深入研究数据库该怎么做
Metadata hubs (or sometimes known as metadata search & discovery tools) seems to be another trend/movement that’s happening in the analytics space. In the past two years alone, we’ve seen a whole host of metadata hub projects being released, written about, or open sourced by major tech companies. These include:
元数据中心(或有时称为元数据搜索和发现工具)似乎是分析空间中正在发生的另一种趋势/运动。 仅在过去的两年中,我们就看到了由主要技术公司发布,编写或开源的大量元数据中心项目。 这些包括:
Airbnb’s Dataportal
Airbnb的数据门户
Netflix’s Metacat
Netflix的Metacat
Uber’s Databook
优步的数据手册
LinkedIn’s Datahub
Lyft’s Amundsen
莱夫特的阿蒙森
Spotify’s Lexikon
Spotify的Lexikon
and Google Cloud Platform’s Data Catalog (though I’m only including this because it seems like a response to the current trend of metadata hubs in the market).
和Google Cloud Platform的数据目录(尽管我只包括了它,因为这似乎是对市场中元数据中心当前趋势的回应)。
In this post, I’ll quickly summarise the problem these products are created to solve, give a quick overview of each of the projects, and then drill down on what seems like the two most promising ones: LinkedIn’s Datahub, and Lyft’s Amundsen.
在这篇文章中,我将快速总结这些产品要解决的问题,快速概述每个项目,然后深入研究似乎最有前途的两个项目:LinkedIn的Datahub和Lyft的Amundsen。
什么是元数据中心? (What is a Metadata Hub?)
Imagine that you’re a data user or a data analyst in a large company, with many offices around the globe. Your data organization is spread out across those locations, and you have thousands of tables in different data warehouses and BI tools, plus hundreds of reports, datasets, and data models in varying stages of analysis spread across your entire company. You need to find a report that you need, or a dataset that you want. If you can’t find those things, then you need to find the next best thing: you need to know who to ask, or which team in the organization to direct your query to.
想象一下,您是一家大型公司的数据用户或数据分析师,而该公司在全球各地都有许多办事处。 您的数据组织分布在这些位置,您在不同的数据仓库和BI工具中有成千上万个表,并且在整个公司的不同分析阶段中有数百个报告,数据集和数据模型。 您需要找到所需的报告或所需的数据集。 如果找不到这些东西,那么您需要找到下一个最好的东西:您需要知道向谁询问,或者组织中的哪个团队将您的查询定向到。
Enter the metadata hub.
输入元数据中心。
The goal of the metadata hub is to act as a ‘search engine’ for the entire data ecosystem within your company. The ‘metadata’ in the name means ‘data about the data’. This includes information like your table schema, your column descriptions, the access records and SLAs associated with each database or data warehouse, and also things like “which team is responsible for this dataset” and “who was the last person to have created a report from it?”
元数据中心的目标是充当公司内整个数据生态系统的“搜索引擎”。 名称中的“元数据”表示“有关数据的数据”。 这包括诸如表架构,列说明,与每个数据库或数据仓库关联的访问记录和SLA之类的信息,以及“由哪个团队负责此数据集”和“谁是最后一个创建报告的人”之类的信息。从中?”
Of course, as Mars Lan of LinkedIn’s DataHub puts it: “if you are able to find out where everything is by asking one person in your company, then you definitely don’t need this.” Metadata hubs only become important when you are a large organization, and you need to scale data access across that entire organization. You’ll hear words like ‘data democratization’ and ‘data discoverability’ being thrown around a lot; what they’re really referring to is the ability for a random data analyst to keep up with the rest of a very large data-producing and data-consuming organization.
当然,作为LinkedIn的DataHub的火星兰所说的那样:“如果你能找到这里的一切是问一个人在你的公司,那么你肯定不需要这个” 仅当您是大型组织时,元数据中心才变得重要,并且您需要在整个组织中扩展数据访问。 您会听到很多类似“数据民主化”和“数据可发现性”的词; 他们真正指的是随机数据分析师能够跟上非常庞大的数据产生和数据消耗组织的其余部分的能力。
Metadata hubs only become important when you are a large organization, and you need to scale data access across that entire organization.
仅当您是大型组织时,元数据中心才变得重要,并且您需要在整个组织中扩展数据访问。
Broadly speaking, all of the metadata hub projects I’ve mentioned above provide some combination of the following:
从广义上讲,我上面提到的所有元数据中心项目都提供以下内容的组合:
Search: A search interface for end-users.
搜索:最终用户的搜索界面。
Discovery: An explorable interface for users to browse and navigate different data sources, and drill down to the table or row level.
发现:用户可浏览的界面,用于浏览和导航不同的数据源,并向下钻取到表或行级别。
Automation: An API service that exposes metadata for other internal data services to consume.
自动化:一种API服务,公开元数据供其他内部数据服务使用。
Curation: Some ability to select, save, and curate collections of metadata.
策展:选择,保存和策划元数据集合的某种能力。
Certain projects, like Airbnb’s Dataportal and Spotify’s Lexikon, are able to expose user profiles, so that you may find individuals or teams who have last touched the dataset you are interested in. But others do not.
