前言
最近正在复习数据结构,正好复习到了哈夫曼树这个地方,想起之前学习word2Vec时碰到了哈夫曼树,网上的资料也比较少,很多博文只是摆了一堆的晦涩难啃的数学公式(菜鸡不配),当时并没有好好去深究背后的逻辑,所以现在正好对之前学习的知识进行梳理,希望能让自己的理解更上一层楼~~~~~
废话少说,开整
1.对于word2Vec随便google都有几万篇博文,原理这里就不再赘述(CBOW,Skip-gram,负采样,重采样,层次softmax)
2.关于哈夫曼树的原理之前有写过基于java构建的哈夫曼树,这里也不再赘述
3.为什么word2Vec里面需要使用哈夫曼树(层次softmax)呢?
先回顾下传统的神经网络词向量语言模型,里面一般有三层,输入层(词向量),隐藏层和输出层(softmax层)。里面最大的问题在于从隐藏层到输出的softmax层的计算量很大,因为要计算所有词的softmax概率,再去找概率最大的值。这个模型如下图所示。其中V是词汇表的大小,
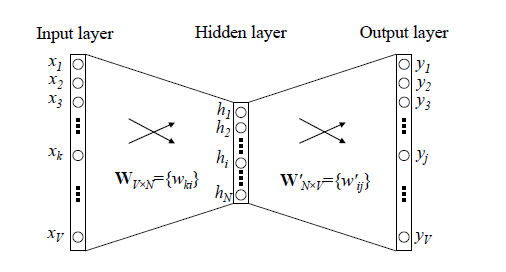
word2vec对这个模型做了改进,首先,对于从输入层到隐藏层的映射,没有采取神经网络的线性变换加激活函数的方法,而是采用简单的对所有输入词向量求和并取平均的方法。比如输入的是三个4维词向量:(1,2,3,4),(9,6,11,8),(5,10,7,12),那么我们word2vec映射后的词向量就是(5,6,7,8)。由于这里是从多个词向量变成了一个词向量。
第二个改进就是从隐藏层到输出的softmax层这里的计算量个改进。**为了避免要计算所有词的softmax概率,word2vec采样了霍夫曼树来代替从隐藏层到输出softmax层的映射。**我们在上一节已经介绍了霍夫曼树的原理。如何映射呢?这里就是理解word2vec的关键所在了。
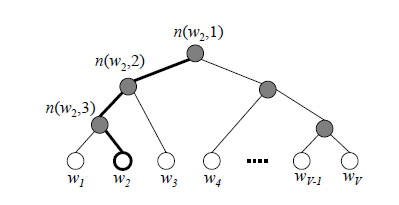
和之前的神经网络语言模型相比,我们的霍夫曼树的所有内部节点就类似之前神经网络隐藏层的神经元,其中,根节点的词向量对应我们的投影后的词向量,而所有叶子节点就类似于之前神经网络softmax输出层的神经元,叶子节点的个数就是词汇表的大小。在霍夫曼树中,隐藏层到输出层的softmax映射不是一下子完成的,而是沿着霍夫曼树一步步完成的,因此这种softmax取名为"Hierarchical Softmax"。
在word2vec中,我们采用了二元逻辑回归的方法,即规定沿着左子树走,那么就是负类(霍夫曼树编码1),沿着右子树走,那么就是正类(霍夫曼树编码0)。判别正类和负类的方法是使用sigmoid函数
使用霍夫曼树有什么好处呢?首先,由于是二叉树,之前计算量为V,现在变成了log2V。第二,由于使用霍夫曼树是高频的词靠近树根,这样高频词需要更少的时间会被找到,这符合我们的贪心优化思想。
容易理解,被划分为左子树而成为负类的概率为P(−)=1−P(+)。在某一个内部节点,要判断是沿左子树还是右子树走的标准就是看P(−),P(+)谁的概率值大。而控制P(−),P(+)谁的概率值大的因素一个是当前节点的词向量,另一个是当前节点的模型参数θ。
对于上图中的w2,如果它是一个训练样本的输出,那么我们期望对于里面的隐藏节点n(w2,1)的P(−)概率大,n(w2,2)的P(−)概率大,n(w2,3)的P(+)概率大。
回到基于Hierarchical Softmax的word2vec本身,我们的目标就是找到合适的所有节点的词向量和所有内部节点θ, 使训练样本达到最大似然。那么如何达到最大似然呢?
