fastai 文本分类
The internet has become a basic necessity in recent times and a lot of things which happen physically in our world are on the verge of being digitised. Already a substantial proportion of the world population uses the internet for day to day chores, entertainment, academic research etc. It then is a big responsibility to keep the internet a safe space for everyone to come and interact because there are all sorts of people posting stuff on the internet without being conscious of its consequences.
互联网已成为近来的基本必需品,我们世界上发生的许多实际事情都处于数字化的边缘。 世界上已经有很大一部分人将互联网用于日常琐事,娱乐,学术研究等。因此,为互联网提供一个安全的空间,让所有人都能参与互动是一大责任,因为各种各样的人都在张贴信息。互联网上的任何内容,而不会意识到其后果。
This post goes through the process of making a text classifier which takes in a piece of text (phrase, sentence, paragraph any length text) and tells if the text falls under a range of different types of malignant prose. The topics covered in this post are
这篇文章经历了一个文本分类器的过程,该文本分类器接受一段文本(短语,句子,段落任意长度的文本),并判断该文本是否属于一系列不同类型的恶性散文。 这篇文章涵盖的主题是
You can click on any of the above bullet points to navigate to the respective section.
您可以单击以上任何要点以导航到相应部分。
Disclaimer: The dataset used here contains some text that may be considered profane, vulgar, or offensive.
免责声明:此处使用的数据集包含一些可能被亵渎,粗俗或令人反感的文本。
介绍 (Introduction)
Natural Language Processing is a field that deals with understanding the interactions between computers and human language. Since a lot of things are going online or digital, and since these services are democratised to the whole world, the scale at which this data is generated is humongous. In these times where everyone on the planet is putting their opinions, thoughts, facts, essays, poems etc. online, monitoring and moderating these pieces of text is an inhuman task (even when we think of humans as a community and not an individual being).
自然语言处理是一个致力于理解计算机与人类语言之间交互作用的领域。 由于许多事物正在在线或数字化,并且由于这些服务已普及到整个世界,因此生成此数据的规模是巨大的。 在当今这个星球上的每个人都将其观点,思想,事实,论文,诗歌等置于网上的时代,监视和整理这些文本片段是一项不人道的任务(即使我们将人类视为一个社区而不是一个个体, )。
Thanks to the advent of high capacity GPUs and TPUs and the recent advances in AI for text applications, we have come up with a lot of techniques to tackle this problem. Recurrent Neural Networks are the key element with the help of which these problems are addressed. fastai, a deep learning library built on top of PyTorch, developed by Jeremy Howard and Sylvain Gugger makes building applications for tasks like these very user-friendly and simple.
由于大容量GPU和TPU的出现以及用于文本应用程序的AI的最新发展,我们提出了许多技术来解决此问题。 递归神经网络是解决这些问题的关键要素。 fastai是杰里米·霍华德(Jeremy Howard)和西尔文·古格(Sylvain Gugger)开发的基于PyTorch的深度学习库,它为此类任务的构建应用程序变得非常用户友好和简单。
Let’s the get started and learn how to do text classification using fastai.
让我们开始吧,学习如何使用fastai进行文本分类。
从Kaggle获取数据 (Getting Data from Kaggle)
The data we’ll use for demonstrating the process of multi-label text classification is obtained from Toxic Comment Classification Challenge on Kaggle.
我们将用于证明多标签文本分类过程的数据是从Kaggle的有毒评论分类挑战中获得的。
Our model will be responsible for detecting different types of toxicity like threats, obscenity, insults, and identity-based hate. The dataset consists comments from Wikipedia’s talk page edits. Without any further ado, let’s get started with downloading this dataset.
我们的模型将负责检测不同类型的毒性,例如威胁,淫秽,侮辱和基于身份的仇恨。 数据集包含来自Wikipedia对话页编辑的评论。 事不宜迟,让我们开始下载此数据集。
import os
# Set your username and key from the kaggle API from your account
os.environ["KAGGLE_USERNAME"] = "###"
os.environ["KAGGLE_KEY"] = "###"
os.chdir("/content/drive/My Drive/Colab Notebooks")
os.mkdir("Toxic Comments Data")
os.chdir("./Toxic Comments Data")
# Download the data from kaggle to the directory you just created
!pip install kaggle
!kaggle competitions download -c jigsaw-toxic-comment-classification-challenge
# Unzip all the csv files and remove the zip files
!unzip train.csv.zip && rm train.csv.zip
!unzip test.csv.zip && rm test.csv.zip
!unzip test_labels.csv.zip && rm test_labels.csv.zip
!unzip sample_submission.csv.zip && rm sample_submission.csv.zip
You can either download the dataset from Kaggle manually or use the API provided by kaggle using the above commands.