某些项目(例如Airbnb的Dataportal和Spotify的Lexikon)能够公开用户个人资料,以便您可以找到上次接触您感兴趣的数据集的个人或团队,而其他项目则没有。
简短的项目 (The Projects, Briefly)
I’m going to go over the projects in chronological order, and give you enough outbound links to go and chase down the original announcement posts, repositories (if available), and tech talks, if you wish. But if you want to get to the good bits, I’d recommend skimming this section (paying special attention to the bits about Amundsen and Datahub) and then skip forward to the next section, where I compare the two projects. Those two seem to have the most community activity around them right now.
我将按时间顺序浏览这些项目,并为您提供足够的出站链接,以便按您的意愿追踪原始的公告帖子,存储库(如果有)和技术讲座。 但是,如果您想精通本章,我建议略过本节(特别注意有关Amundsen和Datahub的内容),然后跳到下一部分,在此我比较两个项目。 目前,这两个人似乎是他们周围社区活动最多的人。
Airbnb的数据门户,2017年5月11日 (Airbnb’s Dataportal, 11 May 2017)
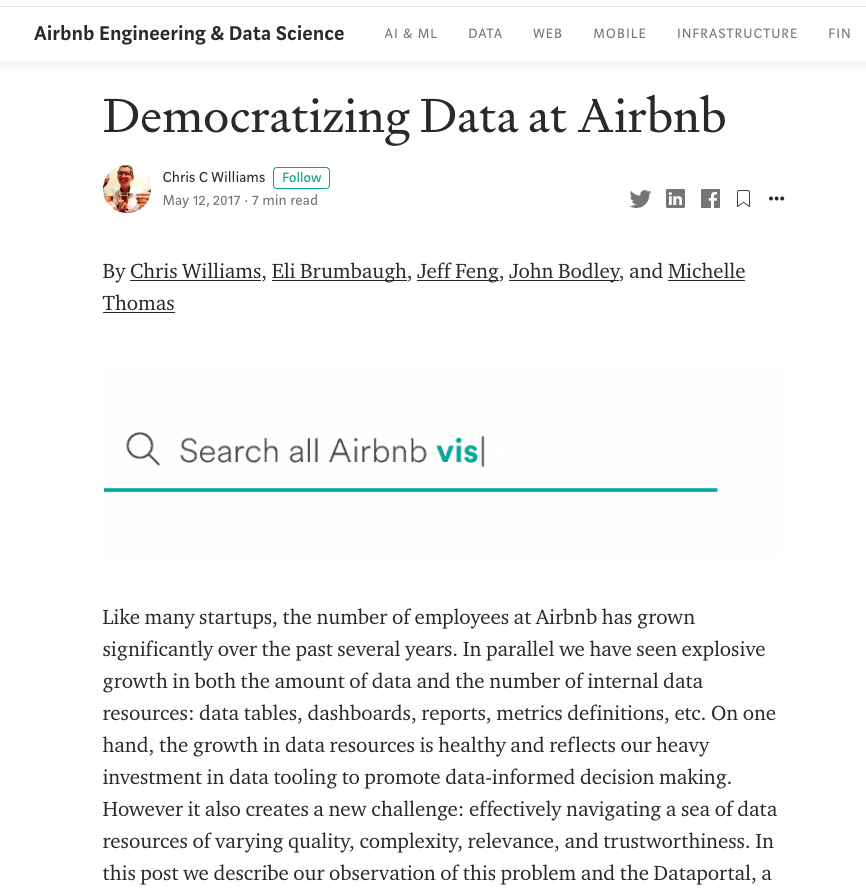
The earliest project in this list is Airbnb’s Dataportal. Dataportal was first described on stage by Chris Williams and John Bodley at GraphConnect Europe 2017 (slides available here). They then published a blog post a day later, on May 12th 2017, describing the system.
此列表中最早的项目是Airbnb的Dataportal。 克里斯·威廉姆斯(Chris Williams)和约翰·博德利(John Bodley)在GraphConnect Europe 2017上首次在舞台上描述了数据端口(可在此处找到幻灯片)。 然后,他们在一天后的2017年5月12日发表了一篇博客文章,描述了该系统。
Dataportal kept track of who produced or consumed data resources within Airbnb. The project modelled these relationships as a graph in neo4j, which then meant that they could provide a search engine, using the Pagerank algorithm, to surface those connections to users.
Dataportal跟踪谁在Airbnb中生产或消费了数据资源。 该项目将这些关系建模为neo4j中的图形,这意味着它们可以使用Pagerank算法提供搜索引擎,以向用户显示这些连接。
Dataportal was never open-sourced, and interestingly enough, doesn’t show up on https://airbnb.io/projects/. We haven’t heard about it since.
Dataportal从未开源,而且有趣的是,它没有出现在https://airbnb.io/projects/上。 此后我们再也没有听说过。
Netflix的Metacat,2018年6月15日 (Netflix’s Metacat, 15 Jun 2018)
About a year later, Netflix published a blog post describing their metadata system, named Metacat. This project was built to plug a hole in Netflix’s data stack — essentially, they needed a metadata service to sit between their Pig ETL system and Hive.
