机器学习 开始
前言 (Preface)
Below is an essay I had written for a university requirement. I admit, it’s pretty rough. I attempt to condense two essays into the word count of one, and I don’t go into as much depth as perhaps I would’ve liked.
以下是我为大学要求而写的文章。 我承认,这很粗糙。 我试图将两篇文章压缩成一个单词的单词数,但没有深入到我可能想要的深度。
But it reflects an important transformation in my thinking around mental health. Therefore I’ve decided to present it.
但这反映了我对心理健康的看法发生了重要的转变。 因此,我决定介绍一下。
I grew up in a semi-conservative middle class Immigrant family. There isn’t really much link between this kind of upbringing and mental health except for the fact my father was a psychiatrist. Was he the kind of psychiatrist that had patients lounge on a sofa and confess their concerns to the ceiling? Nope. He was a self-professed biological psychiatrist; adamant that psychiatric pathologies had some biological root that, once revealed, would be the nucleus for the next great psychopharmacological revolution.
我在一个半保守的中产阶级移民家庭中长大。 这种养育与心理健康之间并没有多大关系,除了我父亲是一名精神科医生。 他是那种让患者躺在沙发上并向天花板承认自己的担忧的精神科医生吗? 不。 他是一个自称生物精神病医生; 坚持认为精神病学病理学具有某些生物学根源,一旦揭示出来,它将成为下一次伟大的心理药理学革命的核心。
Now, he didn’t believe that human connection and experience was irrelevant. His view was more so one borne out of frustration by the lack of progress (and sometimes event destructive effects of) psychological and Freudian psychodynamic approaches. See, psychiatry never had the same kind of “scientific revolution” that other fields have had (e.g. heart transplantation, dialysis, penicillin). Drugs were discovered to have transformative effects on mental health really by accident. And even now, the understanding of psychopharmacology is nascient in comparison to fields like cardiology or oncology.
现在,他不认为人类之间的联系和经验无关紧要。 他的观点更多地是由于缺乏心理学和弗洛伊德心理动力学方法的进步(有时是事件的破坏性影响)而感到沮丧。 精神病学从来没有像其他领域那样经历过“科学革命”(例如心脏移植,透析,青霉素)。 发现药物确实是偶然地对心理健康产生了变革性影响。 甚至到现在,与心脏病学或肿瘤学等领域相比,对心理药理学的了解还只是初期。
Enter machine learning. Now, I’m a medical student, not a computer scientist. My only knowledge of machine learning is that it might make radiology obsolete. So, when the opportunity to write a 3000 word essay on AI and medicine, it was an opportunity that provided a completely different perspective. I’ll leave my personal ramblings here, and allow you to read the (below par) work below.
进入机器学习。 现在,我是一名医学生,而不是计算机科学家。 我对机器学习的唯一了解是,它可能会使放射学过时。 因此,当有机会撰写有关AI和医学的3000字论文时,这是一个提供了完全不同观点的机会。 我将在这里留下我的个人杂谈,并允许您阅读下面的(低于面值)工作。
人工智能在心理健康中的应用 (AI’s application in mental health)
介绍 (Introduction)
One can appreciate artificial intelligence’s (AI’s) implication in mental health through the context of two disease: depression and schizophrenia. These are two conditions that are unrelated, and present two different problems. Depression is one of the most prevalent diseases worldwide[1]. ON the other hand, schizophrenia is one of the most disabling mental health diseases[2].
人们可以通过两种疾病(抑郁症和精神分裂症)来体会人工智能(AI)对心理健康的影响。 这是两个不相关的条件,并且存在两个不同的问题。 抑郁症是世界上最流行的疾病之一[1]。 另一方面,精神分裂症是最致残的精神健康疾病之一[2]。
Researchers have used imaging modalities like MRI scanning and EEG imaging to develop algorithms that can diagnose patients with depression and schizophrenia. In areas like research, AI is being applied differently for both. In depression, researchers are using AI to subclassify the disease and discover new subtypes of depression based on differences in neuroanatomy picked up by the machine learning algorithm[3]. In schizophrenia, AI is attempting to discover molecular phenotypes of genes associated with schizophrenia[4]. AI is also being used in the prediction of suicide[5]: the most severe consequence of both depression and schizophrenia[2,6].
研究人员已使用MRI扫描和EEG成像等成像方式来开发可诊断患有抑郁症和精神分裂症患者的算法。 在研究等领域,两者的人工智能应用有所不同。 在抑郁症中,研究人员正在使用AI对疾病进行分类,并根据机器学习算法发现的神经解剖学差异来发现抑郁症的新亚型[3]。 在精神分裂症中,人工智能正试图发现与精神分裂症有关的基因的分子表型[4]。 AI也被用于自杀预测[5]:抑郁症和精神分裂症的最严重后果[2,6]。
诊断 (Diagnosis)
Diagnosis of depression has been a major challenge in the field of psychiatry. One significant issue is that despite being prevalent, it remains misdiagnosed due to poor sensitivity and specificity[1]. This might be due to current methods of diagnosis as being inadequate, as diagnosis is not based on an objective clinical measurement as highlighted when discussing its heterogeneity[2].
抑郁症的诊断一直是精神病学领域的主要挑战。 一个重要的问题是,尽管它很普遍,但由于敏感性和特异性差而仍被误诊[1]。 这可能是由于当前的诊断方法不充分所致,因为诊断并非基于讨论异质性时强调的客观临床测量[2]。
Artificial intelligence has been extensively applied to the area of depression and schizophrenia diagnostics. Specifically, there has been an interest in using AI to classify neuroimaging to determine whether a patient has depression. It does this by creating multi-modal data from imaging and using it to separate a training set of patients and controls. The effectiveness can be tested with a set the algorithm hasn’t seen.
