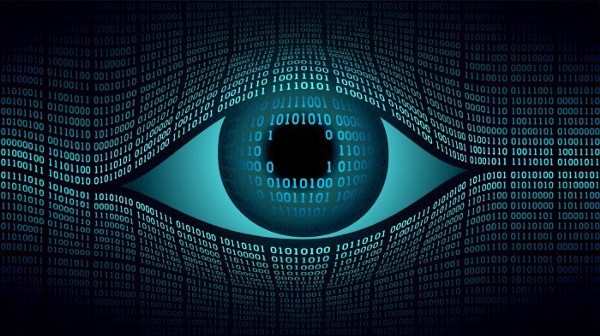
Have you ever wondered how Instagram takes care of the abusive and inappropriate images shared by some of its users? How Google Photos image tagging works? Or how companies like Twitter, Facebook, Pinterest, Reddit etc. take care of copyrighted content?
您是否曾经想过Instagram如何处理一些用户共享的辱骂和不当图片? Google相册图片标签如何工作? 还是Twitter,Facebook,Pinterest,Reddit等公司如何处理受版权保护的内容?
This classification of images into different categories is what is known as image classification
将图像分类为不同的类别就是所谓的图像分类
These are just some of the examples of what image classification can do. Multi-class Image classification has become an important computer vision problem in today’s world.
这些只是图像分类功能的一些示例。 多类图像分类已成为当今世界上重要的计算机视觉问题。
介绍 (Introduction)
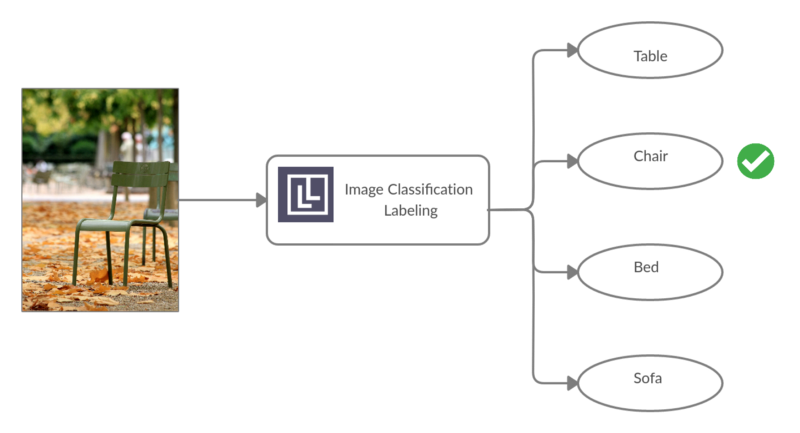
Image classification is the process of identifying and classifying what is present in the images. As human beings, we see a lot of objects everyday and identify most of them very easily and that too non-deliberately. This capability of object identification develops over the age as we gain experience.
图像分类是识别和分类图像中存在的内容的过程。 作为人类,我们每天都会看到很多物体,并且非常容易地识别它们中的大多数,而且太过刻意。 随着经验的积累,这种物体识别的能力随着年龄的增长而发展。
As we learn with experience, computers tend to learn with the help of data — lots of data. So, we feed the computer enough data so that it can learn to identify objects quickly. This data is then used to train deep learning models also called artificial neural networks (ANNs). In case of image classification, we use special types of ANNs called convolutional neural networks.
随着经验的积累,计算机趋向于借助数据(大量数据)进行学习。 因此,我们向计算机提供了足够的数据,以便它可以学习快速识别对象。 然后,该数据将用于训练也称为人工神经网络(ANN)的深度学习模型。 在图像分类的情况下,我们使用称为卷积神经网络的特殊类型的人工神经网络。
进行图像分类时面临的挑战 (Challenges faced while performing image classification)
It looks easy that what we need is just data and neural networks trained on that data and we get a model that classifies images conveniently. But in reality there are a lot of complications.
看起来很简单,我们需要的只是数据和基于该数据训练的神经网络,并且我们得到了一个方便地对图像进行分类的模型。 但实际上存在很多复杂性。
Lack of labeled data First and the foremost challenge faced is the lack of structured and labeled data. For tasks like image classification, we need labeled data.