大约一年后,Netflix发布了一篇描述其元数据系统的博客文章,名为Metacat。 该项目旨在填补Netflix数据堆栈中的一个漏洞-本质上,他们需要元数据服务才能位于其Pig ETL系统和Hive之间。
The Metacat system lives as a federated service within Netflix’s data infrastructure. It provides a unified API to access metadata of various data stores within Netflix’s internal ecosystem, but it is highly customised to Netflix’s own tools and data stack. It doesn’t seem particularly extensible.
Metacat系统作为Netflix数据基础架构中的联合服务而存在。 它提供了一个统一的API,可以访问Netflix内部生态系统中各种数据存储的元数据,但是它是针对Netflix自己的工具和数据堆栈而高度定制的。 它似乎不是特别可扩展。
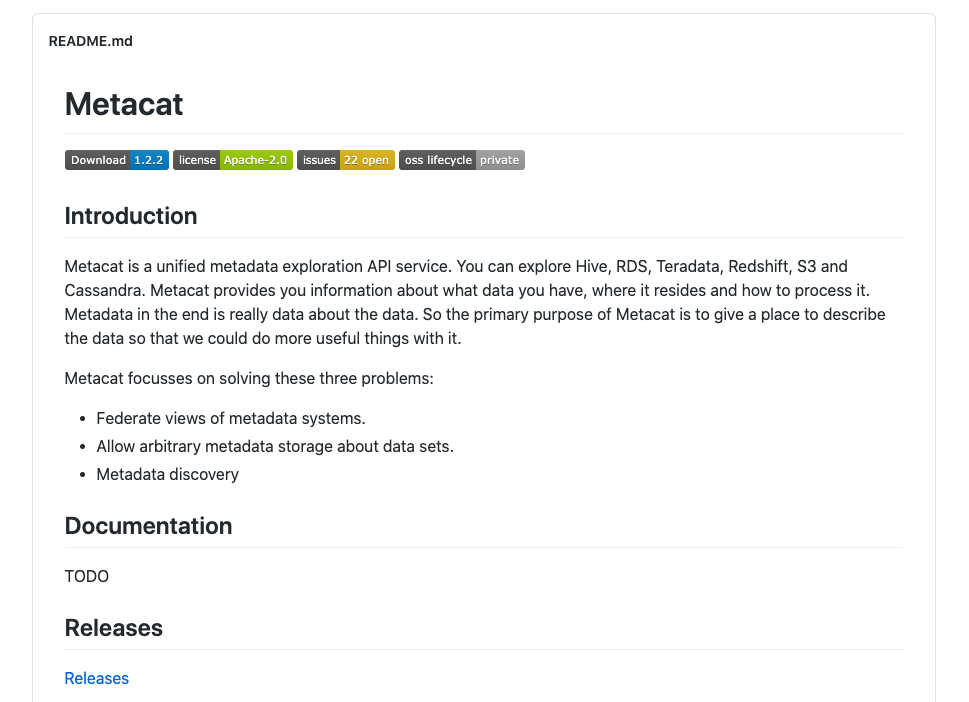
Metacat is open-sourced and being actively developed, but not designed for serious external adoption, it seems. As of writing, their Github readme contains a section titled ‘Documentation’ with the words ‘TODO’ written underneath.
看起来,Metacat是开源的,并且正在积极开发中,但是它并不是为严重的外部采用而设计的。 在撰写本文时,他们的Github自述文件包含标题为“文档”的部分,其下方写有“ TODO”字样。
优步数据手册,2018年8月3日 (Uber’s Databook, 3 Aug 2018)
Uber’s Databook was announced in a blog post a month or so after Netflix’s Metacat announcement.
