1.Inception-ResNet-V2结构:
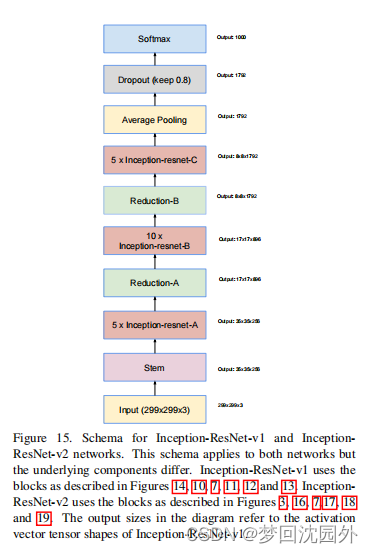
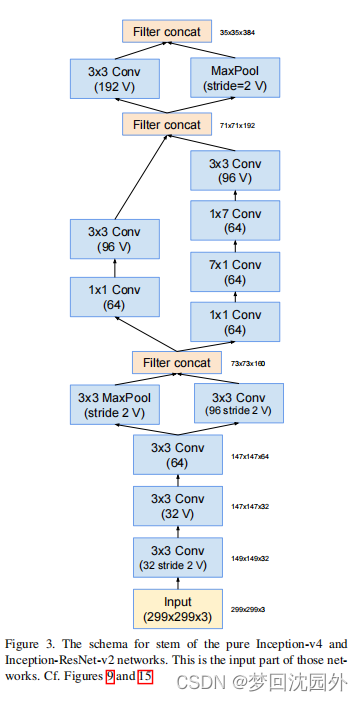
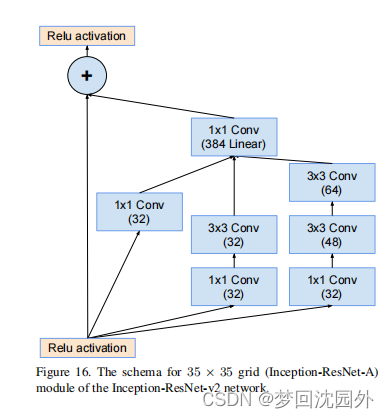
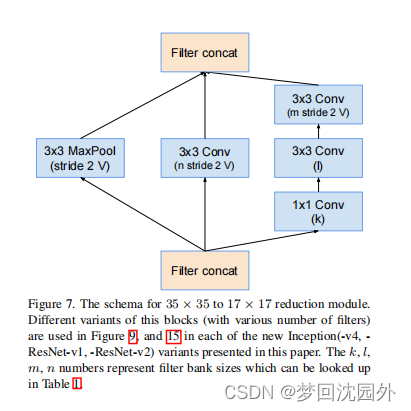
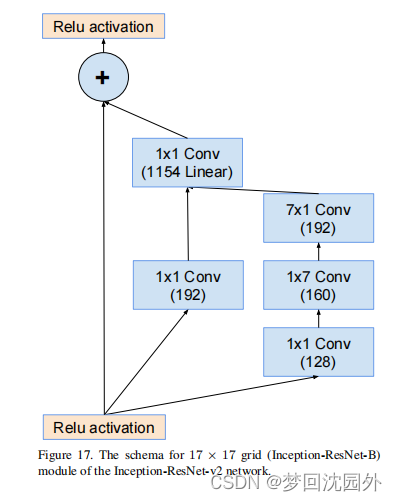
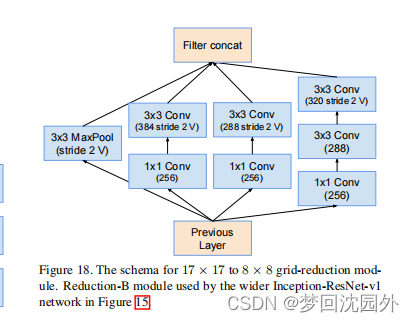
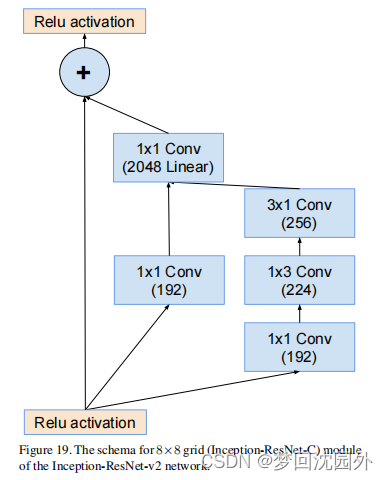
2.代码实现
# 构建卷积块
def conv_fn(x,filters,kernel_size,strides,padding="same",activation="relu"):
x = keras.layers.Conv2D(filters,kernel_size=kernel_size,strides=strides,padding=padding)(x)
x = keras.layers.BatchNormalization()(x)
if activation is not None:
x = keras.activations.get(activation)(x)
return x
# 构建inception_resnet模块
k = keras.backend
def inception_resnet(x,scale,block_name,activation="relu"):
if block_name == 'Inception_Resnet_A':
# 路径1 1*1 32
branch1 = conv_fn(x,32,1,1)
# 路径2 1*1 3*3
branch2 = conv_fn(x,32,1,1)
branch2 = conv_fn(x,32,32,1)
# 路径3 1*1—3*3
branch3 = conv_fn(x,32,1,1)
branch3 = conv_fn(x,48,3,1)
branch3 = conv_fn(x,64,32,1)
branch = keras.layers.Concatenate(axis=3)([branch1,branch2,branch3])
mixed = conv_fn(branch,384,1,1)
elif block_name == 'Inception_Resnet_B':
# 路径1 1*1 192
branch1 = conv_fn(x,192,1,1)
# 路径2 1*1-1*7-7*1
branch2 = conv_fn(x,128,1,1)
branch2 = conv_fn(x,160,(1,7),1)
branch2 = conv_fn(x,192,(7,1),1)
branch = keras.layers.Concatenate(axis=3)([branch1,branch2])
mixed = conv_fn(branch,1154,1,1)
elif block_name == 'Inception_Resnet_C':
# 路径1 1*1 192
branch1 = conv_fn(x,192,1,1)
# 路径2 1*1-1*3-3*1
branch2 = conv_fn(x,192,1,1)
branch2 = conv_fn(x,224,(1,3),1)
branch2 = conv_fn(x,224,(3,192),1)
branch = keras.layers.Concatenate(axis=3)([branch1,branch2])
mixed = mixed = conv_fn(branch,2048,1,1)
up = conv_fn(x,k.int_shape(mixed)[3],1,1,activation=None)
x = keras.layers.Lambda(lambda inputs:inputs[0]+scale*inputs[1])([up,mixed])
if activation is not None:
x = keras.activations.get(activation)(x)
return x
# 构建Inception_Resnet_v2
def inception_resnet_v2(input_shape,n_classes=1000):
##stem
x_input = keras.layers.Input(shape=input_shape)
# 149*149*3
x = conv_fn(x_input,32,3,2,padding="valid")
# 147*147*32
x = conv_fn(x,32,3,1,padding="valid")
# 147*147*64
x = conv_fn(x,64,3,1)
# 73*73*160
branch1 = keras.layers.MaxPooling2D(pool_size=3,strides=2,padding="valid")(x)
branch2 = conv_fn(x,32,3,2,padding="valid")
