- 文章转自微信公众号:机器学习炼丹术
- 作者:陈亦新(欢迎交流共同进步)
- 联系方式:微信cyx645016617
- 学习论文:Analyzing and Improving the Image Quality of StyleGAN
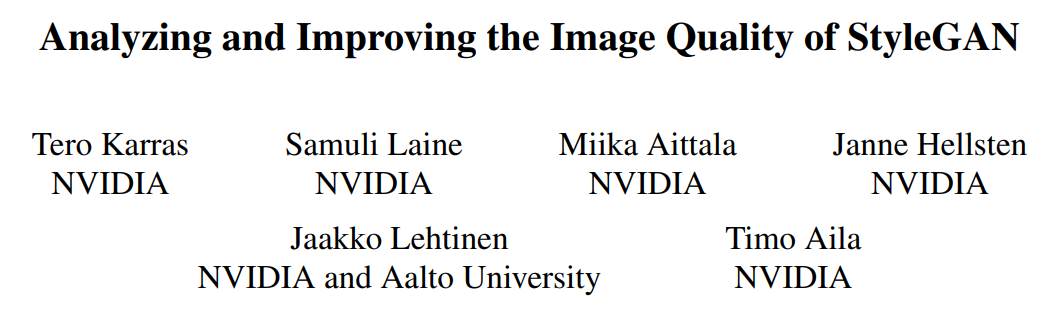
3.1 AdaIN
StyleGAN第一个版本提出了通过AdaIN模块来实现生成,这个模块非常好也非常妙。
图片中的latent Code W是一个一维向量。然后Adaptive Instance Norm其实是基于Instance Norm修改的。Instance Norm当中,包含了2个可学习参数,shift和scale。而AdaIN就是让这两个可学习参数是从W向量经过全连接层直接计算出来的。因为shift scale会影响生成的图片,所以这样可以让生成的图片收到latent code W的控制,从而实现生成的可控。
3.2 AdaIN的问题
研究人员发现,StyleGAN生成的图片中,大概率存在一些水滴样子的补丁。
研究人员说:We pinpoint the problem to the AdaIN operation that normalizes the mean and variance of each feature map separately, thereby potentially destroying any information found in the magnitudes of the features relative to each other.
导致水珠的原因是AdaIN操作,AdaIN对每一个feature map的通道进行归一化,这样可能破坏掉feature之间的信息。当然实验证明发现,去除AdaIN的归一化操作后,水珠就消失了。
我们来看StyleGAN2是如何改进AdaIN模块的:
- 图a是原始的styleGAN1的结构图;
- 图b把AdaIN拆分成了Norm mean/std和Mod mean/std两部分,Norm是做的归一化操作,而Mod则是从latent code计算shift和scale参数的步骤;
- 图c,现在我们修改一下模型,我们去除对于mean的norm和mod的操作,只留下对方差的操作。
- 图d则是在c的基础上,进一步提出了weight demodulation的操作。
3.3 weight demodulation
虽然我们修改了网络结构,去除了水滴问题,但是styleGAN的目的是对特征实现可控的精细的融合。
StyleGAN2说,style modulation可能会放大某些特征的影像,所以style mixing的话,我们必须明确的消除这种影像,否则后续层的特征无法有效的控制图像。如果他们想要牺牲scale-specific的控制能力,他们可以简单的移除normalization,就可以去除掉水滴伪影,这还可以使得FID有着微弱的提高。现在他们提出了一个更好的替代品,移除伪影的同时,保留完全的可控性。这个就是weight demodulation。
我们继续看这个图c:
里面包含三个style block,每一个block包含modulation(Mod),convolution and normalization。
modulation可以影响着卷积层的输入特征图。所以,其实Mod和卷积是可以继续宁融合的。比方说,input先被Mod放大了3倍,然后在进行卷积,这个等价于input直接被放大了3倍的卷积核进行卷积。Modulation和卷积都是在通道维度进行操作。所以有如下公式:
W i j k ′ = s i ⋅ w i j k W'_{ijk}=s_i \cdot w_{ijk} Wijk′=si⋅wijk
接下来的norm部分也做了修改:
w i j k ′ ′ = w i j k ′ ∑ i , k w ′ i j k 2 + ϵ w''_{ijk}=\frac{w'_{ijk}}{\sqrt{\sum_{i,k}{{w'}_{ijk}^2+\epsilon}}} wijk′′=∑i,kw′ijk2+ϵwijk′
这里替换了对特征图做归一化,而是去卷积的参数做了一个归一化,先前有研究提出,这样会有助于GAN的训练。
至此,我们发现,Mod和norm部分的操作,其实都可以融合到卷积核上。
3.4 代码学习
class GeneratorBlock(nn.Module):
def __init__(self, latent_dim, input_channels, filters, upsample = True, upsample_rgb = True, rgba = False):
super().__init__()
self.upsample = nn.Upsample(scale_factor=2, mode='bilinear', align_corners=False) if upsample else None
self.to_style1 = nn.Linear(latent_dim, input_channels)
self.to_noise1 = nn.Linear(1, filters)
self.conv1 = Conv2DMod(input_channels, filters, 3)
self.to_style2 = nn.Linear(latent_dim, filters)