Netflix的Metacat发布后一个月左右,Uber的Databook在博客中发布。
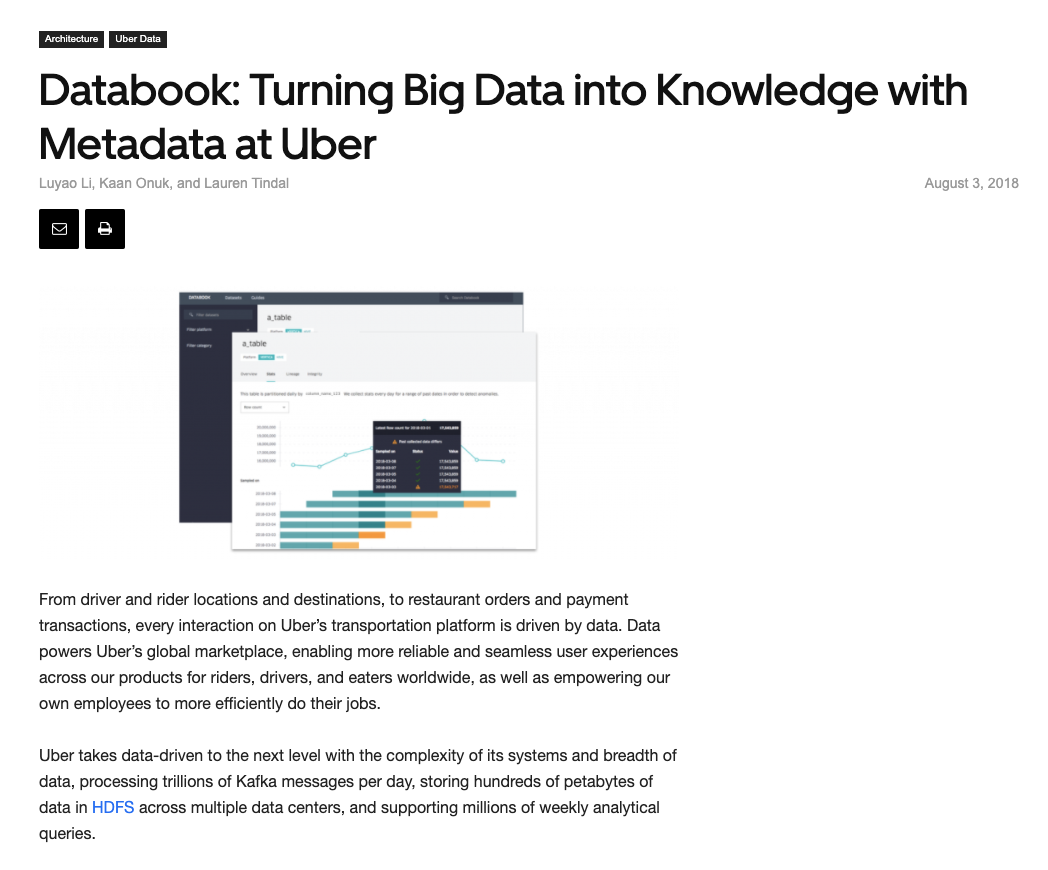
Databook grabs metadata from Hive, Vertica, MySQL, Postgres and Cassandra, and exposes things like table schemas, column descriptions, sample data from the databases, statistics, lineage, freshness, SLA guarantees and data owners, all in one interface. It also allows Databook users to organize and curate these pieces of metadata within their user interface. In the early stages, Databook was built on a crawler architecture (that is, Databook would run crawlers to go out and collect metadata from the various data sources), but as time passed, Uber’s data engineering team eventually switched the system to one built on top of Kafka. They also switched from MySQL to Cassandra in order to support multiple data centres without suffering from increased latency.
数据手册从Hive,Vertica,MySQL,Postgres和Cassandra获取元数据,并在一个界面中公开表架构,列说明,数据库中的示例数据,统计信息,沿袭,新鲜度,SLA保证和数据所有者等内容。 它还允许Databook用户在其用户界面内组织和管理这些元数据。 在早期阶段,Databook是建立在搜寻器架构上的(也就是说,Databook将运行搜寻器以出去并从各种数据源中收集元数据),但是随着时间的流逝,Uber的数据工程团队最终将系统切换为基于卡夫卡的顶部。 他们还从MySQL切换到Cassandra,以支持多个数据中心而不会增加延迟。
Databook is not open sourced, and we haven’t heard about it in the years since. Presumably, it remains widely used within Uber.
Databook不是开源的,从那以后我们还没有听说过。 据推测,它在Uber中仍被广泛使用。
Lyft的Amundsen,2019年8月3日 (Lyft’s Amundsen, 3 Aug 2019)
Lyft’s Amundsen was announced on the 3rd of August, 2019, and then open sourced a few months later, on the 31st of October the same year.
Lyft的Amundsen于2019年8月3日宣布,然后在几个月后的同年10月31日开源。
Amundsen generated some serious buzz when it came out. Tristan Handy of dbt wrote, of Amundsen’s open sourcing:
阿蒙森(Amundsen)出来时引起了严重的嗡嗡声。 dbt的Tristan Handy写道,Amundsen的开源:
This product is important, and I really believe the product category is going to be potentially the single most important product category in data in the coming five years. Everything is going to need to feed the catalog.
该产品非常重要,我真的相信,在未来五年中,产品类别可能会成为数据中最重要的单一产品类别。 一切都需要提供目录。
Amundsen has traces of past systems in its DNA. Like Dataportal, it allows you to search for data resources within your company, and it exposes a discoverable interface down to the row level for each table in each data source it has access to. At launch, the creators also talked about how they intended to integrate with tools like Workday, in order to expose employee information within Amundsen’s search graph, so that you could reach out to fellow employees who had context around the datasets you were interested in.