缺少标记数据首先要面对的最大挑战是缺乏结构化和标记数据。 对于图像分类等任务,我们需要标记数据。
Fine-tuning models While training neural networks for real world applications, we may not get expected performance. This usually happens because either there might be some errors in data preprocessing or inconsistencies in selection of hyperparameters.
模型的微调在为实际应用训练神经网络时,我们可能无法获得预期的性能。 这通常是因为数据预处理中可能存在一些错误或超参数的选择不一致。
我们在Labellerr的工作方式 (How we do it at Labellerr)
Labellerr is a data annotation tool powered by artificial intelligence. It is aimed at bridging the gap between layman users and artificial intelligence providing features for a wide range of users from complete techy to beginners. Labellerr provides a lot of such features like:
Labellerr是由人工智能提供支持的数据注释工具。 它旨在弥合外行用户和人工智能之间的鸿沟,为从初学者到初学者的广泛用户提供功能。 Labellerr提供了许多这样的功能,例如:
ML assisted labeling Labellerr provides machine learning assisted labeling features also known as auto labeling.
ML辅助标签Labellerr提供了机器学习辅助标签功能,也称为自动标签。
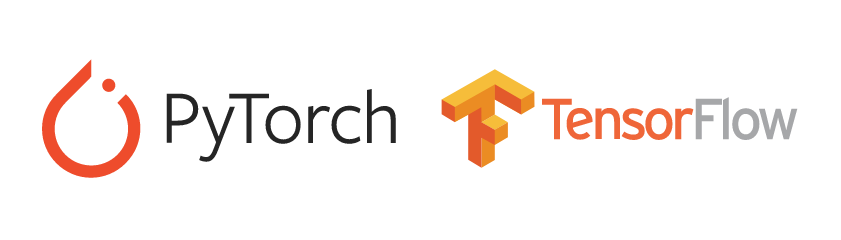
User friendly interface Labellerr’s user interface has been developed in such a way that even a beginner in ML field can train their models with ease.
用户友好的界面Labellerr的用户界面经过开发,即使是ML领域的初学者也可以轻松地训练他们的模型。
Faster labeling speed Labellerr supports multiple annotators at a time to increase the productivity in the labeling process.
更快的贴标速度Labellerr一次支持多个注释器,以提高贴标过程的生产率。
Multiple data connectors to import data Labellerr provides you with support of all these data storage options like Google Drive, Google Cloud Storage, AWS S3, Dropbox, local storage, as csv of file links etc. Labellerr recently launched a feature to extract image data by scraping from google.
用于导入数据的多个数据连接器Labellerr为您提供所有这些数据存储选项的支持,例如Google Drive,Google Cloud Storage,AWS S3,Dropbox,本地存储以及文件链接的csv等。Labellerr最近启动了一项功能,可通过从Google抓取。
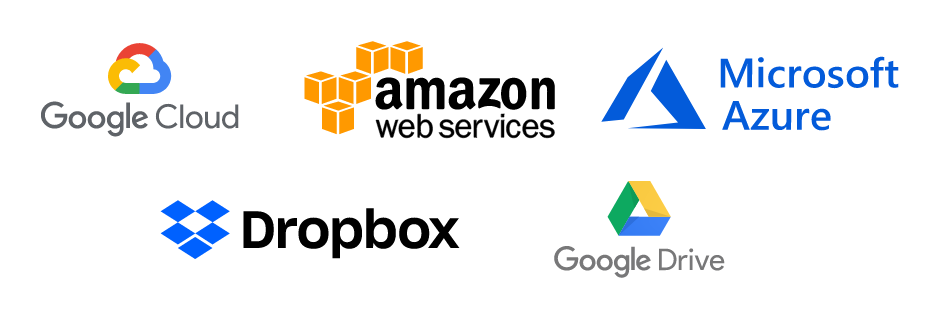
Easy export of labelled data in various formats. Labellerr supports multiple labelled data export options like csv, json, xml for different use cases.
