仅为个人笔记使用
Representations
good representation might differ from task to task.
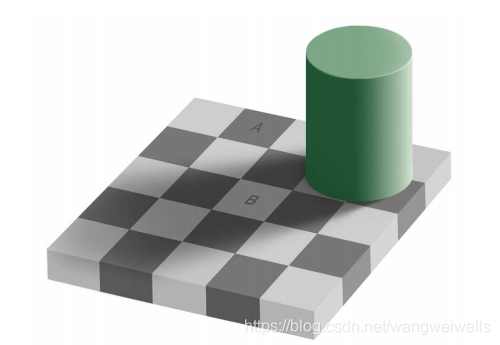
There is a video link
pattern recognition system
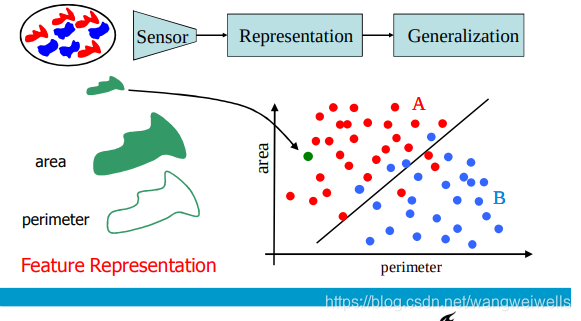
Dissimilarity Representation
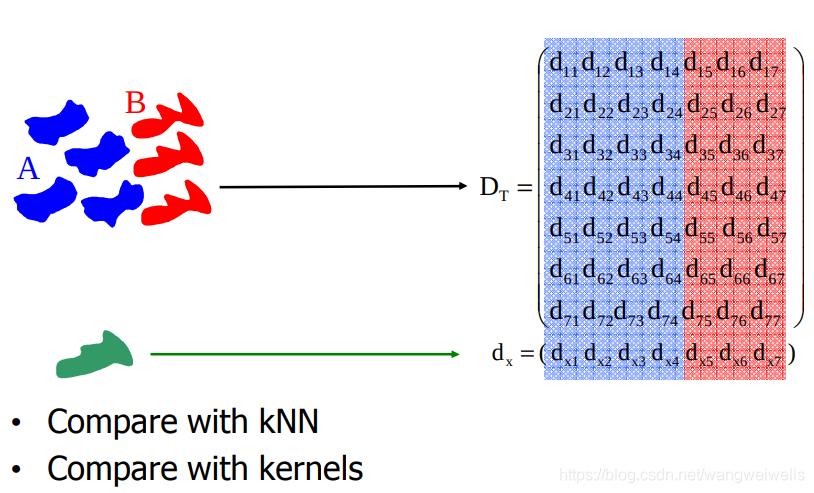
k
(
x
,
z
)
=
<
ϕ
(
x
)
,
ϕ
(
z
)
>
k(x,z) =<\phi(x),\phi(z)>
k(x,z)=<ϕ(x),ϕ(z)>
we can transfer euclidean distance through mapping inplicitly.
d
(
x
,
z
)
=
∣
∣
ϕ
(
x
)
−
ϕ
(
z
)
∣
∣
2
d(x,z)= || \phi(x)-\phi(z)||^2
d(x,z)=∣∣ϕ(x)−ϕ(z)∣∣2
registration- based classification
people what to do brain segmentation, we have rigid representation and no-rigid representation. you minimize the energy to make the brain more look like each other.
Multiple Instance Learning
- use local information
- detecting activity of action units
orginal goal = twofold
MIL aims to classify new and previously unseen bags as accurate as possible
- MI-svm:
first iteration:
- conpy the bag label to the instance label
- train a standard SVM
subsequent iterations :
- choose a single instance in every positive bag on the correct side of the decision boundaty
- retrain