您可以从Kaggle手动下载数据集,也可以使用上述命令使用kaggle提供的API。
To use the API, you’ll have to create an account with Kaggle and generate the API key which allows you to use shell commands for downloading the dataset from kaggle and also making submissions for predictions from the working notebook or from the shell. Once you create a Kaggle account and create the API key, you will get a json file which contains both your username and key. Those need to be input in the above code as per your unique credentials.
要使用该API,您必须使用Kaggle创建一个帐户并生成API密钥,该API密钥使您可以使用Shell命令从kaggle下载数据集,还可以从工作笔记本或Shell中提交预测数据。 创建Kaggle帐户并创建API密钥后,您将获得一个包含用户名和密钥的json文件。 这些需要根据您的唯一凭据输入以上代码。
This post by MRINALI GUPTA nicely explains how to get started with Kaggle API for downloading datasets.
MRINALI GUPTA的这篇帖子很好地解释了如何开始使用Kaggle API下载数据集。
数据探索 (Data Exploration)
Let’s read in both the train and test sets and get a hang of what data is contained in them.
让我们阅读训练和测试集,并了解其中包含的数据。
import os
# Read in the train and test sets.
os.chdir("/content/drive/My Drive/Colab Notebooks/Toxic Comments Data")
train_df = pd.read_csv("train.csv")
test_df = pd.read_csv("test.csv")
# Look at a few entries from the dataset
train_df.head()
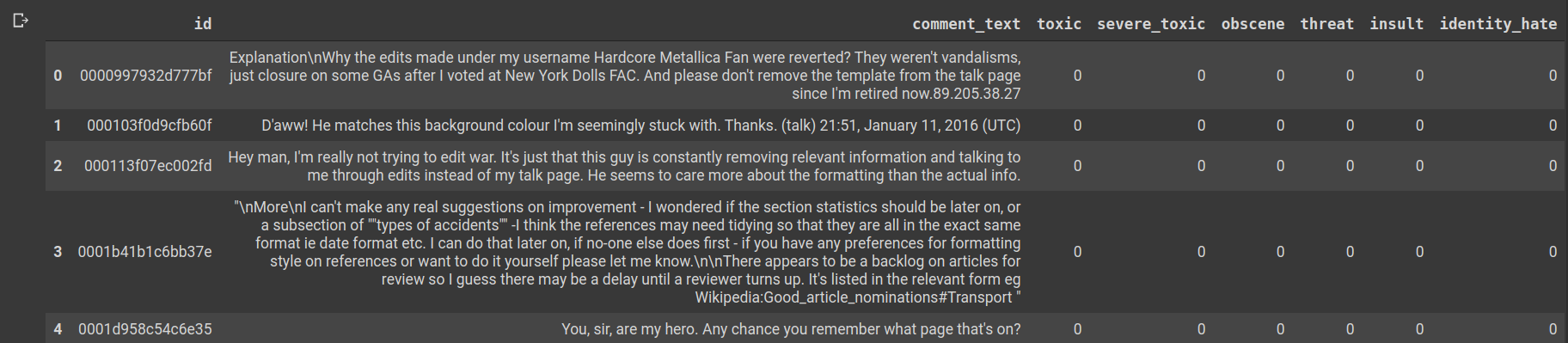
There are several fields in the dataframe.
数据框中有几个字段。
id: An identifier which is associated with ever comment text. Since this is picked up from Wikipedia’s talk page, it could probably be the identification of a person who has commented, or an HTML DOM id of the text that they’ve posted.
id :与曾经评论文本关联的标识符。 由于此内容是从Wikipedia的讨论页中提取的,因此很可能是发表评论的人的身份,或者是他们发布的文本HTML DOM ID。
comment_text: The text of the comment which the user has posted.
comment_text :用户已发布的评论的文本。
toxic, severe_toxic, obscene, threat, insult, identity_hate: These columns denote the presence of the eponymous elements in comment_text. If they’re absent they’re represented by zeros else they’re represented by a 1.