轻松导出各种格式的标签数据。 Labellerr支持针对不同用例的多个标记数据导出选项,例如csv,json,xml。
现在,让我们通过图像分类示例了解Labellerr如何在机器学习注释世界中取得突破: (Now let’s understand how Labellerr has become a breakthrough in the machine learning annotation world with an image classification example:)
问题陈述 (Problem Statement)
Let us take a look at the medical image classification problem- Detection of fractures in X-Rays. For a diagnostician or a doctor, sometimes it becomes very difficult to identify whether there is any evidence of fracture in X-Ray or not. If fracture is not identified on time, then it may lead to bone deformity, permanent nerve damage, and muscle and ligament damage. So, here comes deep learning into the picture. We train neural networks on X-Ray data and then it will identify fractures within a fraction of second.
让我们看一下医学图像分类问题-X射线中的骨折检测。 对于诊断师或医生而言,有时很难确定X射线是否存在骨折迹象。 如果未及时发现骨折,则可能导致骨骼变形,永久性神经损伤以及肌肉和韧带损伤。 因此,将深度学习融入其中。 我们使用X射线数据训练神经网络,然后它将在几分之一秒内识别出骨折。
数据集准备 (Dataset Preparation)
A model need not be as great if we have a sufficient amount of data. But where does this data come from? It is obvious that a lot of such scans are conducted every day in India and these scans are stored in digital formats. So, the data for this problem statement can be collected from diagnostic centres all across the country. Now, even after collecting the data, it is unstructured and unlabeled. So, we need some kind of labeling tool which should provide some kind of interface to ease the labeling process.
如果我们有足够的数据量,则模型不必太大。 但是这些数据从何而来? 显然,每天在印度进行很多此类扫描,并且这些扫描以数字格式存储。 因此,可以从全国各地的诊断中心收集此问题陈述的数据。 现在,即使在收集数据之后,它也是非结构化和未标记的。 因此,我们需要某种标记工具,该工具应提供某种接口以简化标记过程。
Labellerr provides a seamless interface with a variety of features to label the datasets and structure them in the best possible way.
Labellerr提供了具有各种功能的无缝界面,以标记数据集并以最佳方式构造它们。
Soon we are planning to add Tensorflow Datasets and Kaggle Datasets connectors as well.
不久我们计划添加Tensorflow数据集和Kaggle数据集连接器。
在Labellerr上注册 (Sign Up On Labellerr)
You can sign up on Labellerr by visiting Labellerr.
您可以通过访问Labellerr来注册Labellerr 。
在Labellerr上创建新项目并导入数据 (Creating new project on Labellerr and import data)
When you sign in in your tool for the first time you will automatically be redirected to the project creation page. Or you can go to the settings screen to create a new project.
首次登录工具时,您将自动重定向到项目创建页面。 或者,您可以转到设置屏幕来创建一个新项目。
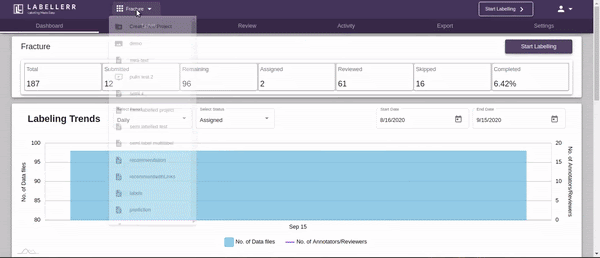
开始贴标 (Start Labeling)
To start labeling just click on the label navigation tab or click the Start labeling button in the top right corner and you will be redirected to the labeling screen
要开始贴标签,只需单击标签导航选项卡或单击右上角的“开始贴标签”按钮,您将被重定向到标签屏幕
You can select the labels on the left side of the screen and then click submit to save the labels. You can also skip a file if you are not sure about any particular labels and want to label it later or the image is not clear enough to label it.
您可以选择屏幕左侧的标签,然后单击提交以保存标签。 如果您不确定某个特定标签并想稍后对其进行标签,或者图像不够清晰以致无法对其进行标签,则也可以跳过该文件。
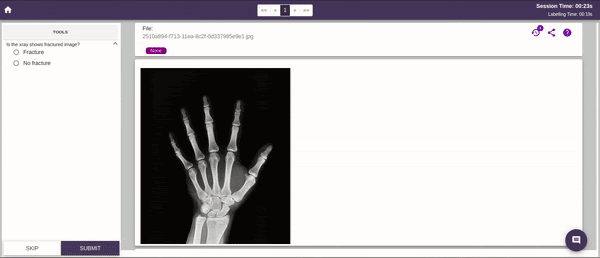
标签分析(Labeling analytics)
You can view labeling stats on the dashboard on the home screen.