x = keras.layers.Concatenate(axis=3)([branch1,branch2])
# 71*71*192
# 路径1 1*1-3*3 1v
branch1 = conv_fn(x,64,3,1)
branch1 = conv_fn(branch1,96,3,1,padding="valid")
#路径2 1*1-1*7-7*1-3*3 1v
branch2 = conv_fn(x,64,3,1)
branch2 = conv_fn(branch2,64,(1,7),1)
branch2 = conv_fn(branch2,64,(7,1),1)
branch2 = conv_fn(branch2,96,3,1,padding="valid")
x = keras.layers.Concatenate(axis=3)([branch1,branch2])
# 35*35*384
branch1 = keras.layers.MaxPooling2D(pool_size=3,strides=2,padding="valid")(x)
branch2 = conv_fn(x,192,3,2,padding="valid")
x = keras.layers.Concatenate(axis=3)([branch1,branch2])
## 5*inception_resnet_A
# 35*35*384
for i in range(5):
x = inception_resnet(x,0.2,'Inception_Resnet_A')
## reduction_A
#17*17*1152
branch1 = keras.layers.MaxPooling2D(pool_size=3,strides=2,padding="valid")(x)
branch2 = conv_fn(x,384,3,2,padding="valid")
branch3 = conv_fn(x,256,1,1)
branch3 = conv_fn(branch3,256,3,1)
branch3 = conv_fn(branch3,384,3,2,padding="valid")
x = keras.layers.Concatenate(axis=3)([branch1,branch2,branch3])
## 10*inception_resnet_B
# 17*17*1154
for i in range(10):
x = inception_resnet(x,0.2,'Inception_Resnet_B')
## reduction_B
# 8*8*2146
branch1 = keras.layers.MaxPooling2D(pool_size=3,strides=2,padding="valid")(x)
branch2 = conv_fn(x,256,1,1)
branch2 = conv_fn(branch2,384,3,2,padding="valid")
branch3 = conv_fn(x,256,1,1)
branch3 = conv_fn(branch3,288,3,2,padding="valid")
branch4 = conv_fn(x,256,1,1)
branch4 = conv_fn(branch4,288,3,1)
branch4 = conv_fn(branch4,320,3,2,padding="valid")
x = keras.layers.Concatenate(axis=3)([branch1,branch2,branch3,branch4])
## 5*inception_resnet_C
# 8*8*2048
for i in range(5):
x = inception_resnet(x,0.2,'Inception_Resnet_C')
x = keras.layers.GlobalAveragePooling2D()(x)
x = keras.layers.Dropout(0.8)(x)
outputs = keras.layers.Dense(n_classes,activation="softmax")(x)
model = keras.models.Model(inputs=[x_input],outputs=[outputs])
return model
3.训练模型(使用指数调度)
model = inception_resnet_v2([299,299,3],12)
n_epochs = 100
s = 20*len(train_images)//16
learning_rate = keras.optimizers.schedules.ExponentialDecay(0.01,s,0.1)
optimizer = keras.optimizers.SGD(learning_rate,momentum=0.9,nesterov=True)
model.compile(loss="categorical_crossentropy",optimizer=optimizer,metrics=["accuracy"])
history = model.fit(train_images,y_train,epochs=n_epochs,validation_split=0.2,batch_size=16)