阿蒙森(Amundsen)在其DNA中拥有过去系统的痕迹。 与Dataportal一样,它允许您在公司内部搜索数据资源,并且为它有权访问的每个数据源中的每个表公开一个可发现的接口,直到行级。 在发布时,创建者还讨论了他们打算如何与Workday之类的工具集成,以便在Amundsen的搜索图中显示员工信息,以便您可以与对您感兴趣的数据集有上下文的其他员工接触。
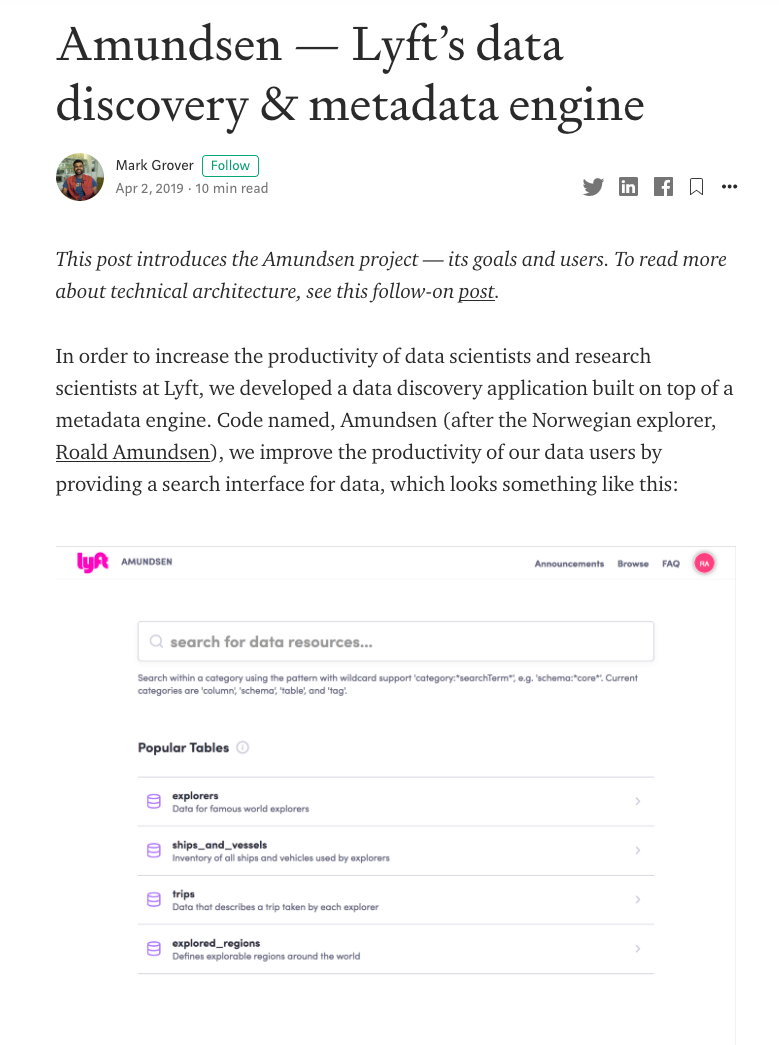
Amundsen consists of a number of different components (source):
Amundsen由许多不同的组件组成(来源):
The Metadata Service handles metadata requests from the front-end service as well as other micro services. By default the persistent layer is Neo4j.
元数据服务处理来自前端服务以及其他微服务的元数据请求。 默认情况下,持久层是Neo4j。
The Search Service is backed by Elasticsearch to handle search requests from the front-end service. This search engine may also be substituted.
Elastic Search支持Search Service ,以处理来自前端服务的搜索请求。 该搜索引擎也可以被替换。
The Front-End Service is a Flask webapp, hosting a React frontend. This is the primary interface that users interact with.
前端服务是一个Flask Web应用程序,托管一个React前端。 这是用户与之交互的主要界面。
Databuilder is a generic data ingestion framework that extracts metadata from various sources. It was inspired by Apache Gobblin, and serves as an ETL tool for shoving metadata into Amundsen’s metadata service. It uses Airflow as a workflow tool.
Databuilder是一个通用的数据提取框架,可从各种来源提取元数据。 它受Apache Gobblin的启发,并用作将元数据推入Amundsen的元数据服务的ETL工具。 它使用Airflow作为工作流工具。
And finally, Common is a library repo which holds common code among all micro services in Amundsen.
最后, Common是一个库存储库,它在Amundsen的所有微服务中保存通用代码。
In Amundsen’s launch blog post, the authors note that it has driven down the time to discover a data artefact to 5% of the pre-Amundsen baseline. Amundsen’s community is probably the largest of all the projects covered here, and it is still rapidly growing today.
在Amundsen的启动博客文章中,作者指出,这已将发现数据伪像的时间缩短到了Amundsen之前基线的5%。 阿蒙森(Amundsen)的社区可能是此处涵盖的所有项目中最大的社区,并且今天它仍在快速增长。
LinkedIn的Datahub,2019年8月14日 (LinkedIn’s Datahub, 14 Aug 2019)
Announced less than two weeks after Amundsen, LinkedIn’s Datahub is actually their second attempt at building a metadata hub. In 2016, LinkedIn open sourced WhereHow, which influenced some of the other projects mentioned in this post. But Datahub was written from scratch to resolve some of the problems LinkedIn experienced with WhereHow.