人工智能已广泛应用于抑郁症和精神分裂症的诊断领域。 具体而言,人们对使用AI对神经影像进行分类以确定患者是否患有抑郁症感兴趣。 它通过从成像创建多模态数据并将其用于分离患者和控制人员的训练集来实现。 可以使用该算法尚未看到的集合来测试其有效性。
One modality being explored for use with AI in depression diagnostics is MRI imaging. IN one study, researchers took diffuse tensor MRI scans from 44 healthy controls (HC) and 52 patients with Major Depressive Disorder (MDD). They trained, then tested a Support Vector Machine Learning (SVM ML) algorithm to be able to separate patients into either MDD or HC classifications[7].
MRI成像正在探索与AI一起用于抑郁症诊断的一种方式。 在一项研究中,研究人员对44位健康对照(HC)和52位严重抑郁症(MDD)患者进行了弥散张量MRI扫描。 他们训练并测试了支持向量机学习(SVM ML)算法,以将患者分为MDD或HC分类[7]。
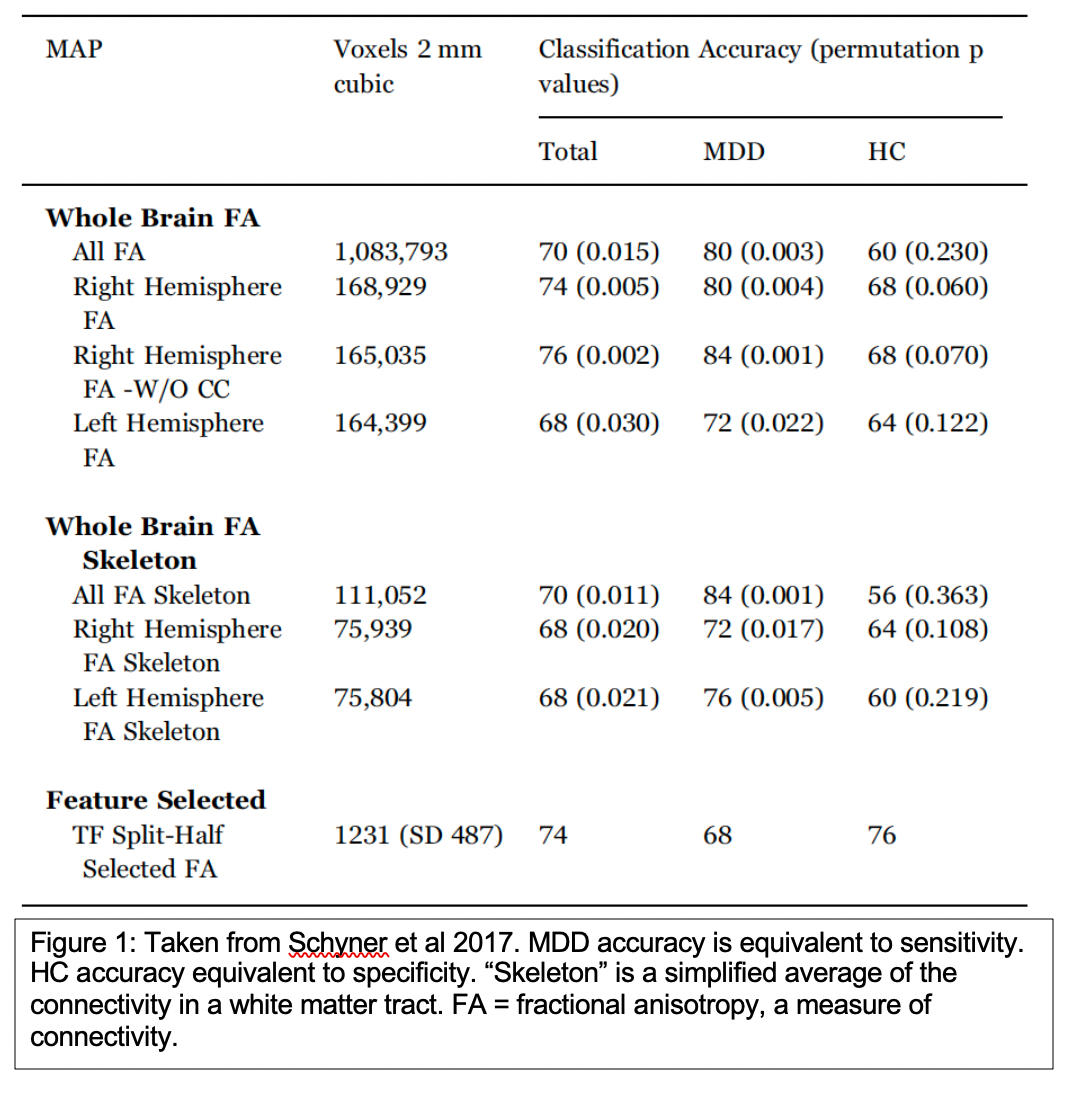

They looked at different connectivity patterns in the whole brain; right; and left hemispheres to find the algorithm with the highest accuracy of classification.
他们研究了整个大脑中不同的连接方式; 对; 然后从左半球中找到分类精度最高的算法。
The mean sensitivity of the algorithm was 82%, whereas the mean specificity for the algorithms was 50%[7]. From this its clear the SVM algorithm was over diagnosing patients with MDD. It may be because the training and testing data set are from the same site, resulting in overfitting. As we’ll discuss in the Wider Discussion segment, this is an important consideration for AI algorithms.
该算法的平均灵敏度为82%,而该算法的平均特异性为50%[7]。 由此可见,SVM算法在诊断MDD患者方面已过分。 可能是因为培训和测试数据集来自同一站点,导致过拟合。 正如我们将在“更广泛的讨论”部分中讨论的那样,这是AI算法的重要考虑因素。
Little evidence exists around the accuracy of psychiatric diagnosis to compare to, however one study looking at the accuracy of GP diagnosis of MDD found that their sensitivity was 50.1% but their specificity was 81.3%[8]. The high specificity and low sensitivity suggests GPs may underdiagnose depression.