您可以在主屏幕上的仪表板上查看标签统计信息。
Statuses and their meanings:
状态及其含义:
Total — Total number of files linked to the project
总计-链接到项目的文件总数
Submitted — Number of files that have been labelled and reviewed by the reviewer.
已提交-已由审阅者标记和审阅的文件数。
Reviewed — Number of files that have been labelled and pending for review.
已审阅—已标记并等待审阅的文件数。
Remaining — Number of files yet to be labelled
剩余-尚未标记的文件数
Assigned — Number of files that have been assigned to different users but still pending to get labelled.
已分配-已分配给不同用户但仍待标记的文件数。
Skipped — Number of files skipped by annotators.
已跳过—注释者跳过的文件数。

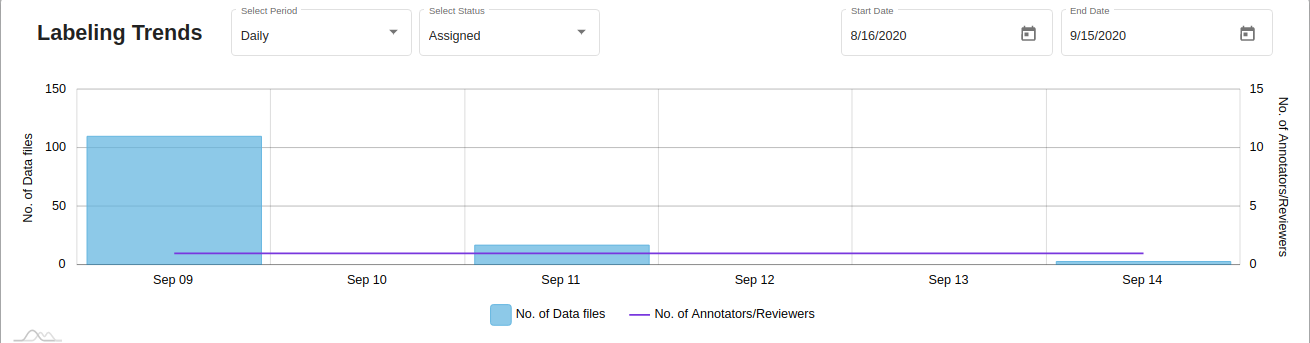
ML辅助贴标(ML Assisted Labeling)
How does it (training) work? — ML assisted labeling needs little amount of data to be labeled beforehand so that it can use that data to train models. These models are then used for labeling rest of the data automatically. Models are continuously retrained if and when required by Labellerr depending upon the performance they give.
(培训)如何工作? — ML辅助标记需要预先标记少量数据,以便可以使用该数据训练模型。 然后,这些模型将用于自动标记其余数据。 Labellerr视需要提供的性能而定,并在需要时对模型进行连续训练。
Prediction on data — While labeling, if the autolabel feature is enabled, you can see suggestions for the questions. You can either just see the suggestion or automatically select the label that is suggested.
