生成对抗网络gans
介绍 (Introduction)
Generative Adversarial Networks (GANs) were first introduced in a paper by Goodfellow and other researchers at the University of Montreal in 2014. Since then we have seen significant development in GANs and its various types. GANs can be thought of as a robot artist who can learn and mimic any distribution. This includes images, speech, music, text, etc. They have great potential for generating fake information which is very similar to the real ones. As a result, GANs have a variety of applications like,
生成对抗网络(GANs)最早是由Goodfellow和其他研究人员于2014年在蒙特利尔大学发表的一篇论文中介绍的。自那时以来,我们已经看到GAN及其各种类型的显着发展。 可以将GAN视为可以学习和模仿任何分布的机器人艺术家。 其中包括图像,语音,音乐,文本等。它们具有产生与真实信息非常相似的伪造信息的巨大潜力。 结果,GAN具有多种应用,例如,
- Generation of human faces for criminal investigations 为刑事调查生成人脸
- Text-to-image translation 文字到图片翻译
- Image-to-image translation 图像到图像翻译
- Photo editing/ Photo blending etc. 照片编辑/照片融合等
Before moving on to GANs let’s first have a look at generative models
在转到GAN之前,我们先来看一下生成模型
什么是生成模型 (What are Generative Models)
A generative model aims to learn a data distribution using unsupervised learning and then generate new similar data points with some variation. Let us review the idea of generative models by considering supervised vs. unsupervised and discriminative vs. generative modeling.
生成模型旨在使用无监督学习来学习数据分布,然后生成具有一些变化的新相似数据点。 让我们通过考虑监督模型与非监督模型以及判别模型与生成模型来回顾生成模型的思想。
A modeling technique that maps an input to an output given a labeled data is called supervised learning. It requires a labeled training data with input samples X and labels y. The aim here is to learn a function that maps the input values to the output values. Then this newly learned function is used to predict new values. Using supervised learning we can predict the class labels (y). This task of predicting the class labels is called classification aka discriminative modeling.
在给定标记数据的情况下将输入映射到输出的建模技术称为监督学习。 它需要带有输入样本X和标签y的标记训练数据。 此处的目的是学习将输入值映射到输出值的函数。 然后,该新学习的函数将用于预测新值。 使用监督学习,我们可以预测班级标签(y)。 预测类标签的这项任务称为分类又称为判别建模 。
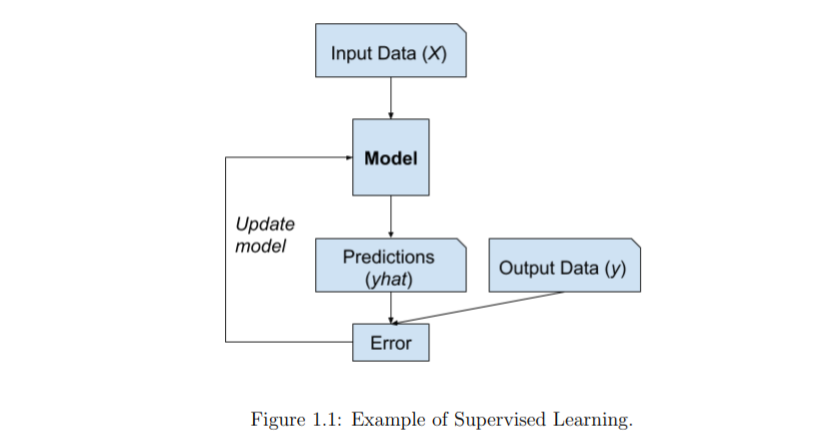
While in case of unsupervised learning the data is not labeled. Here the goal is to find a pattern in the input samples X. Thus, using unsupervised learning we can find patterns and summarize the input examples and generate new examples of similar types. These are called as generative models.