精神病诊断的准确性与之相比,鲜有证据可比,但是一项针对MDD GP诊断的准确性的研究发现,其敏感性为50.1%,而特异性为81.3%[8]。 高特异性和低敏感性提示GPs可能无法诊断抑郁症。
This suggests rather than AI being solely responsible for diagnosis, MRI diagnostics may be used in combination with the judgement of healthcare professionals for the best diagnostic outcome. However, to know this for sure, studies must be done to evaluate the effectiveness of psychiatric diagnosis, as well as AI assisted psychiatric diagnosis.
这表明,不是由AI独自负责诊断,而是可以将MRI诊断与医疗专业人员的判断结合起来使用,以获得最佳的诊断结果。 但是,要确定这一点,就必须进行研究以评估精神病诊断以及AI辅助的精神病诊断的有效性。
Much like depression, evidence has existed about the role of neuroanatomy in schizophrenia development. Some evidence suggests that there are neuroanatomical differences between patients with and without schizophrenia[9]. As we’ve seen before with depression, AI could be used in conjunction with modalities that measure brain structure and function to diagnose schizophrenia.
与抑郁症很像,存在关于神经解剖学在精神分裂症发展中的作用的证据。 有证据表明,精神分裂症患者与非精神分裂症患者在神经解剖学上存在差异[9]。 正如我们以前在抑郁症中所见,可以将AI与测量大脑结构和功能的模式结合使用,以诊断精神分裂症。
Similar to the use of MRI imaging in depression, studies have fed MRI scans of patients with schizophrenia into AI algorithms with the purpose of classification. One study took MRI imaging from 941 patients from 5 different sites and used these to train and validate different algorithms.[9]
与在抑郁症中使用MRI成像相似,研究将精神分裂症患者的MRI扫描用于AI算法,以进行分类。 一项研究对来自5个不同地点的941名患者进行了MRI成像,并将其用于训练和验证不同的算法。[9]

This paper used multiple sites for training, then sites the algorithm hadn’t seen for validation (SETs 4 and 5). This is important for testing whether the algorithm was “overfitting”, in that the algorithm used non-generalizable set-specific factors to diagnose patients. Given the testing sets were from different sites, overfitting would have resulted in a lower accuracy. From this table, you can see AUC was over 0.7 for pooled data from SETs 1–3 when tested against SET 4 and 5.[9]
本文使用了多个站点进行训练,然后使用该算法未进行验证的站点(SET 4和5)。 这对于测试算法是否“过拟合”很重要,因为该算法使用了不可泛化的特定于集合的因素来诊断患者。 如果测试集来自不同的站点,则过度拟合会导致精度降低。 从该表中,您可以看到,针对SET 4和5进行测试时,SET 1–3中合并数据的AUC超过0.7。[9]
However, when individual sites were used to train the data, the accuracy was more variable when tested with external site data. The average AUC for algorithms tested against data from site 4 trained against individual site data (so sites 1–3) was 0.684/0.659, which is not high enough to indicate significance. When comparing it to the algorithm when trained with all three sites (0.731/0.738), it demonstrates the importance of multi-site training and validation.[9]
但是,当使用单个站点来训练数据时,使用外部站点数据进行测试时,准确性的可变性更大。 针对来自站点4的数据(针对站点1-3)进行训练的算法所测试算法的平均AUC为0.684 / 0.659,不足以表明其重要性。 当将其与在所有三个站点训练时的算法(0.731 / 0.738)进行比较时,它证明了多站点训练和验证的重要性。[9]
There is another imaging modality that could be used: the electroencephalogram (EEG). The application is similar to MRI imaging: an algorithm is developed from EEGs to diagnose patients with depression or schizophrenia.
可以使用另一种成像方式:脑电图(EEG)。 该应用类似于MRI成像:从EEG开发了一种算法来诊断患有抑郁症或精神分裂症的患者。
There is evidence of EEG data being used to create AI algorithms that could diagnose depression and schizophrenia. IN fact, according to one systematic review, 17.1% of papers looking at AI applications in depression were using EEG data.[10]
有证据表明,脑电数据可用于创建可诊断抑郁症和精神分裂症的AI算法。 实际上,根据一项系统评价,研究抑郁症中AI应用的论文中有17.1%使用的是EEG数据。[10]

The accuracy from EEGs has been more impressive than MRI imaging. Since 2012, using a variety of AI techniques including machine learning and neural networks, the accuracy of AI/EEG algorithms have been over 80%, with a mean accuracy of 94.4%.[11]
脑电图的准确性比MRI成像更令人印象深刻。 自2012年以来,使用包括机器学习和神经网络在内的各种AI技术,AI / EEG算法的准确性已超过80%,平均准确性为94.4%。[11]

What’s interesting is that the average accuracy using the SVM algorithm ( the ML algorithm used in the MRI depression paper) is 92.5%[11], which is greater than the mean total classification accuracy of MRI, which was 66.7%[7]. Combine this with the fact that EEG’s are cheaper and easier to use, and it would be sensible to assume that if AI assisted diagnosis of depression were to occur, it would do so using data from EEGs. That being said, the superior localisation and visualisation power of MRI lends itself well to AI assisted research, a point we explore later.
