1、数据获取
from sklearn.datasets import load_iris
import pandas as pd
x_data = load_iris().data
y_data = load_iris().target
x_data = pd.DataFrame(x_data, columns=['花萼长度','花萼宽度','花瓣长度','花瓣宽度'])
pd.set_option('display.unicode.east_asian_width', True)
x_data['类别'] = y_data
x_data
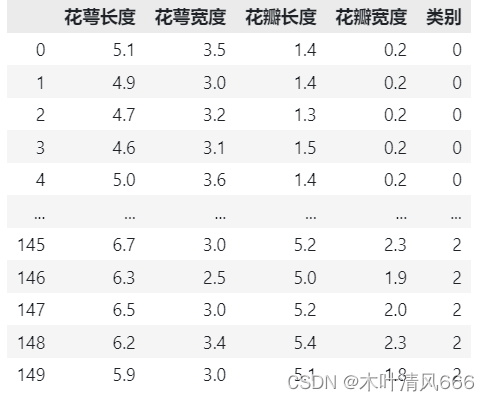
2、数据集构建
import tensorflow as tf
import numpy as np
from sklearn.datasets import load_iris
x_data = load_iris().data
y_data = load_iris().target
np.random.seed(1)
np.random.shuffle(x_data)
np.random.seed(1)
np.random.shuffle(y_data)
tf.random.set_seed(1)
x_train, x_test = x_data[:-30], x_data[-30:]
y_train, y_test = y_data[:-30], y_data[-30:]
train_db = tf.data.Dataset.from_tensor_slices((x_train, y_train)).batch(32)
test_db = tf.data.Dataset.from_tensor_slices((x_test, y_test)).batch(32)
3、模型的训练验证
lr = 0.1
loss_all = 0
Epoch = 500
train_loss_list = []
test_acc = []
w = tf.Variable(tf.random.truncated_normal([4,3], stddev=0.1, seed=1))
b = tf.Variable(tf.random.truncated_normal([3], stddev=0.1, seed=1))
for epoch in range(Epoch):
for step, (x_, y_) in enumerate(train_db):
with tf.GradientTape() as tape:
x_ = tf.cast(x_, tf.float32)
y_pre = tf.matmul(x_, w) + b
y_pre = tf.nn.softmax(y_pre)
y_lab = tf.one_hot(y_, depth=3)
loss = tf.reduce_mean(tf.square(y_lab - y_pre))
loss_all += loss.numpy()
grads = tape.gradient(loss, [w,b])
w.assign_sub(lr * grads[0])
b.assign_sub(lr * grads[1])
print(f'Epoch: {epoch}, loss: {loss_all/4}')
train_loss_list.append(loss_all/4)
loss_all = 0
total_correct, total_number = 0, 0
for x_,y_ in test_db:
x_ = tf.cast(x_, tf.float32)
y_pre = tf.matmul(x_, w) + b
y_pre = tf.nn.softmax(y_pre)
y_p = tf.argmax(y_pre, axis=1)
y_p = tf.cast(y_p, dtype=y_.dtype)
correct = tf.cast(tf.equal(y_p, y_), dtype=tf.int32)
correct = tf.reduce_sum(correct)
total_correct += int(correct)
total_number += x_.shape[0]
acc = total_correct / total_number
test_acc.append(acc)
print("Test_acc:", acc)
print("-"*30)
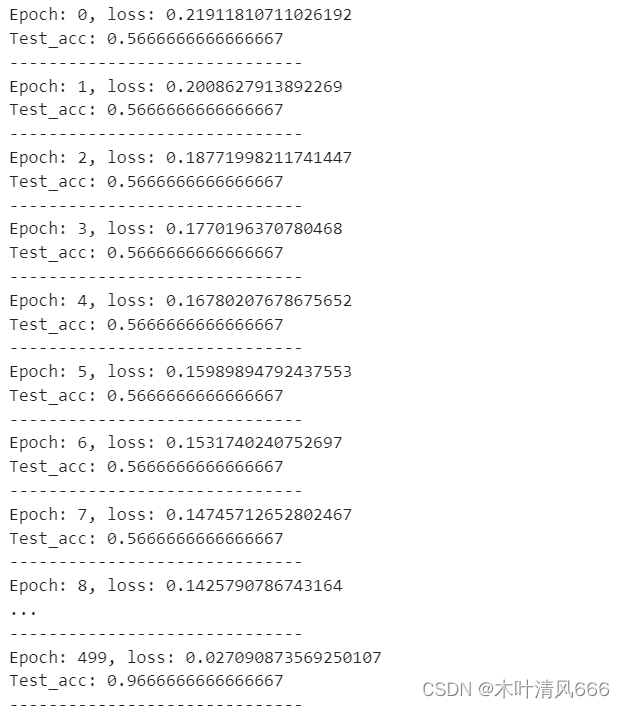
4、可视化训练过程
import matplotlib.pyplot as plt
fig, ax = plt.subplots()
ax.plot(train_loss_list,'b-')
ax.set_xlabel('Epoch')
ax.set_ylabel('Loss')
ax1 = ax.twinx()
ax1.plot(test_acc,'r-')
ax1.set_ylabel('Acc')
ax1.spines['left'].set_color('blue')
ax1.spines['right'].set_color('red')
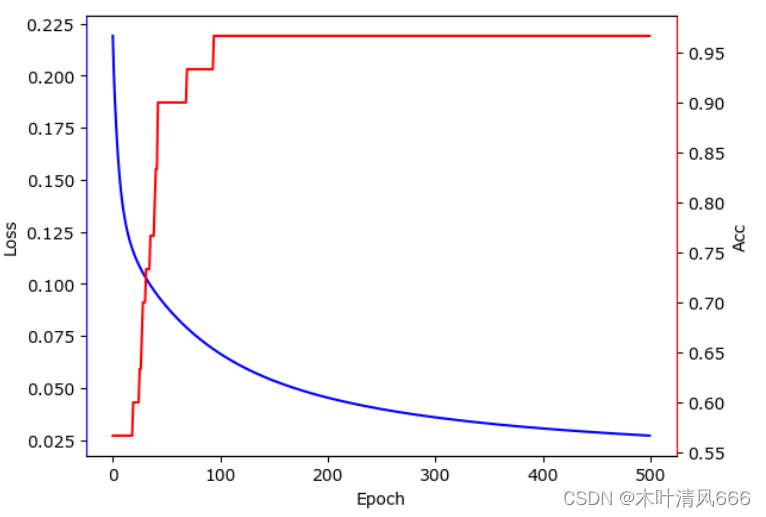