在无监督学习的情况下,数据不会被标记。 这里的目标是在输入样本X中找到模式。因此,使用无监督学习,我们可以找到模式并汇总输入示例,并生成相似类型的新示例。 这些被称为生成模型 。
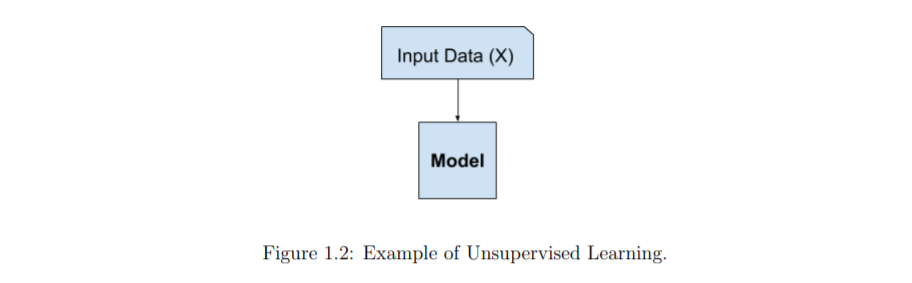
什么是生成对抗网络(GAN) (What are Generative Adversarial Networks (GANs))
First, let’s understand each word in the term Generative Adversarial Network. In the previous part, we saw that are generative models. Since GAN is a generative model, hence the term ‘Generative’.
首先,让我们了解术语“生成对抗网络”中的每个单词。 在上一部分中,我们看到了生成模型。 由于GAN是生成模型,因此称为“ 生成” 。
Now let’s understand the term ‘Adversarial’ . To understand this, we will take a typical example of a detective (discriminator) and a counter fitter (generator). Here we have two adversaries; the counter fitter and the detective. The aim of the counter fitter is to generate fake 1-dollar bills and the aim of the detective is to determine if the dollar bill in real or fake. Thus, we have a game like scenario where the generator has to compete against an adversary i.e. the discriminator.
现在让我们了解“ 对抗”一词。 为了理解这一点,我们将以侦探(区分器)和反向装配工(生成器)的典型示例为例。 这里有两个对手; 柜台钳工和侦探。 柜台钳工的目的是生成假的1美元面额的钞票,而侦探的目的是确定美钞是真实的还是假的。 因此,我们有一个类似于游戏的场景,其中生成器必须与对手(即鉴别器)竞争。
If the generator fails in fooling the discriminator then we update the generator to do a better job and similarly if the discriminator fails to determine the counter fitted bills then we update the discriminator to do a better job.
如果生成器无法欺骗鉴别器,则我们将更新生成器以更好地完成工作,类似地,如果鉴别器无法确定适合柜台的纸币,则我们将更新鉴别器以更好地完成工作。
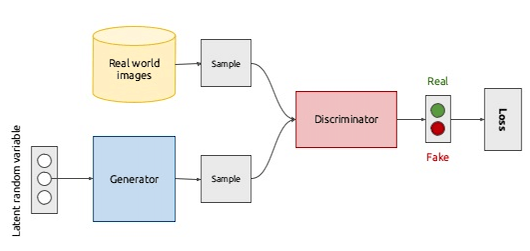
In the beginning, the generator doesn’t know how to make 1-dollar bills, so it outputs random noise. Similarly, the discriminator doesn’t know how to distinguish real and fake bills and thus, it makes a random decision. But thorough time both compete against each other giving them a chance to improve. When this training converges the discriminator cannot tell the difference between real and fake 1-dollar bills. This is because the generator is now creating samples that look exactly similar to the original one.
最初,生成器不知道如何制作1美元的钞票,因此它会输出随机噪声。 同样,鉴别者也不知道如何区分真钞和假钞,因此会做出随机决定。 但是彻底的时间彼此竞争,使他们有机会提高。 当该训练收敛时,判别器无法分辨真实的和伪造的1美元钞票之间的区别。 这是因为生成器现在正在创建看起来与原始样本完全相似的样本。
This competition between the two adversaries is what is meant by the term ‘Adversarial’.
这两个对手之间的竞争就是“ 对手 ”一词的含义。
Lastly, the term ‘Network’ in GAN refers to neural networks. This is because both the generator and the discriminator are implemented with neural networks. Neural networks are preferred because a neural network can approximate about any function.