有趣的是,使用SVM算法(MRI抑郁症论文中使用的ML算法)的平均准确性为92.5%[11],高于MRI的平均总分类准确性为66.7%[7]。 将此与脑电图便宜且易于使用这一事实结合起来,可以合理地假设,如果要进行AI辅助的抑郁症诊断,则可以使用脑电图的数据来进行。 话虽这么说,MRI优越的定位和可视化功能非常适合AI辅助研究,这是我们稍后探讨的重点。
AI assisted EEG diagnosis shows promise with schizophrenia as well. One study looked at three different types of EEG data: source-level data, sensor-level data and a combination of both. It found accuracies greater than 70% across all three modalities.[12]
人工智能辅助的脑电图诊断也显示出精神分裂症的前景。 一项研究研究了三种不同类型的EEG数据:源级别的数据,传感器级别的数据以及两者的组合。 它发现在所有三种方式中的准确性都超过70%。[12]

What’s interesting is that the average accuracy increases when using a combination of EEG data types, rather than using one or the other. This is also seen with algorithms using MRI data from different sites to train its algorithms. The important distinction is that this paper shows using multiple modalities produces more accurate results, whereas the other paper suggests using more data from multiple sites does the same. Both demonstrate that the accuracy of AI increases with data of greater complexity and volume.
有趣的是,当使用EEG数据类型的组合而不是使用其中一种时,平均准确性会提高。 使用来自不同站点的MRI数据来训练其算法的算法也可以看到这一点。 重要的区别在于,本文显示使用多种方法可以产生更准确的结果,而另一篇论文则建议使用来自多个站点的更多数据也可以做到这一点。 两者都表明,随着数据复杂性和数量的增加,AI的准确性也会提高。
研究 (Research)
We don’t have a neuropathological model of depression. Its been suspected that there is a neuroanatomical basis for the disease, but the results have not been consistent. One explanation for this uncertainty is that depression is a uniform disease. [3] Depression is heterogenous, and can vary in its presentation. For instance, a patient can be diagnosed with the same label of MDD despite having different symptom presentations, as a combination of up to nine symptoms is required for a diagnosis, according to NICE.[13]
我们没有抑郁症的神经病理模型。 人们怀疑该疾病具有神经解剖学基础,但结果并不一致。 这种不确定性的一种解释是,抑郁症是一种统一的疾病。 [3]抑郁症是异质性的,其表现形式可能有所不同。 例如,根据NICE的报道,尽管症状表现不同,但仍可以用相同的MDD标签诊断患者,因为诊断最多需要结合9种症状。[13]
One landmark paper used unsupervised machine learning to subclassify patients with depression into 4 groups based not on their symptom profile but differences in fMRI imaging. Each subtype was based on differences in fMRI connectivity patterns in different parts of the brain (see figure 7).[13]
一篇具有里程碑意义的论文使用无监督机器学习将抑郁症患者分为四类,这不是基于他们的症状特征,而是基于功能磁共振成像的差异。 每个亚型均基于大脑不同部位的fMRI连接模式的差异(见图7)。[13]

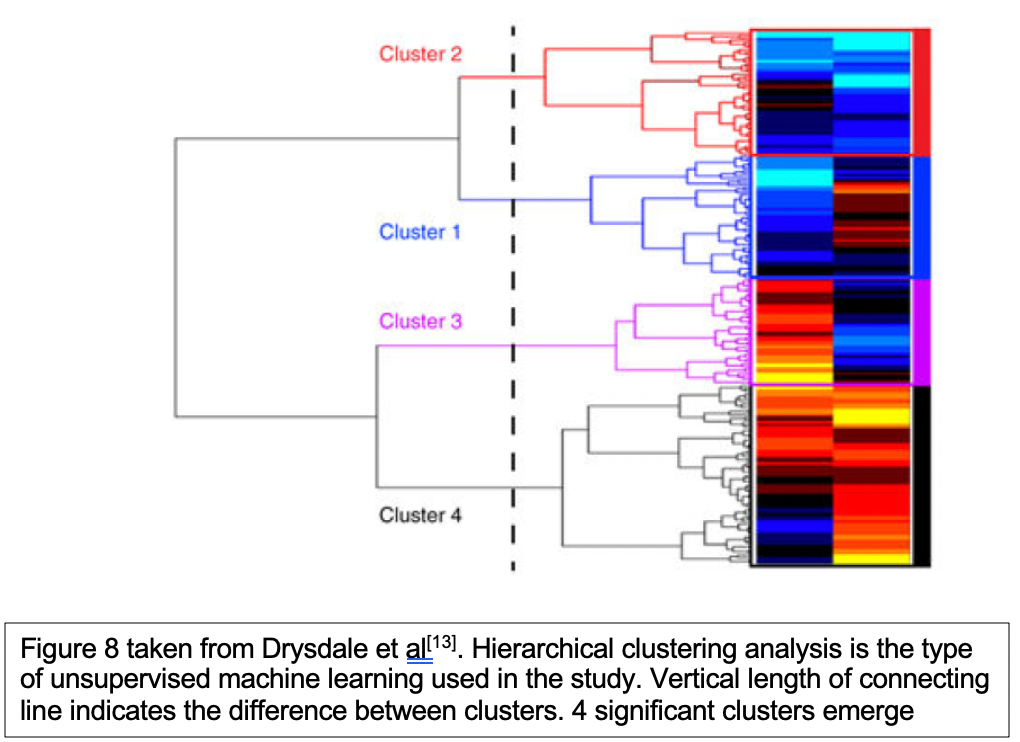
They found that where the anatomical structures between the groups were similar, those regions were associated with the most common depression symptoms (“anhedonia”, “fatigue” and “mood” symptoms). The more “abnormal” the connection, the more severe the symptoms.