数据预测—标记时,如果启用了自动标记功能,则可以看到有关问题的建议。 您可以只看到建议,也可以自动选择建议的标签。
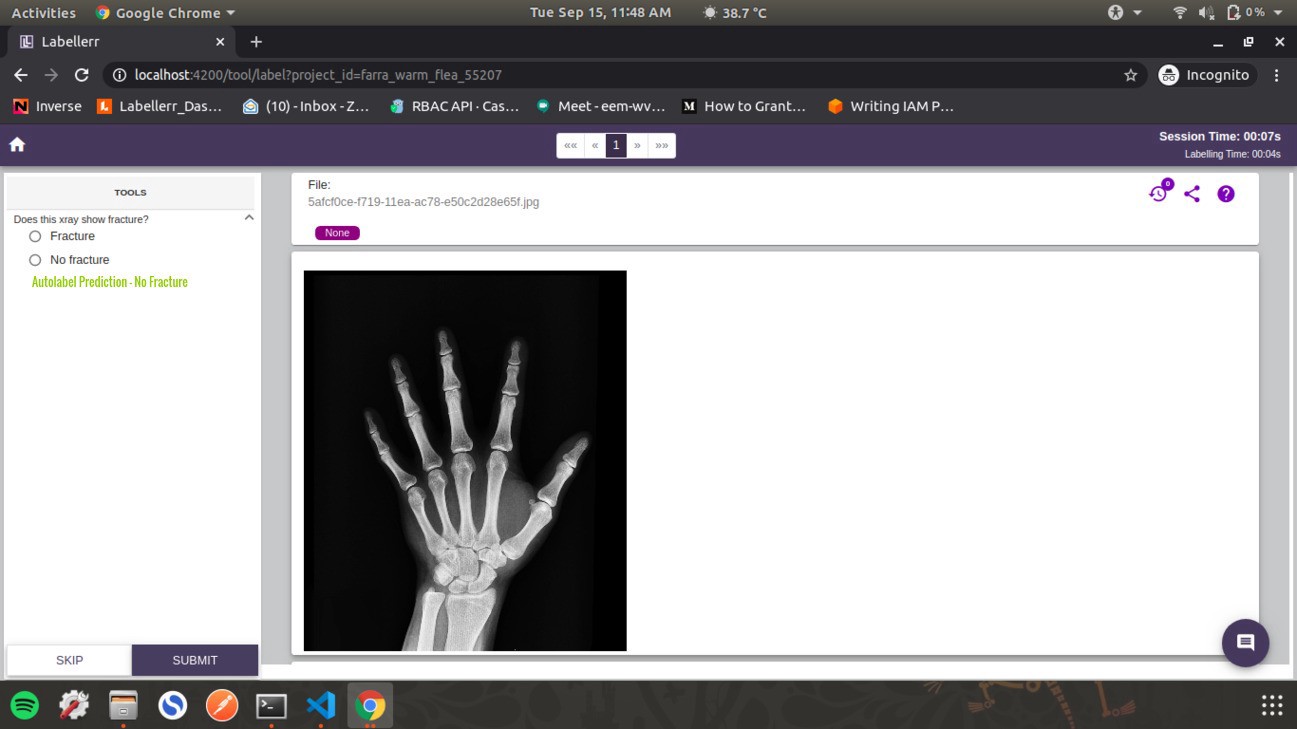
Trained model stats (Accuracy) — You can always view the models that have been trained on your data with stats like accuracy and loss specific to each model and select a model you want to use for your autolabel. By default, the best model trained on most files and the best accuracy is used for predictions.
