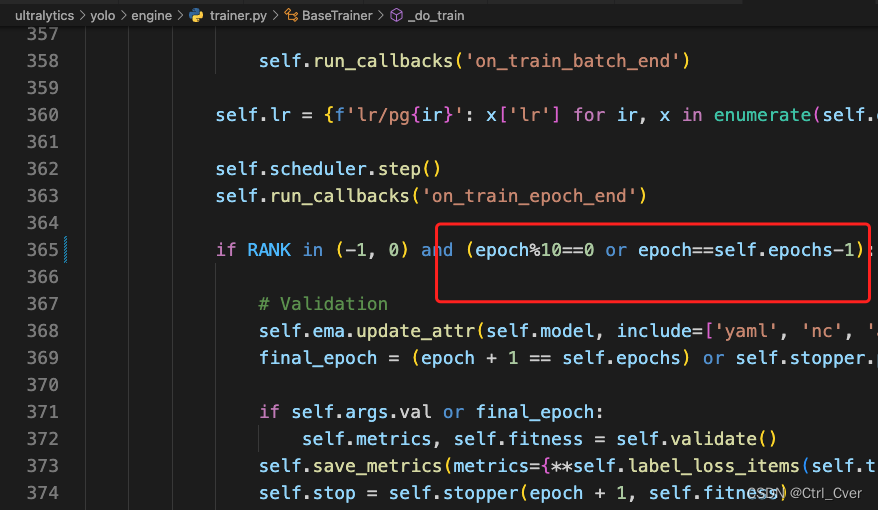
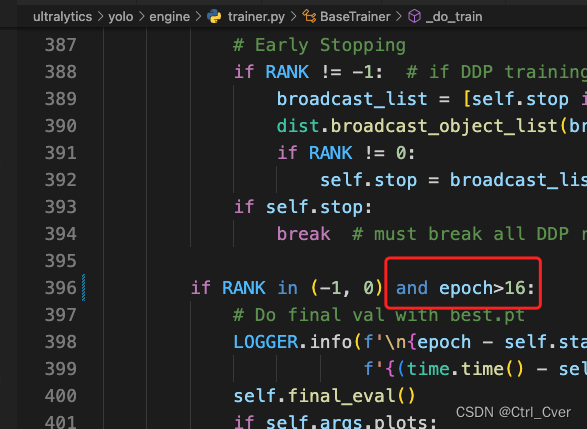
实验结果: 结果如图所示:

额外实验:增加稀疏训练后,再剪枝。
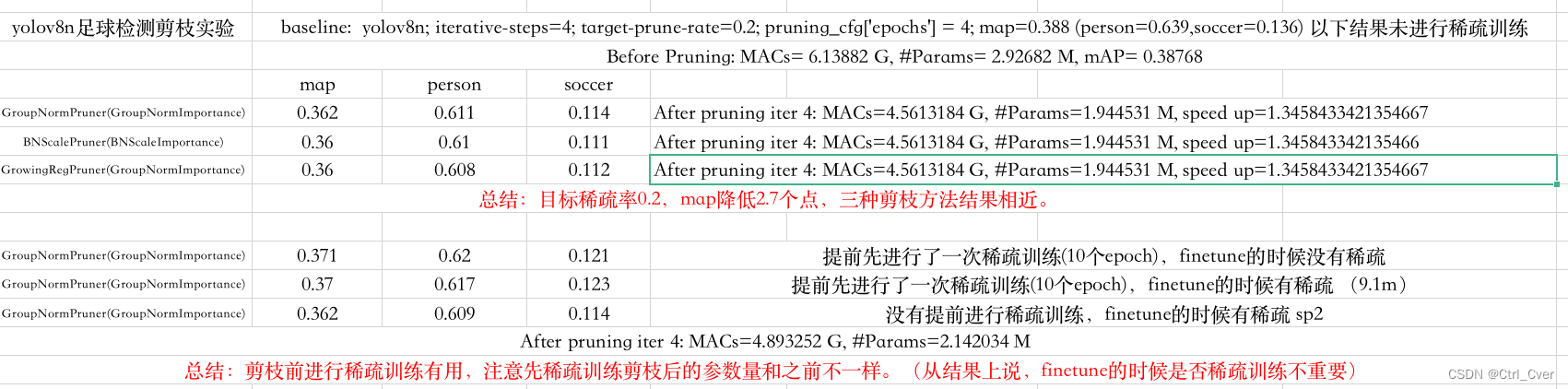