毒性,严重毒性,淫秽,威胁,侮辱,identity_hate:这些列表示comment_text中存在同名元素。 如果不存在,则以零表示,否则以1表示。
These elements are independent in the sense, they’re not mutually exclusive for eg. A comment can be both toxic and insulting, or it’s not necessary that if a comment is toxic it couldn’t be obscene and so on.
这些元素在某种意义上是独立的,例如,它们并不互斥。 评论既有毒又有侮辱性,或者没有必要,如果评论有毒,就不会淫秽等等。
# Look at the distribution of comments
f = lambda x: train_df[x].sum()
comment_types = train_df.columns[2:]
comment_counts = [f(x) for x in comment_types]
fig, ax = plt.subplots(1, 1, figsize = (10, 6))
plt.bar(comment_types, comment_counts)
ax.set_title(f"Comment distribution of {len(train_df)} wikipedia comments.")
plt.tight_layout();
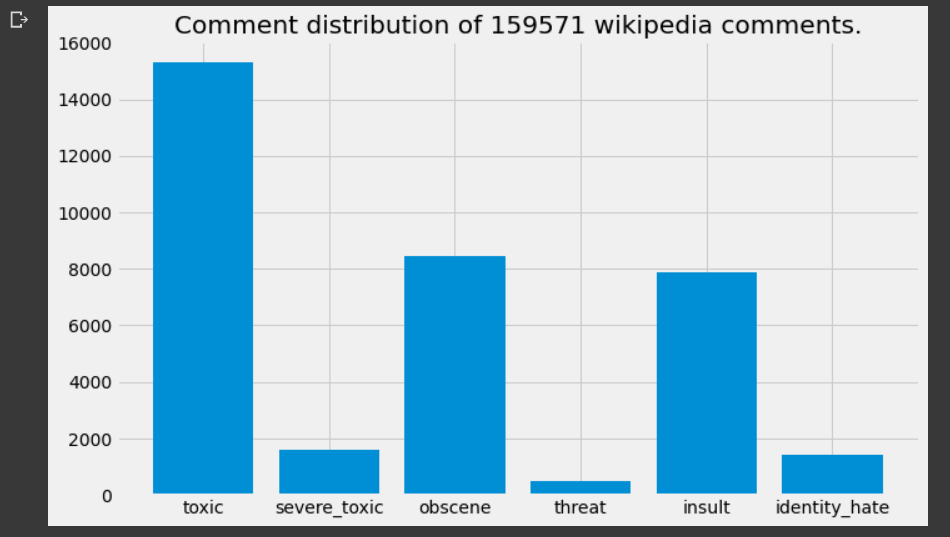
In general, there’s a lot less comments that have objectionable text; considering that we’ve got more than a hundred thousand comments, there’s less than tens of thousand objectionable categories (except toxic which is just a few more). This is good to know but it would be for the best if there were still fewer texts of this kind.
通常,带有令人反感的文字的评论要少得多; 考虑到我们有十万多条评论,只有不到几万种令人反感的类别(有毒的类别除外)。 很高兴知道这一点,但是如果这种文本的数量仍然很少,那将是最好的。
Also, the text was annotated by humans to have these labels. This task of annotation is a huge task and a lot of human interpretation and bias will have come along with these annotations. It’s something that needs to be remembered and we’ll talk about this in closing thoughts.
同样,文本被人类注释以具有这些标签。 注释的这项任务是一项艰巨的任务,并且这些注释会伴随许多人类的解释和偏见。 这是需要记住的事情,我们将在总结思想时加以讨论。
多标签文本分类的方法 (Approach toward multilabel text classification)
Text or sentences are sequences of individual units — words, sub-words or characters (depends on the language you speak). Any Machine Learning algorithm is not capable of handling anything other than numbers. So, we will first have to represent the data in terms of numbers.