训练有素的模型统计信息(准确性)-您始终可以查看已对数据进行训练的模型,这些统计信息包括每种模型特有的准确性和损失等统计信息,并选择要用于自动标签的模型。 默认情况下,将对大多数文件训练的最佳模型和最佳准确性用于预测。
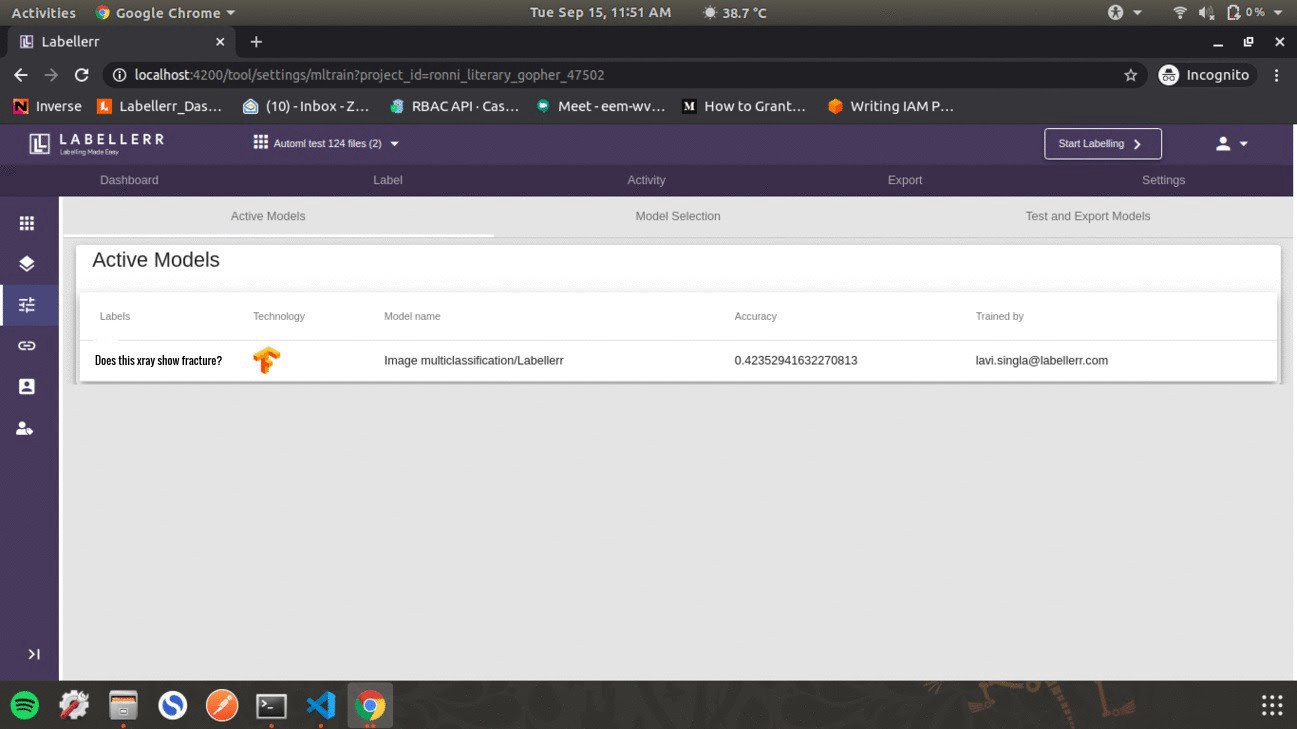
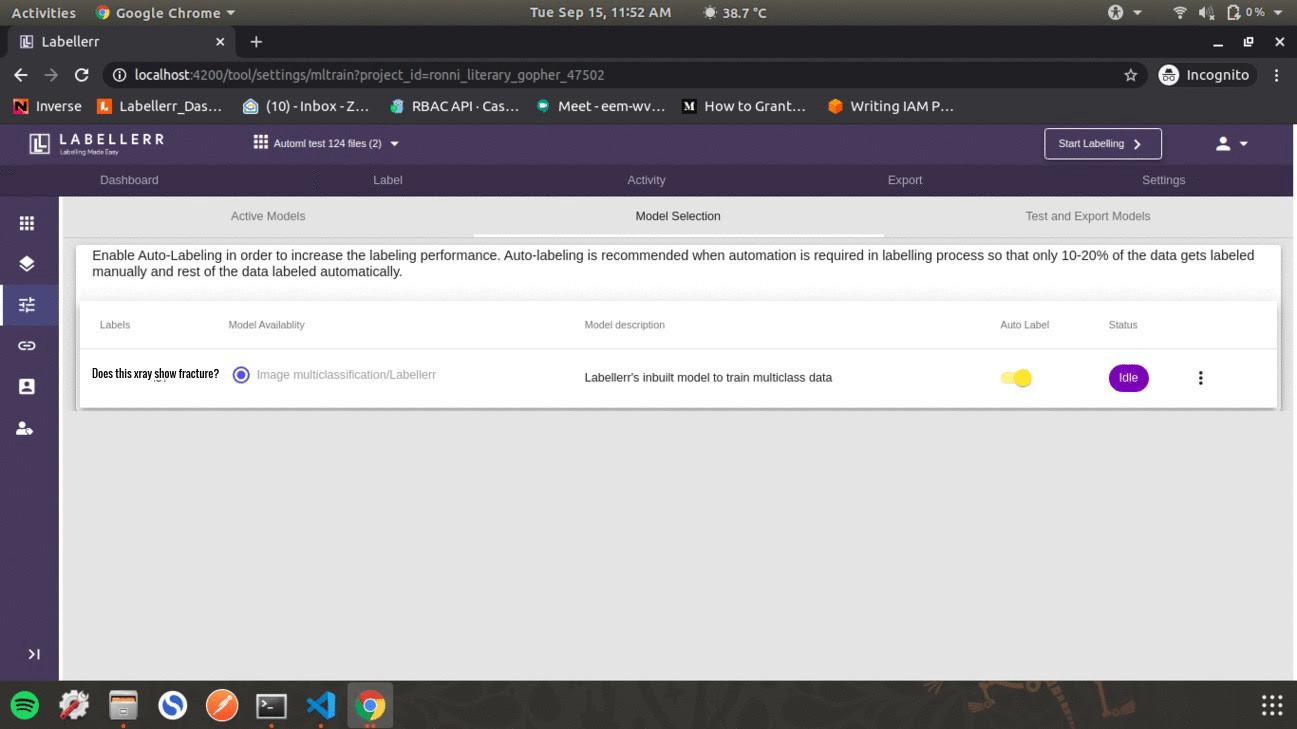
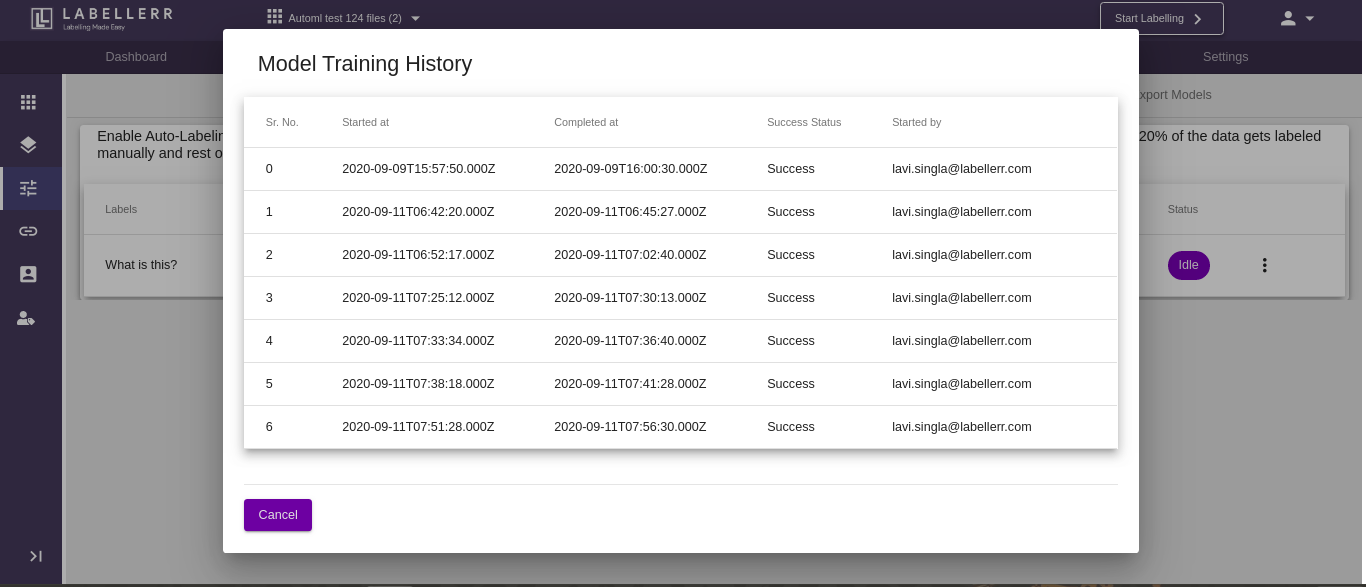
结论(Conclusion)
Labellerr as an annotation tool, handles the complete pipeline of building an image classification model from preparing data, labeling it with minimum effort ,then training the model with best possible algorithm for your use case and exporting the data in your required format.
Labellerr作为注解工具,可以处理从准备数据到构建图像分类模型,以最小的努力对其进行标记,然后针对您的用例使用最佳算法对模型进行训练并以所需格式导出数据的完整流程。
Got your own image classification use case ?
有自己的图像分类用例吗?
Signup on Labellerr and start using it to build AI and ML solutions
在Labellerr上注册并开始使用它来构建AI和ML解决方案
For any queries, write to us at support@tensormatics.com
如有任何疑问,请写信给我们support@tensormatics.com