宣布阿蒙森后不到两个星期,LinkedIn的Datahub实际上是他们在构建元数据中心的第二次尝试。 2016年,LinkedIn开源了WhereHow ,这影响了本文中提到的其他一些项目。 但是Datahub是从零开始编写的,旨在解决LinkedIn在WhereHow中遇到的一些问题。
Most intriguingly, Datahub is built on top of a ‘push-based’ architecture. This means that every data service in one’s organization must be modified to push metadata to Datahub, instead of having Datahub scrape the data from the services. In practice, this is not too difficult to do, since Datahub is built on top of Kafka streams, and Kafka is already widely used within LinkedIn (Kafka was, ahem, created there).
最有趣的是,Datahub建立在“基于推送”的架构之上。 这意味着必须修改组织中的每个数据服务,以将元数据推送到Datahub,而不是让Datahub从服务中抓取数据。 实际上,这并不是一件容易的事,因为Datahub是建立在Kafka流之上的,并且Kafka已经在LinkedIn中被广泛使用(Kafka是ahem所创建的)。
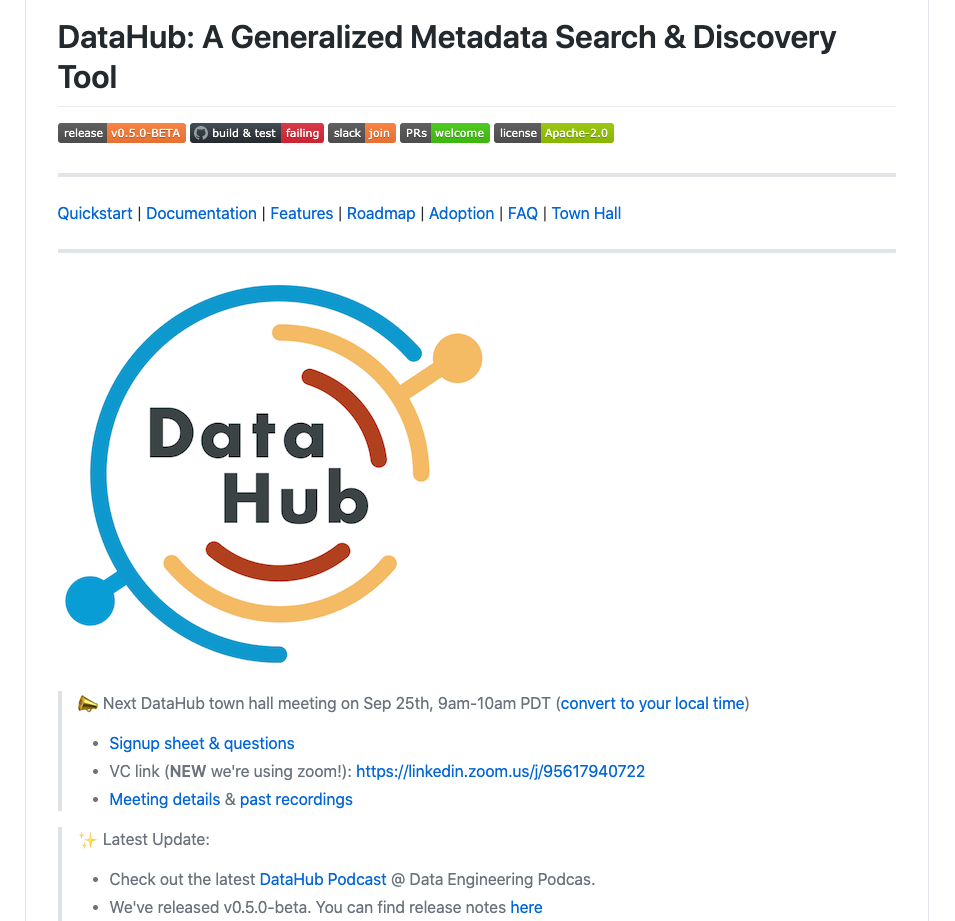
Datahub’s architecture results in a number of interesting benefits. First, it allows incremental ingestion (instead of scraping or snapshotting once every time period). Second, it allows for near-real-time updates, since any change that gets pushed to Datahub will immediately be passed on to downstream consumers. Mars Lan, one of the creators, notes (podcast; around 18:20) that this setup allows you to then build applications that consume the changestream emitted from Datahub, and that they have done this extensively within LinkedIn.
Datahub的体系结构带来了许多有趣的好处。 首先,它允许增量摄取(而不是每个时间段一次抓取或快照)。 其次,它允许近实时更新,因为任何推送到Datahub的更改都将立即传递给下游使用者。 创建者之一Mars Lan注意到(播客;大约18:20 ),此设置使您可以构建使用Datahub发出的变更流的应用程序,并且他们已在LinkedIn中进行了广泛的操作。
In fact, the entire podcast episode is worth listening to. Lan talks about some of the tradeoffs they needed to make when coming up with an extensible but general data model in Datahub (“you know you’ve succeeded when people spend 90% of their time debating how to model the metadata correctly, and then spend 10% of their time actually coding it.”). It gives you a taste of the depth of experience the engineers brought to the project.
实际上,整个播客都值得一听。 Lan谈到了在Datahub中提出可扩展但通用的数据模型时需要做出的一些权衡取舍(“您知道当人们花费90%的时间讨论如何正确地对元数据建模然后再花费时,您就成功了。他们有10%的时间实际在编码它。”)。 它使您领略到工程师带给该项目的丰富经验。
Datahub was open sourced in February this year. More about this in a bit.