基于Hierarchical Softmax的模型梯度计算
我们使用最大似然法来寻找所有节点的词向量和所有内部节点θ。先拿上面的w2例子来看,我们期望最大化下面的似然函数:
∏i=13P(n(wi),i)=(1−11+e−xTwθ1)(1−11+e−xTwθ2)11+e−xTwθ3
对于所有的训练样本,我们期望最大化所有样本的似然函数乘积。
如果对于推导感兴趣可以参考https://www.cnblogs.com/pinard/p/7243513.html
下面用Python创建huffman树
1.创建结点
class HuffmanNode:
def __init__(self,word_id,frequency):
#单词的id
self.word_id = word_id
#词频
self.frequency = frequency
#左孩子
self.left_child = None
#右孩子
self.right_child = None
#父子
self.father = None
#huffman_code
self.Huffman_code = []
#路径
self.path = []
2.实例化
wordid_frequency_dict = {0:4,1:6,2:3,3:2,4:2}
#设置的是词频的对应的word的id
wordid_code = dict()
wordid_path = dict()
unmerged_node_list = [HuffmanNode(wordid,frequency) for wordid,frequency in wordid_frequency_dict.items()]
huffman = [HuffmanNode(wordid,frequency) for wordid,frequency in wordid_frequency_dict.items()]
3.形成结点方法(第4步会用到这个方法)
def merge_node(node1,node2):
sum_frequency = node1.frequency + node2.frequency
mid_node_id = len(huffman)
father_node = HuffmanNode(mid_node_id,sum_frequency)
if node1.frequency >= node2.frequency:
father_node.left_child = node1
father_node.right_child = node2
else:
father_node.left_child = node2
father_node.right_child = node1
huffman.append(father_node)
return father_node
如果你熟悉哈夫曼树编码原理,上面的代码看起来会非常easy
4.建树
def build_tree(node_list):
while len(node_list) > 1:
i1 = 0
i2 = 1
if node_list[i2].frequency < node_list[i1].frequency:
[i1,i2] = [i2,i1]
for i in range(2,len(node_list)):
#这里需要判断的是词频出现最小的两项
if node_list[i].frequency < node_list[i2].frequency:
i2 = i
if node_list[i2].frequency < node_list[i1].frequency:
[i1,i2] = [i2,i1]
#这里只是最后选择i1 i2作为现在的最小的节点的值
father_node = merge_node(node_list[i1],node_list[i2]) #这里是合并最小的两个节点
if i1 < i2:
node_list.pop(i2)
node_list.pop(i1)