他们发现,在两组之间的解剖结构相似的地方,这些区域与最常见的抑郁症状(“快感不足”,“疲劳”和“情绪”症状)相关。 连接越“异常”,症状就越严重。
They were also able to come up with unique symptom profiles associated with each subtype (see figure 9).[13]
他们还能够提出与每种亚型相关的独特症状特征(见图9)。[13]

To test whether the subtypes were just based in irrelevant variation in the data sample or whether they were based on true neuroanatomical differences in patients with depression, the researchers used the SVM machine learning algorithms for each subtype and applied them to an external data set. [13]
为了测试亚型是仅基于数据样本中的不相关变异还是基于抑郁症患者的真实神经解剖学差异,研究人员针对每种亚型使用了SVM机器学习算法,并将其应用于外部数据集。 [13]

The results as shown in figure 10 show that each subtype had an accuracy greater than 80%, and that the algorithm could correctly identify 84% of patients with depression. When data was carefully selected (segmented bars), accuracy increased to 93.3%.[13]
如图10所示,结果表明每种亚型的准确性均高于80%,并且该算法可以正确识别84%的抑郁症患者。 仔细选择数据(分段条形图)后,准确性提高到93.3%。[13]
This suggests that subclassification of depression help improve accuracy, when you compare these results to the results of the MRI study shown in depression part. This is significant as diagnosis was improved with the application of AI-assisted research.
这表明,将这些结果与抑郁症部分显示的MRI研究结果进行比较时,抑郁症的分类有助于提高准确性。 这是重要的,因为通过AI辅助研究的应用可以改善诊断。
For schizophrenia, there is another application for research. Schizophrenia is a polygenetic disease[2]. There is evidence of high probability of inheritance. The problem , is understanding the relationship with the genes associated with schizophrenia and the phenotypes they are responsible for. Understanding this relationship can elucidate more information about the development and pathophysiology of the disease.[4]
对于精神分裂症,还有另一项研究用途。 精神分裂症是一种多基因疾病[2]。 有证据表明遗传的可能性很高。 问题是,了解与精神分裂症相关基因的关系及其所负责的表型。 了解这种关系可以阐明有关疾病发展和病理生理的更多信息。[4]
If a gene that is a part of a biochemical process is associated with a polygenetic disease like schizophrenia, it suggests that the process is related to the underlying pathology of the disease. If this associated was not previously known, It can then be a new focus of study in the field, which could uncover more information about the pathology of the disease. Machine learning is being applied to polygenetic diseases like schizophrenia for this purpose.
如果属于生化过程的基因与精神分裂症等多基因疾病有关,则表明该过程与疾病的潜在病理学有关。 如果以前不知道这种关联,那么它将成为该领域的新研究重点,从而可能发现有关该疾病病理的更多信息。 为此,机器学习已应用于诸如精神分裂症的多基因疾病。
In one paper, Bern M et al. used machine learning first to assess the importance of some schizophrenic genes in causing the disease. They then compared those significant genes to find those which had a significant role in cell motility, a phenotype they hypothesised was associated with schizophrenia.[4]
在一篇论文中,Bern M等人。 首先使用机器学习来评估某些精神分裂症基因在引起疾病中的重要性。 然后,他们比较了那些重要的基因,以发现在细胞运动中具有重要作用的基因,他们假设的表型与精神分裂症有关。[4]
They then identified 6 genes in which the association was the strongest with the intention of studying their effect on the cell, the results of which could be used to further develop our understanding of cell motility’s relationship with schizophrenia.[4]
然后,他们确定了6个关联最强的基因,旨在研究它们对细胞的作用,其结果可用于进一步发展我们对细胞运动与精神分裂症关系的理解。[4]
However, this technique has not been universally successful. A paper by Zheutlin et al compared machine learning with standard linear regression models to see whether they could predict phenotypes based on 77 genotypes that were known to be associated with schizophrenia. What they found was no comparable difference, with evidence that the machine learning algorithm was task specific; it was using features unique to the data set to predict phenotype, rather than actual differences in genotype.[14] This is significant, as it impacts the generalisability of the algorithm in wider research.
但是,该技术尚未普遍成功。 Zheutlin等人的论文将机器学习与标准线性回归模型进行了比较,以了解它们是否可以基于已知与精神分裂症相关的77个基因型来预测表型。 他们发现的结果没有可比的差异,有证据表明机器学习算法是特定于任务的。 它使用数据集特有的功能来预测表型,而不是基因型的实际差异。[14] 这很重要,因为它在更广泛的研究中影响了该算法的通用性。
However, the two results may not be mutually exclusive. The paper by Bern M et al used a pre-made algorithm called SINKSOURCE, which had been used successfully in a previous study[4]. The paper by Zheutlin et al used random forest modelling. [14]
但是,两个结果可能并不互斥。 Bern M等人的论文使用了一种称为SINKSOURCE的预制算法,该算法已在先前的研究中成功使用[4]。 Zheutlin等人的论文使用了随机森林模型。 [14]
The significance of this is that different machine learning algorithms may vary in terms of their reliability, so more research needs to be done to distinguish algorithms that are generalizable in a clinical context. We saw this when looking at the paper by Acharya UR[11], which showed that different AI algorithms (in that case, neural networks and SVM ML) can produce different accuracies.
这样做的意义在于,不同的机器学习算法在可靠性方面可能会有所不同,因此需要做更多的研究来区分可在临床上推广的算法。 我们在看Acharya UR [11]的论文时就看到了这一点,该论文表明不同的AI算法(在这种情况下,是神经网络和SVM ML)可以产生不同的精度。
预测 (Prediction)
800, 000 people die of suicide every year all around the world. According to the Office for National Statistics 2018, there were 6507 suicides in the UK, the equivalent of 11.2 deaths per 100,000 people. [15]
全世界每年有800,000人死于自杀。 根据国家统计局2018年的数据,英国有6507例自杀,相当于每100,000人中11.2例死亡。 [15]
It is one of the most severe outcomes for both schizophrenia and depression. According to a systematic review on risk factors for suicidal thoughts, depression was the third most common cause of suicidal ideation[6]. Suicide is also serious consequence of schizophrenia. Around 10% of patients with schizophrenia will go on to commit suicide.[2]
它是精神分裂症和抑郁症最严重的后果之一。 根据对自杀念头危险因素的系统评价,抑郁是自杀念头的第三大最常见原因[6]。 自杀也是精神分裂症的严重后果。 大约10%的精神分裂症患者会自杀。[2]
The main application of machine learning in suicide prevention is predicting risk. Its able to take into account different risk factors at once. Most current techniques of suicide risk evaluation includes looking at risk factors in isolation. Machine learning can take into account data on multiple risk factors of an individual and use that information to determine a risk algorithm.
