Traffic-sign recognition (TSR) technology- a technology by which a vehicle is able to recognize the traffic signs that are placed on the road e.g. “ Turn right ahead”, “Speed limit”, or “Stop” etc.- can be implemented using CNNs. This is important because a prompt response to real-time traffic events can prevent road accidents.This article will explain all the steps taken to design a Deep Learning model to do that.
可以实施交通标志识别 (TSR)技术-一种车辆能够识别道路上交通标志的技术,例如“向右转”,“限速”或“停车”等。使用CNN。 这很重要,因为对实时交通事件做出SwiftReact可以预防道路交通事故。本文将解释设计深度学习模型以实现此目的的所有步骤。
1.导入库和数据集 (1. Importing Libraries and Dataset)
Key python libraries will be imported.
关键的python库将被导入。
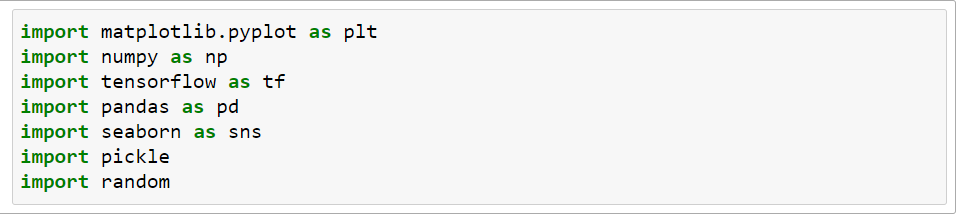
The dataset used is a German Traffic Sign Dataset. We will use around 34,800 images for training dataset, 12630 images for test dataset and 4410 images for validation dataset.
使用的数据集是德国交通标志数据集 。 我们将使用约34,800张图像作为训练数据集,使用12630张图像作为测试数据集,并使用4410张图像作为验证数据集。
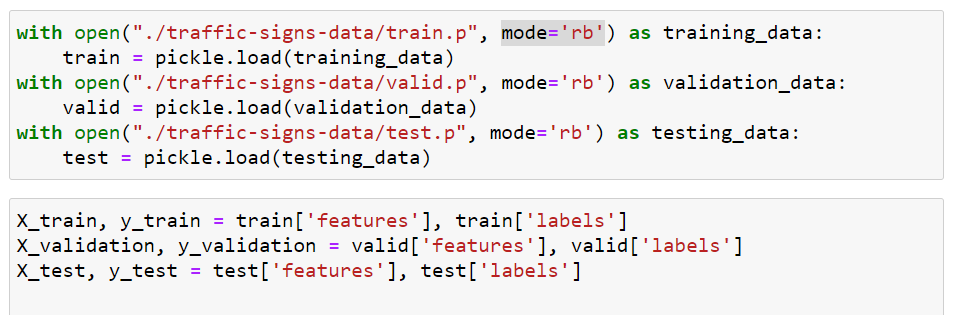
2.显示数据集 (2. Displaying the Dataset)
You can display the dataset using the following code:
您可以使用以下代码显示数据集:
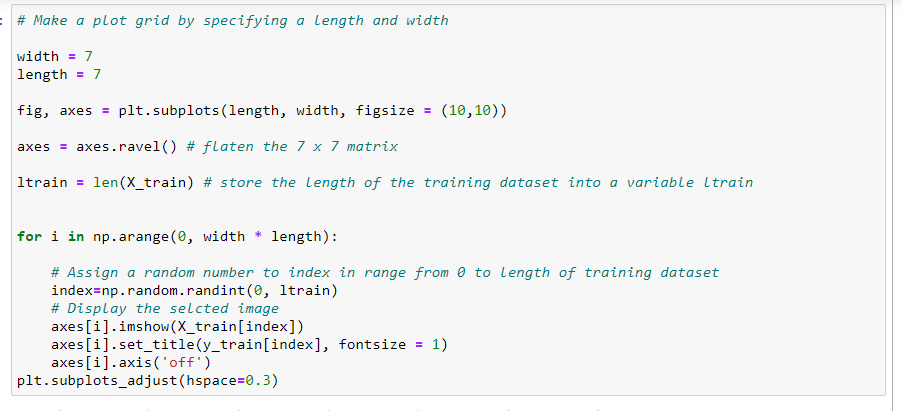
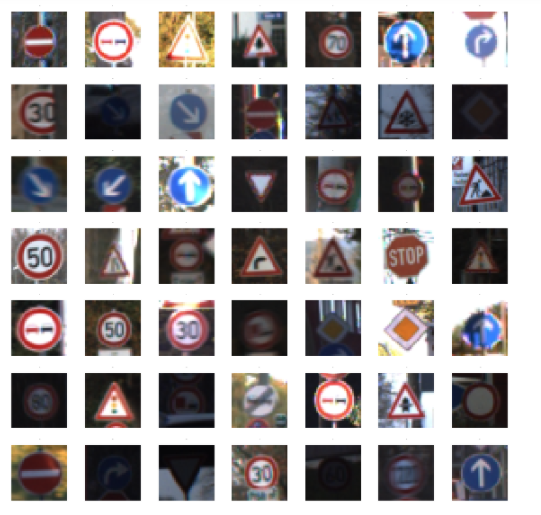