文本或句子是各个单元的序列-单词,子单词或字符(取决于您所使用的语言)。 任何机器学习算法都不能处理数字以外的任何东西。 因此,我们首先必须用数字表示数据。
In any text related problems, first we create a vocabulary of words which basically is the total corpus of words which we will consider; any other word will be tagged with a special tag called unknown and put in that bucket. This process is called tokenization.
在与文本相关的任何问题中,首先,我们创建一个单词词汇表,该词汇表基本上是我们将要考虑的单词总语料库; 其他任何单词都将使用称为unknown的特殊标签进行标记,并放入该存储桶中。 此过程称为令牌化。
Next, we map every word to a numerical token and create a dictionary of words that stores this mapping. So every prose/comment/text is now converted into a list of numbers. This process is called numericalization.
接下来,我们将每个单词映射到一个数字标记,并创建一个存储该映射的单词字典。 因此,每个散文/评论/文本现在都转换为数字列表。 此过程称为数字化。
Most certainly the comments will not be of equal length, because people are not restricted to comment in exactly a fixed number of words. But when creating batches of text to feed to our network, we need them all to be of the same length. Therefore we pad the sentences with a special token or truncate the sentence if it’s too big to constrict to a fixed length. This process is called padding.
最肯定的是,评论的长度不会相等,因为人们不受限于以固定数量的单词进行评论。 但是,当创建一批文本以馈送到我们的网络时,我们需要它们的长度都相同。 因此,如果句子太大以致于不能固定为固定长度,我们会在句子中加上特殊标记或截断句子。 此过程称为填充。
While doing all the above, there are some other operations like lowercasing all the text, dealing with punctuation as separate tokens, understanding capitalization in spite of lowercasing and so on. This is where the good people at fastai make all of these things super easy for us.
在完成上述所有操作的同时,还有其他一些操作,例如将所有文本都小写,将标点符号作为单独的标记处理,尽管大写也理解大写等等。 这就是Fastai的好人使我们所有这些事情变得超级简单的地方。
xxpad: For padding, this is the standard token that’s used.
xxpad :对于填充,这是使用的标准令牌。
xxunk: When an oov (out of vocabulary) word is encountered, this token is used to replace that word.
xxunk :当遇到oov(词汇量)单词时,此标记用于替换该单词。
xxbos: At the start of every sentence, this is a token which indicates the start/beginning of a sequence.
xxbos :在每个句子的开头,这是一个标记,指示序列的开始/开始。
xxmaj: If a word is capitalised or title cased, this token is prefixed to capture that information.
xxmaj :如果单词为大写字母或首字母大写,则此标记带有前缀以捕获该信息。
xxrep: If a word is repeated, then in the tokenized representation, we will have that word followed by xxrep token followed by number of repetitions.
xxrep :如果重复一个单词,则在标记化表示中,我们将得到该单词,后跟xxrep令牌,后跟重复次数。
There’s some more semantic information handled with more such tokens but all of this makes sure to capture precious information about the text and the meaning behind it.
还有更多的语义信息使用更多的这样的令牌来处理,但是所有这些都确保捕获有关文本及其背后含义的宝贵信息。
Once this preprocessing is done, we can then right of the bat build an LSTM model to classify the texts into the respective labels. Words are represented as n-dimensional vectors which are colloquially called encoding/embedding. There’s a construct for Embedding in PyTorch which helps lookup the vector representation for a word given it’s numerical token and that’s followed by other RNN layers and fully connected layers to build an architecture which can take sequence as an input and return a bunch of probabilities as the output. These vector embeddings could be randomly initialized or borrowed from commonly available GLoVE or Word2Vec embeddings which have been trained on a large corpus of text so that they have a good semantic word understanding about context in that particular language in a generic sense.
一旦完成了预处理,我们就可以立即建立一个LSTM模型,将文本分类为各自的标签。 单词被表示为n维向量,俗称编码/嵌入。 在PyTorch中有一个Embedding的构造,可以在给定单词数字令牌的情况下帮助查找单词的向量表示,随后是其他RNN层和完全连接的层,以构建可将序列作为输入并返回几率作为输出。 这些矢量嵌入可以随机初始化,也可以从已经在大量文本集上进行训练的通用GLoVE或Word2Vec嵌入中借用,从而使它们对通用语言具有特定语言上下文的良好语义理解。
However, there’s a trick which could improve the results if we perform it before building a classifier. That’s what we’ll look at next.
