基本介绍
基础信息
- 本文会用到博主写的一些线上脚本,如果搭建者时纯内网的话,可能需要手动压缩镜像,然后解压镜像,才能正常部署
- 本文从GPU服务器获取、到 nvidia-docker的安装、再到Xinference 部署私有化三个模型,最后搭建dify(供从 Agent 构建到 AI workflow 编排、RAG 检索、模型管理等能力,轻松构建和运营生成式 AI 原生应用)、最后再配置实例dify,配置dify实例,整合三个模型,最后实现知识库搭建
GPU服务器获取
基础安装
基础信息安装
- 拿到服务器后,进行基础的命令初始化、docker安装等,这里博主提供脚本给大家
- 基础安装
wget -O centos7-init.sh https://files.rundreams.net/sh/run-sh/init/centos7-init.sh && sh centos7-init.sh
wget -O docker-install.sh https://files.rundreams.net/sh/run-sh/docker/docker-install.sh && sh docker-install.sh
- 由于Xinference的docker镜像包比较大,所以建议大家在docker安装成功后,就进行进行包拉取,差不多在18G左右
docker pull registry.cn-hangzhou.aliyuncs.com/xprobe_xinference/xinference
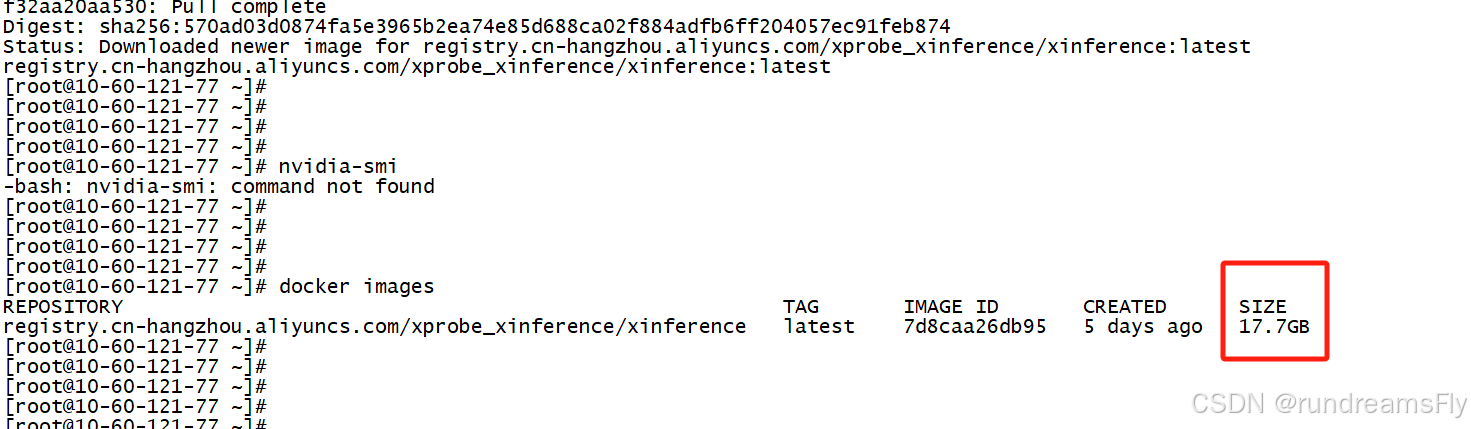
显卡驱动配置
显卡检查
下载
wget https://cn.download.nvidia.cn/tesla/550.90.07/NVIDIA-Linux-x86_64-550.90.07.run
禁用nouveau系统自带驱动
- 安装驱动前,需要禁用nouveau系统自带驱动
- 查看系统自带的驱动,如果有结果,则说明存在nouveau,没有则直接跳过这一步
lsmod | grep nouveau
vi /usr/lib/modprobe.d/dist-blacklist.conf
blacklist