机器学习在自杀预防中的主要应用是预测风险。 它能够同时考虑不同的风险因素。 当前,自杀风险评估的大多数技术都包括单独研究风险因素。 机器学习可以考虑有关个人多个风险因素的数据,并使用该信息来确定风险算法。
TO achieve this, some researchers used data from electronic health records to generate machine learning algorithms that could predict whether a patient was at risk of a suicide attempt. Machine learning was applied to electronic health records of 5167 patients, of which 3250 had evidence of a suicide attempt. They looked at the algorithm’s ability to predict an attempt by using the data at different time intervals between 7 and 720 days before it.[5]
为了实现这一目标,一些研究人员使用电子健康记录中的数据生成了机器学习算法,可以预测患者是否有自杀未遂的风险。 将机器学习应用于5167名患者的电子健康记录,其中3250名有自杀企图。 他们研究了算法通过在数据出现前7到720天之间的不同时间间隔使用数据来预测尝试的能力。[5]
The results showed the algorithm was able to successfully predict whether a patient would go on to attempt suicide. The algorithm, for all time intervals in between 7 days and 2 years before the attempt had an AUC that never fell below 0.8, indicating the classification was significant.[5]
结果表明,该算法能够成功预测患者是否会继续自杀。 该算法在尝试前7天到2年之间的所有时间间隔内的AUC从未低于0.8,这表明分类是重要的。[5]
An interesting find was that similar to the study that looked at Facebook data[17] to predict depression diagnosis, the algorithm had a higher AUC the closer the data was to the suicide attempt. This is important for a screening program. If this technology is to be implemented, there must be research into developing algorithms that use the same data to predict how close the patient is to an attempt of suicide to be used side by side. This is to determine whether the prediction of the algorithm is accurate and an attempt is likely soon, or whether a prediction is not as accurate, and an attempt is likely far away. The proximity of the event is important for the degree of clinician intervention.
一个有趣的发现是,类似于研究Facebook数据[17]来预测抑郁症诊断的研究,该算法的数据越接近自杀企图,AUC越高。 这对于筛选程序很重要。 如果要实施该技术,则必须研究开发使用相同数据的算法,以预测患者离自杀企图并列的距离有多近。 这是为了确定算法的预测是否准确并且可能很快尝试,或者确定预测不是那么准确并且尝试很远。 事件的临近程度对于临床医生的干预程度很重要。


The study then went on to use a different cohort for the control cases. Whereas for the initial algorithm took into account cases that were classified as attempts but were later found not to be (around 1917 patients), the new control cohort included a random selection of hospital patients (around 12,695 patients). The area under the curve values improved to 0.92 at 7 days before attempt and 0.86 at 720 days. [5]
然后研究继续对对照病例使用不同的队列。 最初的算法考虑了被归类为尝试但后来却没有发现的病例(约1917例患者),而新的对照队列包括随机选择的住院患者(约12,695例患者)。 曲线值下的面积在尝试前7天改善为0.92,在720天改善为0.86。 [5]

The algorithms performance actually improves when using real clinical data indicating its applicability as a potential tool for suicide screening. It proves that the algorithm used in this paper can be generalised to the hospital patient population. Note however that the decrease in accuracy with time before attempt is more marked than with curated data, highlighting the need for a complementary algorithm to assess likely time of event, as mentioned previously.
使用实际临床数据表明该算法可作为自杀筛查的潜在工具时,该算法的性能实际上得到了改善。 证明了本文所使用的算法可以推广到医院患者人群。 但是,请注意,尝试之前的时间精度降低的幅度要远大于策展数据的降低幅度,这突显了需要一种补充算法来评估事件的可能时间,如前所述。
The paper also attempted to use standard statistical analysis measure that only produced an AUC of 0.66 at 7 days and 0.68 at 2 years before attempt.[5] This demonstrates the ability of machine learning to differentiate patients based on a larger number of predictors and produce a more accurate and significant result that what a human can do with statistical analysis tools. It shows that machine learning’s advantage is significant.
该论文还尝试使用标准统计分析方法,该方法仅在尝试前7天产生的AUC为0.66,而两年后产生的AUC为0.68。[5] 这证明了机器学习能够基于大量的预测变量区分患者并产生更准确,更有意义的结果的能力,这是人类可以使用统计分析工具完成的结果。 它表明机器学习的优势是巨大的。
更广泛的讨论 (Wider discussion)
Its clear the type of data used to train these algorithms impacts their accuracy, their generalisability and their stability. We saw when comparing papers by Schyner DM et al and Rozycki et al that accuracy of the algorithm increases when using multiple sites to train the algorithm. If, like in the Schyner DM et al paper, you use a data set with a small sample size, then train and test the algorithm on that data set, you increase the risk of “overfitting”. Overfitting is the phenomena when the algorithm is able to accurately classify data based not on true differences, but rather differences that exist within that data set only. You can spot this when testing the algorithm on data from a separate set. If we want to use algorithms in clinical practice, those algorithms will have to be trained on data from the patient population.
