V
G
G
−
M
o
d
e
l
(
p
y
t
o
r
c
h
版
本
)
VGG-Model(pytorch版本)
VGG−Model(pytorch版本)
import torch
import torch.nn as nn
import torchvision
def Conv3x3BNReLU(in_channels,out_channels):
return nn.Sequential(
nn.Conv2d(in_channels=in_channels,out_channels=out_channels,kernel_size=3,stride=1,padding=1),
nn.BatchNorm2d(out_channels),
nn.ReLU6(inplace=True)
)
class VGGNet(nn.Module):
def __init__(self, block_nums,num_classes=5,num_linear=25088):
super(VGGNet, self).__init__()
self.stage1 = self._make_layers(in_channels=3, out_channels=64, block_num=block_nums[0])
self.stage2 = self._make_layers(in_channels=64, out_channels=128, block_num=block_nums[1])
self.stage3 = self._make_layers(in_channels=128, out_channels=256, block_num=block_nums[2])
self.stage4 = self._make_layers(in_channels=256, out_channels=512, block_num=block_nums[3])
self.stage5 = self._make_layers(in_channels=512, out_channels=512, block_num=block_nums[4])
self.classifier = nn.Sequential(
nn.Linear(in_features=num_linear,out_features=4096),
nn.ReLU6(inplace=True),
nn.Dropout(p=0.2),
nn.Linear(in_features=4096, out_features=4096),
nn.ReLU6(inplace=True),
nn.Dropout(p=0.2),
nn.Linear(in_features=4096, out_features=num_classes)
)
def _make_layers(self, in_channels, out_channels, block_num):
layers = []
layers.append(Conv3x3BNReLU(in_channels,out_channels))
for i in range(1,block_num):
layers.append(Conv3x3BNReLU(out_channels,out_channels))
layers.append(nn.MaxPool2d(kernel_size=2,stride=2))
return nn.Sequential(*layers)
def forward(self, x):
x = self.stage1(x)
x = self.stage2(x)
x = self.stage3(x)
x = self.stage4(x)
x = self.stage5(x)
x = x.view(x.size(0),-1)
out = self.classifier(x)
return out
def VGG16(num_classes,num_linear):
block_nums = [2, 2, 3, 3, 3]
model = VGGNet(block_nums,num_classes,num_linear)
return model
def VGG19(num_classes,num_linear):
block_nums = [2, 2, 4, 4, 4]
model = VGGNet(block_nums,num_classes,num_linear)
return model
rgb = torch.randn(1, 3, 224, 224)
net = VGG19(num_classes=8,num_linear=25088)
out = net(rgb)
print('--VGG19---'*5)
print(out.shape)
print('----------'*5)
net = VGG16(num_classes=8,num_linear=25088)
out = net(rgb)
print('--VGG16---'*5)
print(out.shape)
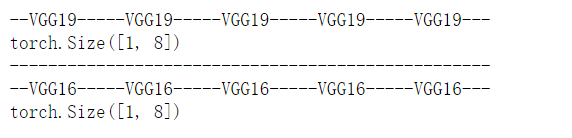
补充:SE_VGG
SE_VGG