Datahub于今年2月开源。 进一步了解这个。
Spotify的Lexikon,2020年2月27日(尽管建于2017年) (Spotify’s Lexikon, 27 Feb 2020 (though built in 2017))
Spotify’s Lexikon covers much of the same ground as previous projects. The original version of Lexikon was built in 2017, in response to an explosion of datasets after Spotify’s move to GCP and BigQuery.
Spotify的Lexikon与以前的项目有很多相同的领域。 Lexikon的原始版本建于2017年,以应对Spotify移至GCP和BigQuery之后数据集的激增。
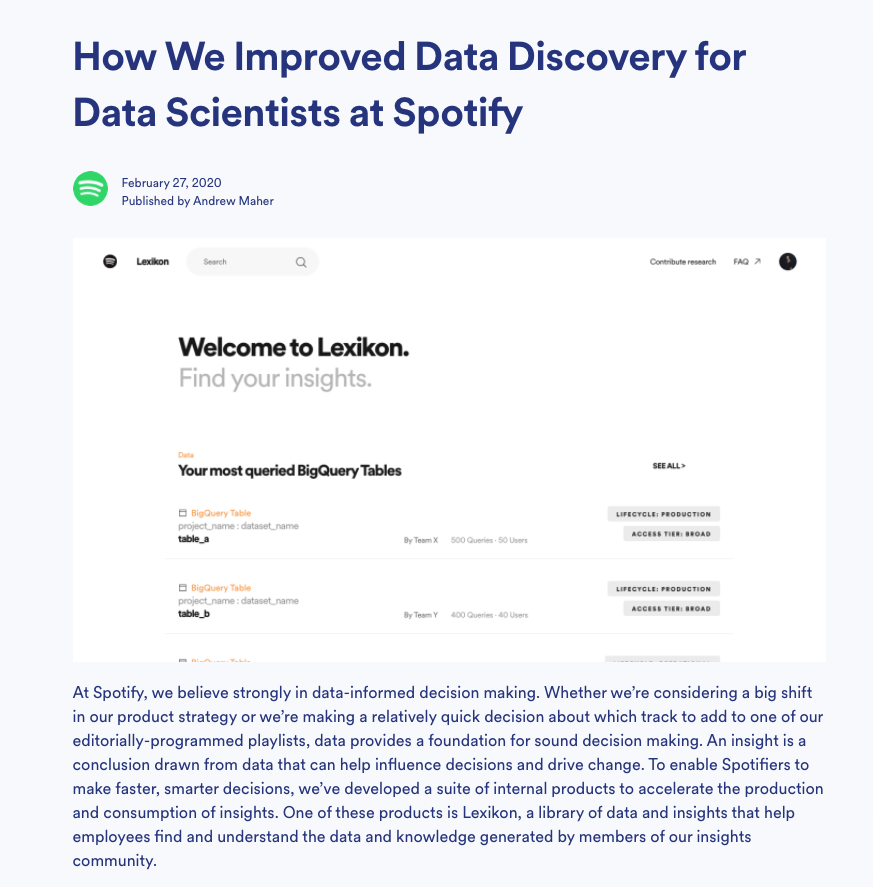
The most novel aspect of the project is that it offers algorithmically generated, personalized suggestions for datasets to data users. (Which is, if you think about it, such a Spotify thing to do). Like Dataportal, Lexikon provides a search experience, and also surfaces users who might help with the dataset you are looking at. Like Amundsen and Datahub, Lexicon shows you table-level and column-level statistics.
该项目最新颖的方面是,它向数据用户提供了算法生成的针对数据集的个性化建议。 (这是,如果您考虑一下,可以执行Spotify这样的操作)。 像Dataportal一样,Lexikon可以提供搜索体验,还可以吸引可能会帮助您查看数据集的用户。 像Amundsen和Datahub一样,Lexicon向您显示表级和列级统计信息。
Lexikon is not open sourced.
Lexikon不是开源的。
Google Cloud的数据目录,2020年5月1日 (Google Cloud’s Data Catalog, 1 May 2020)
Google Cloud’s Data Catalog is a similar metadata service, with the caveat that it only works with GCP services like BigQuery, Pub/Sub, and Cloud Storage filesets. The idea is that you add Data Catalog to an existing GCP-hosted project, flip a switch, and enjoy the benefits of Google’s data catalog by magic.
Google Cloud的数据目录是一种类似的元数据服务,但需要注意的是,它仅适用于GCP服务,例如BigQuery,Pub / Sub和Cloud Storage文件集。 这个想法是,您可以将数据目录添加到现有的GCP托管项目中,进行切换,然后神奇地享受Google数据目录的优势。
I’m only including this project for two reasons. First, Google announced that it had reached general availability on the 1st of May 2020, not too long ago. To an outside observer, it seemed like a response to the current flurry of activity in this space, which bodes well for the overall data ecosystem.