#这里一定要注意先将大的index对应的node出列表
elif i1 > i2:
node_list.pop(i1)
node_list.pop(i2)
else:
raise RuntimeError('i1 should not be equal to i2')
node_list.insert(0,father_node) #插入新的节点
root = node_list[0]
return root
root = build_tree(unmerge_node_list)
5.形成哈夫曼编码
def generate_huffman_code_and_path():
stack = [root]
while len(stack) > 0:
node = stack.pop()
# 顺着左子树走
while node.left_child or node.right_child:
code = node.Huffman_code
path = node.path
node.left_child.Huffman_code = code + [1]
node.right_child.Huffman_code = code + [0]
node.left_child.path = path + [node.word_id]
node.right_child.path = path + [node.word_id]
# 把没走过的右子树加入栈
stack.append(node.right_child)
node = node.left_child
word_id = node.word_id
word_code = node.Huffman_code
word_path = node.path
huffman[word_id].Huffman_code = word_code
huffman[word_id].path = word_path
# 把节点计算得到的霍夫曼码、路径 写入词典的数值中
wordid_code[word_id] = word_code
wordid_path[word_id] = word_path
return wordid_code, wordid_path

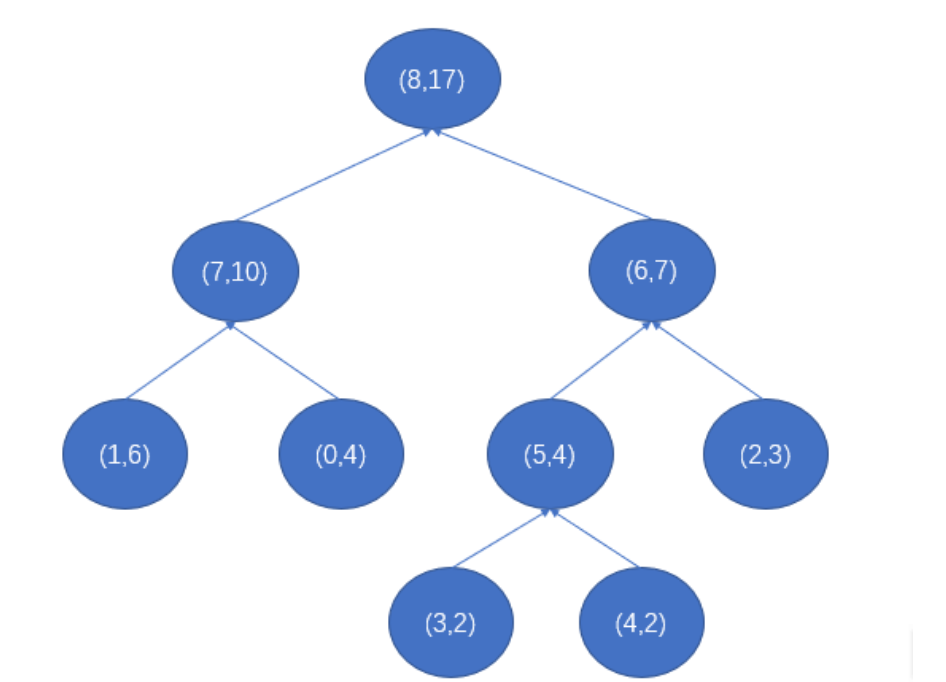
结果:
6.得到正样本和负样本
def get_all_pos_and_neg_path():
positive = [] # 所有词的正向路径数组
negative = [] # 所有词的负向路径数组
for word_id in range(len(wordid_frequency_dict)):
pos_id = [] # 存放一个词 路径中的正向节点id
neg_id = [] # 存放一个词 路径中的负向节点id
for i, code in enumerate(huffman[word_id].Huffman_code):
if code == 1:
pos_id.append(huffman[word_id].path[i])
else:
neg_id.append(huffman[word_id].path[i])
positive.append(pos_id)
negative.append(neg_id)
return positive, negative
结果:
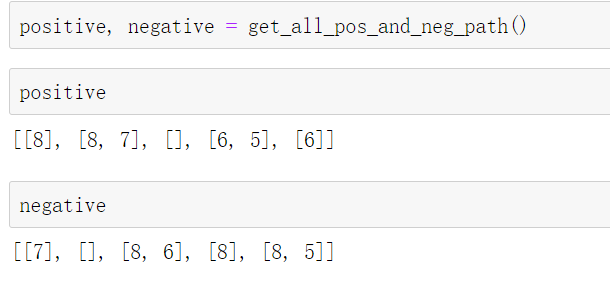
参考博文:
https://github.com/wmathor/nlp-tutorial/blob/master/1-2.Word2Vec/Word2Vec-Torch(Softmax).py
pytorch学习 中 torch.squeeze() 和torch.unsqueeze()的用法_nancheng911的博客-CSDN博客_torch.unsqueeze
word2vec原理(二) 基于Hierarchical Softmax的模型 - 刘建平Pinard - 博客园