基于深度学习lstm
Malware development has seen diversity in terms of architecture and features. This advancement in the competencies of malware poses a severe threat and opens new research dimensions in malware detection. This study is focused on metamorphic malware that is the most advanced member of the malware family. It is quite impossible for anti-virus applications using traditional signature-based methods to detect metamorphic malware, which makes it difficult to classify this type of malware accordingly. Recent research literature about malware detection and classification discusses this issue related to malware behaviour.
恶意软件的开发在体系结构和功能方面具有多样性。 恶意软件能力的提高带来了严重威胁,并为恶意软件检测打开了新的研究领域。 这项研究的重点是变形恶意软件,它是恶意软件家族中最先进的成员。 使用传统的基于签名的方法的防病毒应用程序几乎不可能检测到变形的恶意软件,这使得很难对此类恶意软件进行分类。 有关恶意软件检测和分类的最新研究文献讨论了与恶意软件行为有关的问题。
Cite The Work If you find this implementation useful please cite it:
引用工作如果您发现此实现有用,请引用:
@article{10.7717/peerj-cs.285,
title = {Deep learning based Sequential model for malware analysis using Windows exe API Calls},
author = {Catak, Ferhat Ozgur and Yazı, Ahmet Faruk and Elezaj, Ogerta and Ahmed, Javed},
year = 2020,
month = jul,
keywords = {Malware analysis, Sequential models, Network security, Long-short-term memory, Malware dataset},
volume = 6,
pages = {e285},
journal = {PeerJ Computer Science},
issn = {2376-5992},
url = {https://doi.org/10.7717/peerj-cs.285},
doi = {10.7717/peerj-cs.285}
}
You can access the dataset from my My GitHub Repository.
您可以从我的GitHub存储库中访问数据集。
介绍 (Introduction)
Malicious software, commonly known as malware, is any software intentionally designed to cause damage to computer systems and compromise user security. An application or code is considered malware if it secretly acts against the interests of the computer user and performs malicious activities. Malware targets various platforms such as servers, personal computers, mobile phones, and cameras to gain unauthorized access, steal personal data, and disrupt the normal function of the system.
恶意软件(通常称为恶意软件)是故意设计成对计算机系统造成损害并危及用户安全的任何软件。 如果应用程序或代码秘密地危害计算机用户的利益并执行恶意活动,则被认为是恶意软件。 恶意软件针对服务器,个人计算机,移动电话和相机等各种平台,以获取未经授权的访问,窃取个人数据并破坏系统的正常功能。
One approach to deal with malware protection problem is by identifying the malicious software and evaluating its behaviour. Usually, this problem is solved through the analysis of malware behaviour. This field closely follows the model of malicious software family, which also reflects the pattern of malicious behaviour. There are very few studies that have demonstrated the methods of classification according to the malware families.
解决恶意软件保护问题的一种方法是识别恶意软件并评估其行为。 通常,此问题是通过分析恶意软件行为来解决的。 该领域紧密遵循恶意软件家族的模型,它也反映了恶意行为的模式。 很少有研究证明了根据恶意软件家族进行分类的方法。
All operating system API calls made to act by any software show the overall direction of this program. Whether this program is a malware or not can be learned by examining these actions in-depth. If it is malware, then what is its malware family. The malware-made operating system API call is a data attribute, and the sequence in which those API calls are generated is also critical to detect the malware family. Performing specific API calls is a particular order that represents a behaviour. One of the deep learning methods LSTM (long-short term memory) has been commonly used in the processing of such time-sequential data.
由任何软件执行的所有操作系统API调用均显示该程序的总体方向。 可以通过深入检查这些操作来了解该程序是否为恶意软件。 如果是恶意软件,那么其恶意软件家族是什么。 恶意软件进行的操作系统API调用是一个数据属性,并且生成这些API调用的顺序对于检测恶意软件家族也很关键。 执行特定的API调用是代表行为的特定顺序。 深度学习方法之一LSTM(长期短期记忆)已普遍用于处理此类按时间顺序排列的数据。
系统架构 (System Architecture)
This research has two main objectives; first, we created a relevant dataset, and then, using this dataset, we did a comparative study using various machine learning to detect and classify malware automatically based on their types.