我之所以加入这个项目,是出于两个原因。 首先,谷歌宣布不久之前就已于2020年5月1日实现全面上市。 对于外部观察者来说,这似乎是对当前该领域活动的一种回应,这对于整个数据生态系统而言是个好兆头。
Second, Google says that Data Catalog was built on the insights drawn from their Goods data system, which was (is?) used internally at Google as a very effective data catalog service, for many, many years. The paper describing that system may be found here.
其次,谷歌表示,数据目录是建立在其商品数据系统的洞察力之上的,多年来,谷歌在内部一直将其用作一项非常有效的数据目录服务。 描述该系统的论文可以在这里找到。
观看Amundsen和Datahub (Watch Amundsen and Datahub)
In this group of projects, Amundsen and Datahub appear to be the frontrunners. Amundsen has a buzzing community, a really snazzy website, and a number of interesting case studies: edmunds.com, ING, and Square. Other companies that have adopted Amundsen include Workday, Asana, and iRobot.
在这组项目中,Amundsen和Datahub似乎是领先者。 阿蒙森(Amundsen)拥有一个嗡嗡作响的社区,一个非常时髦的网站以及许多有趣的案例研究: edmunds.com , ING和Square 。 其他采用Amundsen的公司包括Workday,Asana和iRobot。
Amundsen has a bit of a head start on Datahub, since it was open sourced in October last year. The community is larger, and as of August 11th, Amundsen is now a Linux Foundation’s AI Foundation Incubation Project. More importantly, you can see project creator Mark Grover’s ambitions in his statement in the press release:
自从去年10月开源以来,Amundsen在Datahub上就拥有领先优势。 社区更大,截至8月11日,Amundsen现在是Linux基金会的AI基金会孵化项目。 更重要的是,您可以在新闻稿中的声明中看到项目创建者Mark Grover的野心:
“Becoming a part of the LF AI Foundation is a big milestone for the project in its journey towards becoming the de-facto open-source data discovery and metadata engine. It’s been amazing to see the adoption of Amundsen at Lyft, as well the growth of its open source community, which now has over 750 members. I am excited to see the project’s continued growth and success with the support of the LF AI Foundation.”
“成为LF AI Foundation的一部分,对于该项目成为事实上的开源数据发现和元数据引擎的旅程而言,是一个重要的里程碑。 看到Lyft采纳Amundsen以及其开源社区的发展令人惊讶,该社区现在已有750多个成员。 我很高兴看到该项目在LF AI基金会的支持下继续增长并取得了成功。”
On the other hand, Datahub should be taken seriously because of LinkedIn’s track record with open source projects (they created and then open sourced Kafka, which is now a major project in the cloud infrastructure space, and Datahub is built on top of Kafka … making the job of scaling it — in the words of the creators — a ‘solved problem’). According to their Github page, the number of organizations that have adopted Datahub is also pretty impressive: amongst them are companies like Expedia, Saxo Bank, TypeForm, and Experius.
另一方面,由于LinkedIn在开放源代码项目方面的往绩,Datahub应该得到认真对待(他们创建并随后开源了Kafka,Kafka现在是云基础架构领域的重要项目,而Datahub建立在Kafka之上……用创造者的话来说,扩展它的工作是“解决的问题”)。 根据他们在Github上的页面,采用Datahub的组织数量也相当可观:其中包括Expedia,Saxo Bank,TypeForm和Experius等公司。
This is notable because DataHub was only open-sourced six months ago. Mars Lan and Pardhu Gunnam was recently interviewed on the Data Engineering Podcast, and they note that the speed of adoption and the growing community has taken them somewhat by surprise. Lan also argued that their approach was superior to everything else on the market, because of hard-won lessons from building WhereHow within LinkedIn more than four years ago (Lan wasn’t involved with WhereHow, it should be noted, but usage of WhereHow has informed the design of Datahub).
值得注意的是,DataHub仅在六个月前才开源。 Mars Lan和Pardhu Gunnam最近在数据工程播客上接受了采访,他们指出,采用的速度和社区的发展使他们有些惊讶。 Lan还认为,他们的方法优于市场上的其他产品,这是因为四年前从LinkedIn中构建WhereHow方面来之不易的经验教训(Lan并未参与WhereHow,应该指出的是,WhereHow的用法已告知Datahub的设计)。
结论 (Conclusion)
As I’m writing this, it’s been slightly more than a year since these projects were publicly announced, and less than a year since open source adoption started, there’s still a long way to go before they hit maturity.
在我撰写本文时,距离这些项目的公开宣布已经过去了一年多的时间,而自从开始采用开源项目不到一年的时间,要使其成熟,还有很长的路要走。
Given these projects are solved very similar problems, backed by strong tech companies, and most of them opted to go with open-source approach, it’ll be interesting to see which ones will come out ahead and what make them do.
鉴于这些项目得到了强大技术公司的支持,解决了非常相似的问题,并且大多数人选择采用开源方法,因此,看看哪些将领先并取得成功将是很有趣的。
翻译自: https://towardsdatascience.com/a-dive-into-metadata-hub-tools-67259804971f
深入研究数据库该怎么做