但是,有一个技巧可以在构建分类器之前执行以改善结果。 这就是我们接下来要看的内容。
fastai v2中的语言模型 (Language Model in fastai v2)
fastai has suggested this tried and tested method of fine-tuning a Language Model before building any kind of classifier or application.
fastai建议在构建任何种类的分类器或应用程序之前对微调语言模型进行微调的这种久经考验的方法。
In a nutshell, what they say is if you have a set of word embeddings which were trained on a huge corpus, they have a very generic understanding of the words that they learned from that corpus. However, when we talk about classification of hate speech and obnoxious comments and toxic stuff, there’s a specific negative vibe associated with these sentences and that semantic context is not yet present in our embeddings. Also, many words/terms specific to our application (it may be medicine or law or toxic speech) may not be encountered often in that huge corpus from which we obtained the word embeddings. Those should be there included and represented well in the embeddings that our classifier is going to use.
简而言之,他们说的是,如果您有一组在庞大的语料库上训练过的单词嵌入,他们会对从该语料库中学到的单词有非常一般的理解。 但是,当我们谈论仇恨言论,令人讨厌的评论和有毒物品的分类时,与这些句子相关联的是特定的消极氛围,并且语义上下文还没有出现在我们的嵌入物中。 同样,在我们从中获得词嵌入的巨大语料库中,可能经常不会遇到许多我们应用程序特有的词/术语(可能是医学或法律或有毒的言论)。 这些应该包含在其中,并在我们的分类器将要使用的嵌入中很好地表示。
So, before building a classifier we’ll finetune a Language Model which has been trained on wikipedia text corpus. We will bind the train and test dataset comments together and feed them to the language model. This is because we’re not doing classification but simply guessing the next word of a sequence given the current sequence; it’s called a self supervised task. With the embeddings learned this way, we’ll be able to build a better classifier because it has an idea of the concepts specific to our corpus.
因此,在建立分类器之前,我们将对已在Wikipedia文本语料库上进行训练的语言模型进行微调。 我们将训练和测试数据集注释绑定在一起,并将其输入语言模型。 这是因为我们不是在进行分类,而是在给定当前序列的情况下简单地猜测序列的下一个单词。 这称为自我监督任务。 通过这种方式学习到的嵌入,我们将能够构建更好的分类器,因为它对特定于我们的语料库的概念有所了解。
Let’s see how we can instantiate and fine-tune a language model in fastai v2.
让我们看看如何在fastai v2中实例化和微调语言模型。
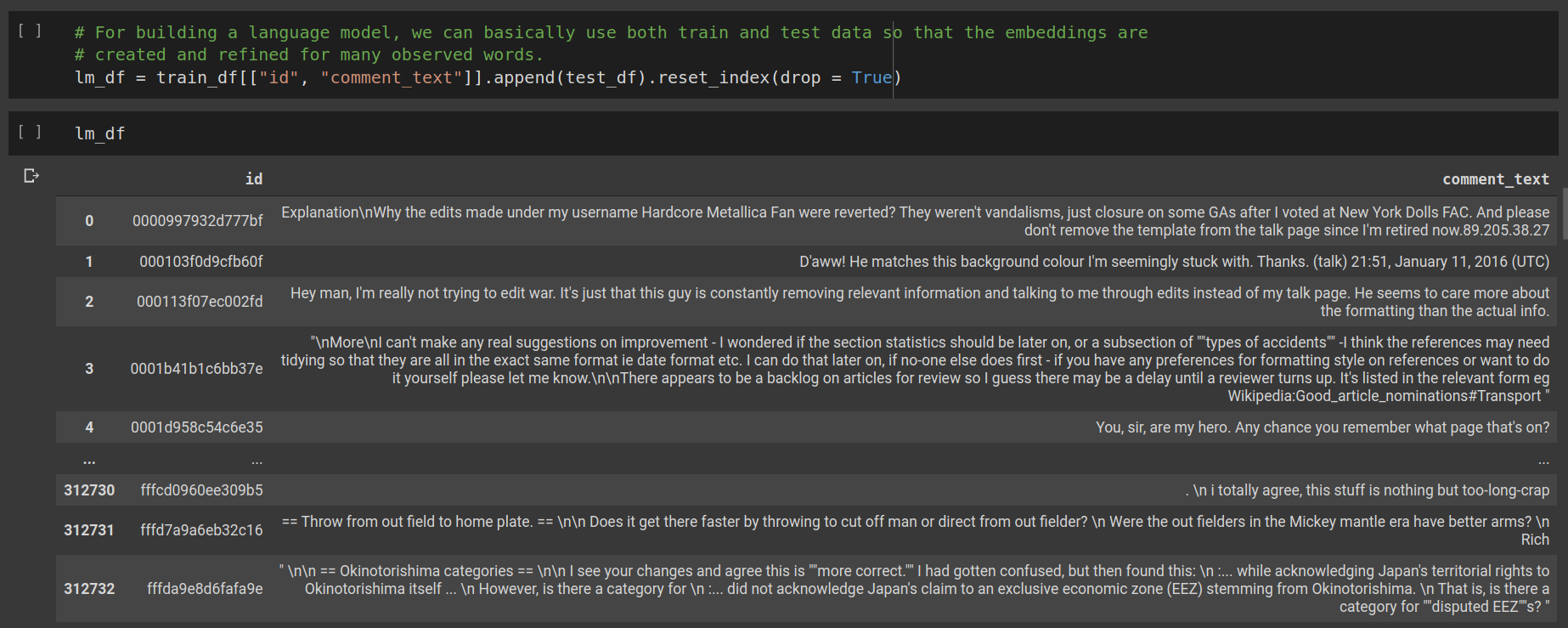