这项研究有两个主要目标; 首先,我们创建了一个相关的数据集,然后,使用此数据集,我们进行了一项比较研究,使用各种机器学习来根据恶意软件的类型自动检测和分类恶意软件。
数据集创建 (Dataset Creation)
One of the most important contributions of this work is the new Windows PE Malware API sequence dataset, which contains malware analysis information. There are 7107 malware from different classes in this dataset. The Cuckoo Sandbox application, as explained above, is used to obtain the Windows API call sequences of malicious software, and VirusTotal Service is used to detect the classes of malware.
这项工作最重要的贡献之一就是新的Windows PE恶意软件API序列数据集,其中包含恶意软件分析信息。 该数据集中有7107个来自不同类别的恶意软件。 如上所述,Cuckoo Sandbox应用程序用于获取恶意软件的Windows API调用序列,而VirusTotal Service用于检测恶意软件的类别。
The following figure illustrates the system architecture used to collect the data and to classify them using LSTM algorithms.
下图说明了用于收集数据并使用LSTM算法对其进行分类的系统体系结构。
Our system consists of three main parts, data collection, data pre-processing and analyses, and data classification.
我们的系统包括三个主要部分,数据收集,数据预处理和分析以及数据分类。
The following steps were followed when creating the dataset.
创建数据集时,遵循以下步骤。
Cuckoo Sandbox application is installed on a computer running Ubuntu Linux distribution. The analysis machine was run as a virtual server to run and analyze malware. The Windows operating system is installed on this server.
Cuckoo Sandbox应用程序安装在运行Ubuntu Linux发行版的计算机上。 分析机作为虚拟服务器运行,以运行和分析恶意软件。 Windows操作系统已安装在此服务器上。
让我们编码 (Let’s coding)
We import the usual standard libraries to build an LSTM model to detect the malware.
我们导入常用的标准库以构建LSTM模型来检测恶意软件。
import pandas as pd
import matplotlib.pyplot as plt
import seaborn as sns
from sklearn.preprocessing import LabelEncoder
from sklearn.model_selection import train_test_split
from sklearn.metrics import confusion_matrix
from keras.preprocessing.text import Tokenizer
from keras.layers import LSTM, Dense, Dropout, Embedding
from keras.preprocessing import sequence
from keras.utils import np_utils
from keras.models import Sequential
from keras.layers import SpatialDropout1D
from mlxtend.plotting import plot_confusion_matrix
In this work, we will use standard our malware dataset to show the results. You can access the dataset from My GitHub Repository. We need to merge the call and the label datasets.
在这项工作中,我们将使用标准的恶意软件数据集来显示结果。 您可以从My GitHub Repository访问数据集。 我们需要合并调用和标签数据集。
malware_calls_df = pd.read_csv("calls.zip", compression="zip",
sep="\t", names=["API_Calls"])
malware_labels_df = pd.read_csv("types.zip", compression="zip",
sep="\t", names=["API_Labels"])
malware_calls_df["API_Labels"] = malware_labels_df.API_Labels
malware_calls_df["API_Calls"] = malware_calls_df.API_Calls.apply(lambda x: " ".join(x.split(",")))
malware_calls_df["API_Labels"] = malware_calls_df.API_Labels.apply(lambda x: 1 if x == "Virus" else 0)
Let’s analyze the class distribution
让我们分析类的分布
sns.countplot(malware_calls_df.API_Labels)
plt.xlabel('Labels')
plt.title('Class distribution')
plt.savefig("class_distribution.png")
plt.show()

Now we can create our sequence matrix. In order to build an LSTM model, you need to create a tokenization based sequence matrix as the input dataset
现在我们可以创建序列矩阵。 为了构建LSTM模型,您需要创建一个基于标记化的序列矩阵作为输入数据集
max_words = 800
max_len = 100
X = malware_calls_df.API_Calls
Y = malware_calls_df.API_Labels.astype('category').cat.codes
tok = Tokenizer(num_words=max_words)
tok.fit_on_texts(X)
print('Found %s unique tokens.' % len(tok.word_index))
X = tok.texts_to_sequences(X.values)
X = sequence.pad_sequences(X, maxlen=max_len)
print('Shape of data tensor:', X.shape)
X_train, X_test, Y_train, Y_test = train_test_split(X, Y,
test_size=0.15)
le = LabelEncoder()
Y_train_enc = le.fit_transform(Y_train)
Y_train_enc = np_utils.to_categorical(Y_train_enc)
Y_test_enc = le.transform(Y_test)
Y_test_enc = np_utils.to_categorical(Y_test_enc)Found 278 unique tokens.