最后,GAN中的“ 网络”一词是指神经网络。 这是因为生成器和鉴别器都是通过神经网络实现的。 优选神经网络,因为神经网络可以近似任何功能。
Now let’s understand some terminologies related to GANs
现在让我们了解一些与GAN相关的术语
潜在空间 (Latent space)
Latent space is a vector space that comprises of latent variables (Latent variables are variables that cannot be observed directly). This latent space provides a compressed representation of the input distribution. The latent space has no meaning apart from the meaning applied to it by the generator model. The GAN tries to map the input distribution in this latent space to generate new output. Once the training is completed, we can explore this latent space and interpolate between points and perform arithmetic operations on the points to generate a targeted output.
潜在空间是一个由潜在变量组成的向量空间(潜在变量是无法直接观察到的变量)。 该潜在空间提供了输入分布的压缩表示。 潜在空间除了生成器模型对其施加的含义外没有其他含义。 GAN尝试映射此潜在空间中的输入分布以生成新的输出。 训练完成后,我们可以探索该潜在空间并在点之间进行插值,并对这些点执行算术运算以生成目标输出。
发电机型号和上采样 (Generator Model and upsampling)
The generator model generates samples in the problem domain from a fixed-length noise given as input. This input vector is chosen from a random distribution. Upon training the generator applies meaning to the points in the latent space and thus, new points from the latent space provided as input can be used to generate new output examples.
生成器模型从作为输入给出的固定长度噪声中生成问题域中的样本。 该输入向量是从随机分布中选择的。 训练后,生成器对潜在空间中的点应用含义,因此,可以将潜在空间中作为输入提供的新点用于生成新的输出示例。
Thus, the generator has to translate the salient features to a detailed and dense output. To do this it has to perform the inverse of pooling operation. This technique of transforming a small input to a larger output is referred to as upsampling. The simplest way of upsampling is to double each row and column as shown below.
因此,生成器必须将显着特征转换为详细而密集的输出。 为此,它必须执行逆池操作。 这种将较小的输入转换为较大的输出的技术称为上采样。 上采样的最简单方法是将每一行和每一列加倍,如下所示。
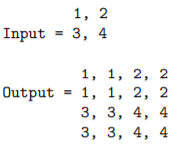
However, this type of upsampling does not perform any learning. It is not able to fill in useful data in the process of upsampling. In order to make it useful, it has to be followed by a convolution operation.
但是,这种类型的上采样不会进行任何学习。 它无法在上采样过程中填充有用的数据。 为了使其有用,必须进行卷积运算。
鉴别模型 (Discriminator Model)
The discriminator is a classification model. It takes in input samples and predicts whether the image is real or fake. The input can either be from the training set or from the generator’s output. It performs the traditional CNN for image classification related tasks like downsampling. The discriminator can be discarded after the training process is completed.
鉴别器是分类模型。 它接受输入样本并预测图像是真实的还是假的。 输入可以来自训练集,也可以来自发电机的输出。 它执行传统的CNN,用于图像分类相关任务,例如下采样。 训练过程完成后,可以将鉴别器丢弃。
加工 (Working)
So, the entire working of GANs can be put together in the following steps,
因此,可以按照以下步骤将GAN的全部工作组合在一起,
- Generator takes in random noise 发电机吸收随机噪声
- Generator returns an image 生成器返回图像
- The discriminator is provided with real images from the distribution and fake images from the generator 向鉴别器提供来自分发的真实图像和来自生成器的伪图像
- Discriminator returns probabilities of the images being real or fake 鉴别器返回图像是真实的还是伪造的概率
Below you can see how this process really works. Here the generator is trying to fit a simple line. The Green dots represent the real samples and the Purple dots represent the fake samples generated by the generator
在下面,您可以看到此过程的真正工作原理。 在这里,生成器试图拟合一条简单的线。 绿色点代表真实样本,紫色点代表发生器生成的假样本
Check out https://poloclub.github.io/ganlab/ for more such visualizations.
