生物信息学生物影像
Technology and Scale’s love affair is well-known, but their adoption of Biology has had its rough patches. Why is now different?
Technology and Scale的恋爱是众所周知的,但是他们对Biology的采用却遇到了困难。 为什么现在不同?
From the early days of high throughput compound screening, to the more recent promises of genomics in precision medicine, the biotech industry has tended to overestimate the impact of “scale” on discovery.
从高通量化合物筛选的早期到精确医学中基因组学的最新发展,生物技术行业都倾向于高估“规模”对发现的影响。
This is scale in experimentation, process, synthesis, and computation. Scale that has emerged in the forms of crafty chemistry combinatorics to create millions of compounds, gargantuan screening facilities capable of speedy readouts, massive omics datasets, and much more.
这是实验,过程,合成和计算的规模。 规模已经以诡计多端的化学组合剂的形式出现,以创建数百万种化合物,能够快速读数的庞大筛选设备,庞大的组学数据集等等。
And those are the legitimate efforts. I’m not even going to get into the unproven claims of scale that can pop up in biotech, à la a certain company ending in “heranos”.
这些都是合法的努力。 我什至不会涉足生物技术中可能出现的未经证实的规模要求, 以“ heranos ” 结尾的某家公司。
While not entirely in vain, attempts at transforming biological discovery with scale have often fallen short, or at the very least taken far longer than expected to buck the hype cycle and make it out of the trough of disillusionment. The massive data sets traditionally produced (or pieced together) as a result of biology at scale have been in many ways flawed, resulting in limited translational value. Further still, there has been a gap between the massive data we currently have available, and our ability to translate that data into something we can run tangible experiments against.
尽管并非徒劳无功,但试图大规模地转变生物发现的尝试往往失败了,或者至少比预期的要花费更长的时间才能打破炒作周期并使之脱离幻灭的低谷 。 传统上由于大规模生物学而产生(或拼凑)的海量数据集在许多方面都存在缺陷,导致翻译价值有限。 更进一步,我们目前可获得的海量数据与我们将数据转换为可以进行有形实验的能力之间还存在差距。
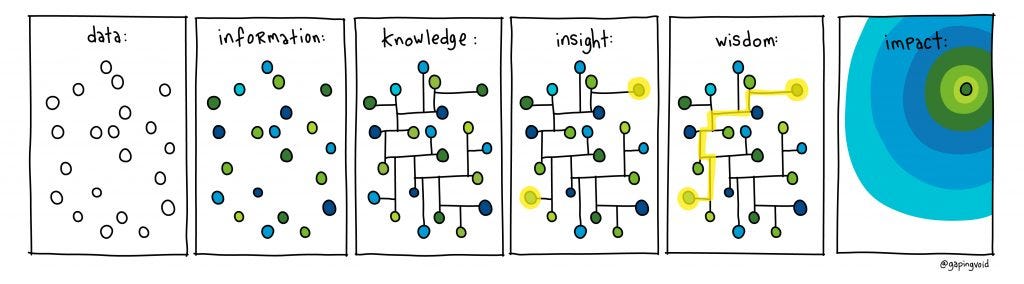
In other words, More is not Better, biology is complex, and the biotech industry has the costly clinical failures to show for it. Adding insult to injury, the term “Eroom’s Law” has been coined, contrasting the negative trends in drug discovery success rate with the ever-upwards climb of compute power in accordance with Moore’s Law.
换句话说,更多不是更好,生物学是复杂的,而生物技术产业有昂贵的临床失败可证明这一点。 不仅增加了侮辱性伤害,还创造了“室定律”一词,将药物发现成功率的负面趋势与根据摩尔定律不断提高的计算能力进行了对比。
But there’s a light. Even in the past decade, something of a reversal in the success rate of drug development has occurred.
但是有灯。 即使在过去的十年中,药物开发的成功率也发生了逆转 。
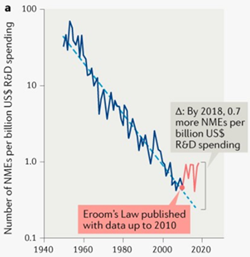
There are many reasons for this potential about-face (friendly FDA, rare genetic disease focus, etc.), but the industry’s re-imagining of what scale means is one reason that becomes ever-more relevant. We are now at a juncture in how we generate and interpret biology at scale.
这种潜在的面貌有很多原因(友好的FDA,罕见的遗传疾病重点等),但是业界重新思考规模的含义是变得越来越重要的原因之一。 现在,我们正处于大规模生成和解释生物学的关键时刻。
We are moving out of an era of brute force in biological experimentation, and into a new era of relevant, intelligent, and validated scale in biology.
我们正在摆脱生物学实验的蛮力时代,进入生物学相关,智能和经过验证的规模的新时代。
This application of scale is poised to actually disrupt biological discovery productivity. In this new era terms like “high throughput”, “massive”, and “automated”, will prove real merit, instead of triggering immediate skepticism in the eyes of your friendly neighborhood pharma executive.
这种规模的应用有望真正破坏生物发现的生产力。 在这个新时代,诸如“高通量”,“大规模”和“自动化”之类的术语将被证明是真正的优点,而不是引起您附近的社区药业高管眼前的怀疑。
We will see functional genomics platforms identifying and validating biological targets at unprecedented speeds; the advent of relevant computational approaches quickly narrowing solution spaces; the rapid, intelligent optimization of technologies that up-level our control over biology. And much more.