Shape of data tensor: (7107, 100)
The LSTM based classification model is then given for example as exercise here:
然后在此处作为示例给出基于LSTM的分类模型:
def malware_model(act_func="softsign"):
model = Sequential()
model.add(Embedding(max_words, 300, input_length=max_len))
model.add(SpatialDropout1D(0.1))
model.add(LSTM(32, dropout=0.1, recurrent_dropout=0.1,
return_sequences=True, activation=act_func))
model.add(LSTM(32, dropout=0.1, activation=act_func, return_sequences=True))
model.add(LSTM(32, dropout=0.1, activation=act_func))
model.add(Dense(128, activation=act_func))
model.add(Dropout(0.1))
model.add(Dense(256, activation=act_func))
model.add(Dropout(0.1))
model.add(Dense(128, activation=act_func))
model.add(Dropout(0.1))
model.add(Dense(1, name='out_layer', activation="linear"))
return model
The next step is to train the model. I trained and saved my model. Because of the dataset, the training stage takes lots of time. In order to reduce the execution time, you can load my previous trained model from the GitHub repository.
下一步是训练模型。 我训练并保存了模型。 由于存在数据集,训练阶段需要很多时间。 为了减少执行时间,您可以从GitHub存储库加载我以前训练有素的模型。
model = malware_model()
print(model.summary())
model.compile(loss='mse', optimizer="rmsprop",
metrics=['accuracy'])
filepath = "lstm-malware-model.hdf5"
model.load_weights(filepath)
history = model.fit(X_train, Y_train, batch_size=1000, epochs=10,
validation_data=(X_test, Y_test), verbose=1)Model: "sequential"
_________________________________________________________________
Layer (type) Output Shape Param #
=================================================================
embedding (Embedding) (None, 100, 300) 240000
_________________________________________________________________
spatial_dropout1d (SpatialDr (None, 100, 300) 0
_________________________________________________________________
lstm (LSTM) (None, 100, 32) 42624
_________________________________________________________________
lstm_1 (LSTM) (None, 100, 32) 8320
_________________________________________________________________
lstm_2 (LSTM) (None, 32) 8320
_________________________________________________________________
dense (Dense) (None, 128) 4224
_________________________________________________________________
dropout (Dropout) (None, 128) 0
_________________________________________________________________
dense_1 (Dense) (None, 256) 33024
_________________________________________________________________
dropout_1 (Dropout) (None, 256) 0
_________________________________________________________________
dense_2 (Dense) (None, 128) 32896
_________________________________________________________________
dropout_2 (Dropout) (None, 128) 0
_________________________________________________________________
out_layer (Dense) (None, 1) 129
=================================================================
Total params: 369,537
Trainable params: 369,537
Non-trainable params: 0
_________________________________________________________________
None
Epoch 1/10