查看https://poloclub.github.io/ganlab/了解更多此类可视化。
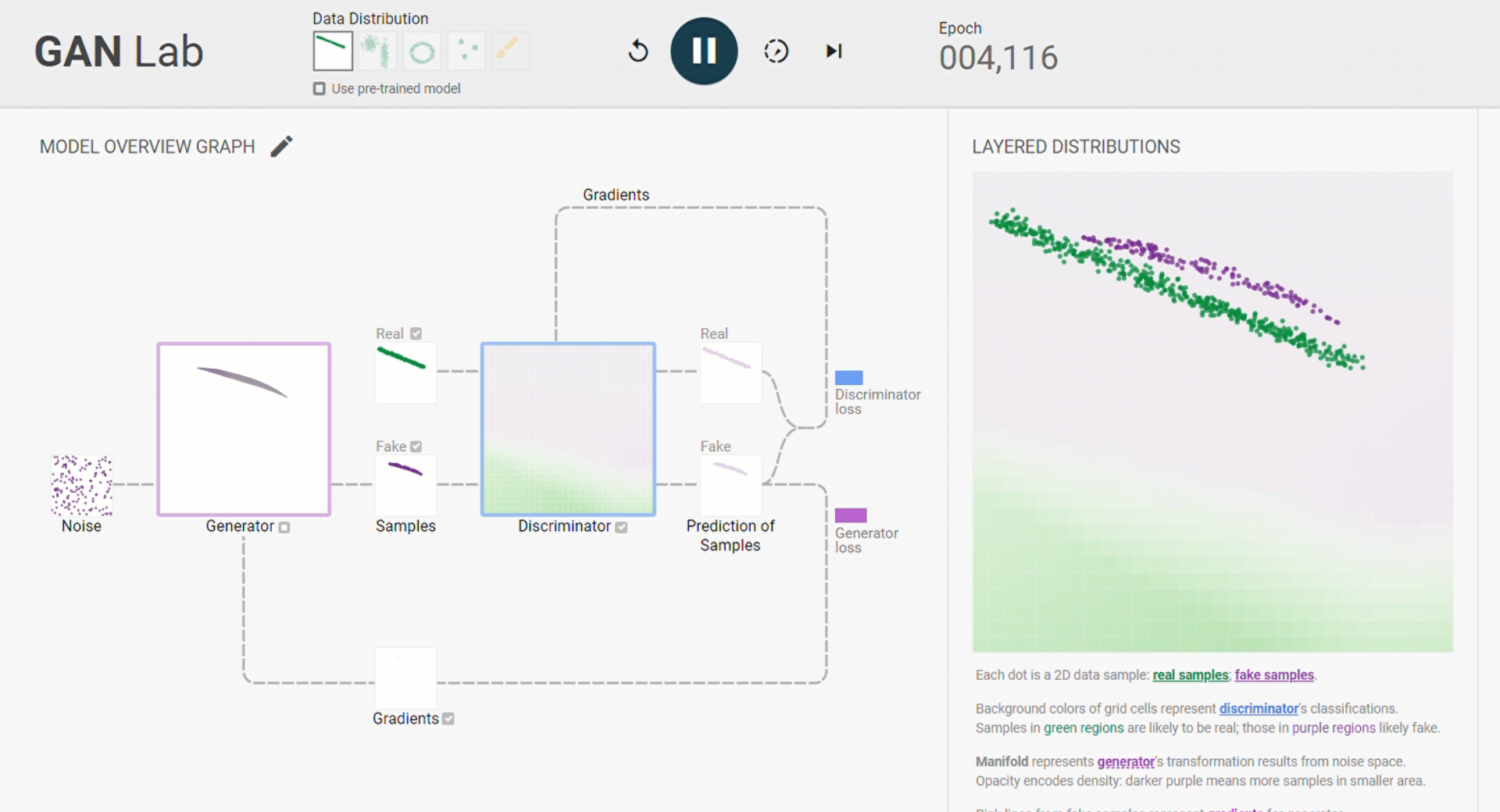
Generative Adversarial Networks with Python — Jason Brownlee
使用Python生成的对抗网络-Jason Brownlee
生成对抗网络gans