显然,用于训练这些算法的数据类型会影响其准确性,通用性和稳定性。 当比较Schyner DM等人和Rozycki等人的论文时,我们发现当使用多个站点训练算法时,算法的准确性会提高。 如果像在Schyner DM等人的论文中那样,使用样本量较小的数据集,然后对该数据集进行训练和测试算法,则会增加“过度拟合”的风险。 当算法能够基于真实差异而不是仅基于该数据集中存在的差异对数据进行准确分类时,就会出现过度拟合现象。 在对来自单独集合的数据进行算法测试时,可以发现这一点。 如果我们想在临床实践中使用算法,则必须对这些算法进行患者群体数据的培训。
Unlike in other fields of medicine, no studies have been undertaken in mental health comparing the efficacy of algorithms against consultants. There is extensive evidence in other specialities of medicine where the efficacy of AI diagnostics is compared to clinicians in real time.
与其他医学领域不同,没有进行过心理健康方面的研究来比较算法与顾问的功效。 在医学的其他专业领域,有广泛的证据可以将AI诊断的功效与临床医生进行实时比较。

No studies with such direct comparison exists in mental health. As we’ve seen when discussing depression diagnostics, there is very little research on the effectiveness of psychiatric diagnosis or prediction. To be confident AI-assisted diagnostics and prediction is safe and effective, future studies should look into direct comparisons with psychiatrists.
在心理健康方面尚无可直接比较的研究。 正如我们在讨论抑郁症诊断时所看到的,关于精神病学诊断或预测有效性的研究很少。 为了确信AI辅助的诊断和预测是安全有效的,未来的研究应考虑与精神科医生进行直接比较。
结论 (Conclusion)
Its clear that the focus in AI-assisted psychiatry is assessing the performance of these algorithms against clinicians in real time. What clear, based on the effectiveness of the algorithms discussed, is that there is promise in using AI in the diagnosis, research and prediction of mental health conditions
很明显,人工智能辅助精神病学的重点是实时评估这些算法对临床医生的性能。 基于所讨论算法的有效性,显而易见的是,使用AI进行精神健康状况的诊断,研究和预测是有希望的
We’ve seen its current application in breaking the psychiatric research barrier, which appear to be the only aspect of psychiatry that AI is currently in application. We may be able to elucidate the pathology of mental health diseases like depression and schizophrenia from gross anatomy, or from molecular biology and genetics.
我们已经看到了其当前在突破精神病学研究障碍方面的应用,这似乎是AI当前正在应用的精神病学的唯一方面。 我们也许能够从整体解剖学或从分子生物学和遗传学来阐明诸如抑郁症和精神分裂症等心理健康疾病的病理。
Its likely we will also see AI assisted EEG diagnosis of both depression and schizophrenia, whereas the future of MRI diagnostics seems less certain. Although, its important to highlight that MRI imaging, especially modalities that assess connectivity and function, have been applied successfully to research.
很有可能我们还将看到AI有助于抑郁症和精神分裂症的脑电图诊断,而MRI诊断的未来似乎不确定。 虽然,重要的是要强调MRI成像,尤其是评估连通性和功能的方法已成功地应用于研究。
The implications for a suicide prediction algorithm extends beyond the mental health clinic. It could be used in workplaces and school by staff who may not be trained to spot those at risk. It likely such an algorithm will be invaluable not just in healthcare, but also outside of it.
自杀预测算法的含义超出了精神卫生诊所的范围。 未经培训的工作人员可以在工作场所和学校中使用它来发现危险人群。 这样的算法可能不仅在医疗保健领域而且在其外部都将是无价的。
IN summation, AI-assisted psychiatry is showing significant promise, but before its implementation in current practice, more research is in the field is needed.
总而言之,人工智能辅助的精神病学显示出巨大的希望,但是在将其应用于当前实践之前,需要在该领域进行更多的研究。
参考书目 (Bibliography)
1. World Health Organisation. Suicide Prevent. Available at: https://www.who.int/mental_health/prevention/suicide/suicideprevent/en/. Accessed March 8th, 2020
1.世界卫生组织。 预防自杀。 可在以下网址获取: https : //www.who.int/mental_health/prevention/suicide/suicideprevent/en/ 。 于2020年3月8日访问
2. Marder SR, Cannon TD. Schizophrenia. New England Journal of Medicine 2019 Oct 31,;381(18):1753–1761.
2. Marder SR,Cannon TD。 精神分裂症。 《新英格兰医学杂志》 2019年10月31日;; 381(18):1753–1761。
3. Drysdale AT, Grosenick L, Downar J, Dunlop K, Mansouri F, Meng Y, et al. Resting-state connectivity biomarkers define neurophysiological subtypes of depression. Nature medicine 2017 Jan;23(1):28–38.
3. Drysdale AT,Grosenick L,Downar J,Dunlop K,Mansouri F,Meng Y等。 静止状态连通性生物标志物定义了抑郁症的神经生理亚型。 自然医学2017年1月; 23(1):28–38。
4. Bern M, King A, Applewhite DA, Ritz A. Network-based prediction of polygenic disease genes involved in cell motility. BMC bioinformatics 2019 Jun 20,;20(Suppl 12):313.
4. Bern M,国王A,Applewhite DA,RitzA。与细胞运动有关的多基因疾病基因的基于网络的预测。 BMC生物信息学2019年6月20日;; 20(增刊12):313。
5. Walsh CG, Ribeiro JD, Franklin JC. Predicting Risk of Suicide Attempts Over Time Through Machine Learning. Clinical Psychological Science 2017 May;5(3):457–469.
5. Walsh CG,Ribeiro JD,Franklin JC。 通过机器学习预测一段时间内自杀未遂的风险。 临床心理科学,2017年5月; 5(3):457–469。
6. Franklin JC, Ribeiro JD, Fox KR, Bentley KH, Kleiman EM, Huang X, et al. Risk factors for suicidal thoughts and behaviors: A meta-analysis of 50 years of research. Psychological bulletin 2017 Feb;143(2):187–232.