7/7 [==============================] - 22s 3s/step - loss: 0.0486 - accuracy: 0.9487 - val_loss: 0.0311 - val_accuracy: 0.9672
Epoch 2/10
7/7 [==============================] - 21s 3s/step - loss: 0.0378 - accuracy: 0.9591 - val_loss: 0.0302 - val_accuracy: 0.9672
Epoch 3/10
7/7 [==============================] - 21s 3s/step - loss: 0.0364 - accuracy: 0.9604 - val_loss: 0.0362 - val_accuracy: 0.9625
Epoch 4/10
7/7 [==============================] - 20s 3s/step - loss: 0.0378 - accuracy: 0.9593 - val_loss: 0.0328 - val_accuracy: 0.9616
Epoch 5/10
7/7 [==============================] - 22s 3s/step - loss: 0.0365 - accuracy: 0.9609 - val_loss: 0.0351 - val_accuracy: 0.9606
Epoch 6/10
7/7 [==============================] - 21s 3s/step - loss: 0.0369 - accuracy: 0.9601 - val_loss: 0.0369 - val_accuracy: 0.9606
Epoch 7/10
7/7 [==============================] - 22s 3s/step - loss: 0.0371 - accuracy: 0.9594 - val_loss: 0.0395 - val_accuracy: 0.9625
Epoch 8/10
7/7 [==============================] - 22s 3s/step - loss: 0.0378 - accuracy: 0.9601 - val_loss: 0.0365 - val_accuracy: 0.9588
Epoch 9/10
7/7 [==============================] - 22s 3s/step - loss: 0.0358 - accuracy: 0.9618 - val_loss: 0.0440 - val_accuracy: 0.9456
Epoch 10/10
7/7 [==============================] - 21s 3s/step - loss: 0.0373 - accuracy: 0.9589 - val_loss: 0.0354 - val_accuracy: 0.9644
模型评估 (Model Evaluation)
Now, we have finished the training phase of the LSTM model. We can evaluate our model’s classification performance using the confusion matrix. According to the confusion matrix, the model’s classification performance quite good.
现在,我们已经完成了LSTM模型的训练阶段。 我们可以使用混淆矩阵评估模型的分类性能。 根据混淆矩阵,该模型的分类性能相当好。
y_test_pred = model.predict_classes(X_test)
cm = confusion_matrix(Y_test, y_test_pred)
plot_confusion_matrix(conf_mat=cm,
show_absolute=True,
show_normed=True,
colorbar=True)
plt.savefig("confusion_matrix.png")
plt.show()

Let’s continue with the training history of our model.
让我们继续我们模型的训练历史。
plt.plot(history.history['accuracy'])
plt.plot(history.history['val_accuracy'])
plt.title('model accuracy')
plt.ylabel('accuracy')
plt.xlabel('epoch')
plt.legend(['train', 'test'], loc='upper left')
plt.grid()
plt.savefig("accuracy.png")
plt.show()
plt.plot(history.history['loss'])
plt.plot(history.history['val_loss'])
plt.title('model loss')
plt.ylabel('loss')
plt.xlabel('epoch')
plt.legend(['train', 'test'], loc='upper left')
plt.grid()
plt.savefig("loss.png")
plt.show()

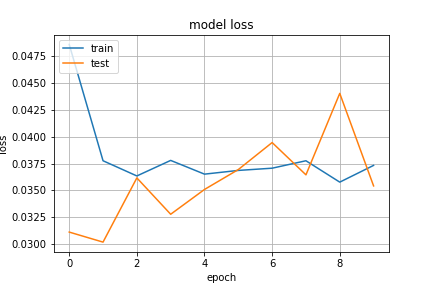
结论 (Conclusion)
The purpose of this study was to create an LSTM based malware detection model using my previous malware dataset. Although our dataset contains instances that belong to some malware families with unbalanced distribution, we have shown that this problem does not affect classification performance.
这项研究的目的是使用我以前的恶意软件数据集创建基于LSTM的恶意软件检测模型。 尽管我们的数据集包含的实例属于不均衡分布的某些恶意软件家族,但我们已经表明此问题不会影响分类性能。
翻译自: https://towardsdatascience.com/deep-lstm-based-malware-analysis-6b36ac247f34
基于深度学习lstm