“We have proposed supervised term weighting (STW), a term weighting methodology specifically designed for IR applications involving supervised learning, such as text categorization and text filtering. Supervised term indexing leverages on the training data by weighting a term according to how different its distribution is in the positive and negative training examples. We have also proposed that this should take the form of replacing idf by the category-based term evaluation function that has previously been used in the term selection phase; as such, STW is also efficient, since it reuses for weighting purposes the scores already computed for term selection purposes.”
我们提出了监督词加权(STW),一种专门为涉及监督学习的IR应用设计的词加权方法,例如文本分类和文本过滤。监督术语索引利用训练数据,根据一个术语在正负训练样本中的分布不同而对其进行加权。我们还提出,应该采用以前在词选择阶段使用的基于类别的术语评价功能取代idf的形式;同时,STW也是高效的,因为它重用了词选择阶段已经计算出来的分数用于词加权阶段。
Supervised Term Weighting for Automated Text Categorization——5. Conclusion 总结
最新推荐文章于 2024-09-20 23:31:36 发布
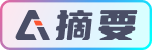