6. Franklin JC,Ribeiro JD,Fox KR,Bentley KH,Kleiman EM,Huang X等。 自杀思想和行为的危险因素:对50年研究的荟萃分析。 心理公告2017年2月; 143(2):187-232。
7. Schnyer DM, Clasen PC, Gonzalez C, Beevers CG. Evaluating the diagnostic utility of applying a machine learning algorithm to diffusion tensor MRI measures in individuals with major depressive disorder. Psychiatry Research: Neuroimaging 2017;264:1–9.
7. Schnyer DM,Clasen PC,Gonzalez C和Beevers CG。 在重度抑郁症患者中评估将机器学习算法应用于扩散张量MRI测量的诊断效用。 精神病学研究:Neuroimaging 2017; 264:1–9。
8. Mitchell AJ, Vaze A, Rao S. Clinical diagnosis of depression in primary care: a meta-analysis. 2009.
8. Mitchell AJ,Vaze A,Rao S.初级保健中抑郁症的临床诊断:一项荟萃分析。 2009年。
9. Rozycki M, Satterthwaite TD, Koutsouleris N, Erus G, Doshi J, Wolf DH, et al. Multisite Machine Learning Analysis Provides a Robust Structural Imaging Signature of Schizophrenia Detectable Across Diverse Patient Populations and Within Individuals. Schizophrenia Bulletin 2018 Aug 20,;44(5):1035–1044.
9. Rozycki M,Satterthwaite TD,Koutsouleris N,Erus G,Doshi J,Wolf DH等。 多站点机器学习分析提供了跨不同患者群体和个人内部可检测到的精神分裂症的强大结构成像特征。 精神分裂症通报,2018年8月20日;; 44(5):1035–1044。
10. Tran BX, McIntyre RS, Latkin CA, Phan HT, Vu GT, Nguyen HLT, et al. The Current Research Landscape on the Artificial Intelligence Application in the Management of Depressive Disorders: A Bibliometric Analysis. International journal of environmental research and public health 2019 Jun 18,;16(12):2150.
10. Tran BX,McIntyre RS,Latkin CA,Phan HT,Vu GT,Nguyen HLT等。 人工智能在抑郁症管理中的应用研究现状:文献计量分析。 国际环境研究与公共卫生杂志2019 Jun 18,; 16(12):2150。
11. Acharya UR, Oh SL, Hagiwara Y, Tan JH, Adeli H, Subha DP. Automated EEG-based screening of depression using deep convolutional neural network. Computer Methods and Programs in Biomedicine 2018 Jul;161:103–113.
11. Acharya UR,哦SL,Ha原Y,Tan JH,Adeli H,Subha DP。 使用深度卷积神经网络自动进行基于EEG的抑郁症筛查。 生物医学中的计算机方法和程序2018 Jul; 161:103–113。
12. Shim M, Hwang H, Kim D, Lee S, Im C. Machine-learning-based diagnosis of schizophrenia using combined sensor-level and source-level EEG features. Schizophrenia Research 2016;176(2–3):314–319.
12. Shim M,Hwang H,Kim D,Lee S,ImC。结合传感器级和源级EEG功能基于机器学习的精神分裂症诊断。 精神分裂症研究2016; 176(2-3):314-319。
13. National Institute for Health and Care Excellence. Depression in adults: recognition and management [Internet]. [London]: NICE; 2009 [updated 2016 Apr; cited 2016 Dec 16]. (Clinical guideline [CG90]). Available from: https://www.nice.org.uk/guidance/cg90
13.国家卫生保健卓越研究所。 成人抑郁症:认识和管理[互联网]。 [伦敦]:NICE; 2009 [2016年4月更新; 引用2016年12月16日]。 (临床指南[CG90])。 可从以下网址获得: https : //www.nice.org.uk/guidance/cg90
14. Zheutlin AB, Chekroud AM, Polimanti R, Gelernter J, Sabb FW, Bilder RM, et al. Multivariate Pattern Analysis of Genotype–Phenotype Relationships in Schizophrenia. Schizophrenia Bulletin 2018 Aug 20,;44(5):1045–1052.
14.Zheutlin AB,Chekroud AM,Polimanti R,Geernter J,Sabb FW,Bilder RM等。 精神分裂症基因型-表型关系的多元模式分析。 精神分裂症通报2018年8月20日;; 44(5):1045–1052。
15. Office for National Statistics. Suicides in the UK: 2018 registrations. 2019; Available at: https://www.ons.gov.uk/peoplepopulationandcommunity/birthsdeathsandmarriages/deaths/bulletins/suicidesintheunitedkingdom/2018registrations. Accessed Mar 8, 2020.
15.国家统计局。 英国的自杀:2018年注册。 2019; 网址 : https : //www.ons.gov.uk/peoplepopulationandcommunity/birthsdeathsandmarriages/deaths/bulletins/suicidesintheunitedkingdom/2018registrations 。 于2020年3月8日访问。
16. Eichstaedt JC, Smith RJ, Merchant RM, Ungar LH, Crutchley P, Preoţiuc-Pietro D, et al. Facebook language predicts depression in medical records. Proceedings of the National Academy of Sciences of the United States of America 2018 Oct 30,;115(44):11203–11208.
16. Eichstaedt JC,Smith RJ,商人RM,Ungar LH,Crutchley P,Preoţiuc-PietroD等。 Facebook语言可预测病历下降。 美国国家科学院院刊2018年10月30日;; 115(44):11203–11208。
17. Steinhubl SR, Muse ED, Topol EJ. The emerging field of mobile health. Science translational medicine 2015 Apr 15,;7(283):283rv3.
17. Steinhubl SR,Muse ED,白杨EJ。 移动医疗的新兴领域。 科学转化医学2015年4月15日;; 7(283):283rv3。
翻译自: https://medium.com/@aizazatachaudhry/my-beginning-into-machine-learning-b6e985b5f627
机器学习 开始