self.to_noise2 = nn.Linear(1, filters)
self.conv2 = Conv2DMod(filters, filters, 3)
self.activation = leaky_relu()
self.to_rgb = RGBBlock(latent_dim, filters, upsample_rgb, rgba)
def forward(self, x, prev_rgb, istyle, inoise):
if exists(self.upsample):
x = self.upsample(x)
inoise = inoise[:, :x.shape[2], :x.shape[3], :]
noise1 = self.to_noise1(inoise).permute((0, 3, 2, 1))
noise2 = self.to_noise2(inoise).permute((0, 3, 2, 1))
style1 = self.to_style1(istyle)
x = self.conv1(x, style1)
x = self.activation(x + noise1)
style2 = self.to_style2(istyle)
x = self.conv2(x, style2)
x = self.activation(x + noise2)
rgb = self.to_rgb(x, prev_rgb, istyle)
return x, rgb
可以发现,这个噪音也会经过Linear层的简单变换,然后里面加入了残差。为什么要输出rgb图像呢?这个会放在下次,或者下下次的内容。styleGAN1是需要用progressive growing的策略的,而StyleGAN2使用新的架构,解决了这种繁琐的训练方式。下次讲styleGAN2的lazy path length regularization,下下次讲这个No progressive growing。
回到代码部分,发现我们讲到的AdaIN的改进,应该在Conv2DMod模块当中:
class Conv2DMod(nn.Module):
def __init__(self, in_chan, out_chan, kernel, demod=True, stride=1, dilation=1, eps = 1e-8, **kwargs):
super().__init__()
self.filters = out_chan
self.demod = demod
self.kernel = kernel
self.stride = stride
self.dilation = dilation
self.weight = nn.Parameter(torch.randn((out_chan, in_chan, kernel, kernel)))
self.eps = eps
nn.init.kaiming_normal_(self.weight, a=0, mode='fan_in', nonlinearity='leaky_relu')
def _get_same_padding(self, size, kernel, dilation, stride):
return ((size - 1) * (stride - 1) + dilation * (kernel - 1)) // 2
def forward(self, x, y):
b, c, h, w = x.shape
w1 = y[:, None, :, None, None]
w2 = self.weight[None, :, :, :, :]
weights = w2 * (w1 + 1)
if self.demod:
d = torch.rsqrt((weights ** 2).sum(dim=(2, 3, 4), keepdim=True) + self.eps)
weights = weights * d
x = x.reshape(1, -1, h, w)
_, _, *ws = weights.shape
weights = weights.reshape(b * self.filters, *ws)
padding = self._get_same_padding(h, self.kernel, self.dilation, self.stride)
x = F.conv2d(x, weights, padding=padding, groups=b)
x = x.reshape(-1, self.filters, h, w)
return x
代码剖析:
y是style code经过全连接层得到的scale参数,假设batch size是16,输入特征图的通道数为256。所以w1.shape=[16,1,256,1,1];
w2是卷积层的weight,w2.shape=[1,out_chan, in_chan, kernel, kernel]
这里为什么要为w1加1呢?说实话,我觉得加不加都无所谓,因为之前的全连接层也有bias,所以无所谓的。
torch.rsqrt就是取平方根后取倒数。weight先求平方,然后对234维度求和,那么就保留了batch维度和输出通道维度。这个运算过程和论文中的weight demodulation是一致的