We append the train and test data and throw away the labels because we don’t need them in this self-supervised learning task. Next, we have to create a dataloader to tokenize these texts, do all the numericalization, padding and preprocessing before feeding it to the language model.
我们附加训练和测试数据并丢弃标签,因为在此自我监督的学习任务中不需要它们。 接下来,我们必须创建一个数据加载器以对这些文本进行标记,在将其输入语言模型之前进行所有的数字化,填充和预处理。
# Tokenize the dataframe created above to have all the descriptions tokenized properly and build a dataloader
# For creating a language model
dls_lm = TextDataLoaders.from_df(lm_df,
# Specify the column that contains the comments
text_col='comment_text',
# Specify how much data is within train and validation sets
valid_pct = .2,
# Mention explicitly that this dataloader is meant for language model
is_lm = True,
# Pick a sequence length i.e. how many words to feed through the RNN at once
seq_len = 72,
# Specify the batch size for the dataloader
bs = 64)
That’s how simple it is in fastai, you just have to wrap all the arguments in a factory method and instantiate the TextDataLoaders class with it. This would have otherwise taken at least a hundred lines of codes with proper commenting and stuff but thanks to fastai, it’s short and sweet. We can have a look at a couple of entries from a batch.
在fastai中就是这么简单,您只需要将所有参数包装在工厂方法中,并使用它实例化TextDataLoaders类。 否则,至少要用一百行代码加上适当的注释和填充,但是要感谢fastai,它又短又好。 我们可以查看一批中的几个条目。

As we can see the output is just offsetting the given sequence by one word which is in alignment with what we want, i.e. given a sequence, predict next word of a sequence. Once we have this dataloader, we can create a language model learner which can tune the encodings as per our corpus instead of the previous corpus of text.
如我们所见,输出只是将给定序列偏移一个单词,该单词与我们想要的对齐,即给定一个序列,预测该序列的下一个单词。 一旦有了这个数据加载器,我们就可以创建一个语言模型学习器,它可以根据我们的语料库而不是先前的文本语料库来调整编码。
# Create & fine tune the language model
learn = language_model_learner(dls_lm,
# Architecture to use
AWD_LSTM,
# Dropout percentage for regularization
drop_mult = .3,
# Metrics to understand the performance
metrics = [accuracy, Perplexity()]).to_fp16()
# To go a little easy on the GPUs, we will save the weights of model with 16-point floating
# Precision instead of the regular 32-point floating precision
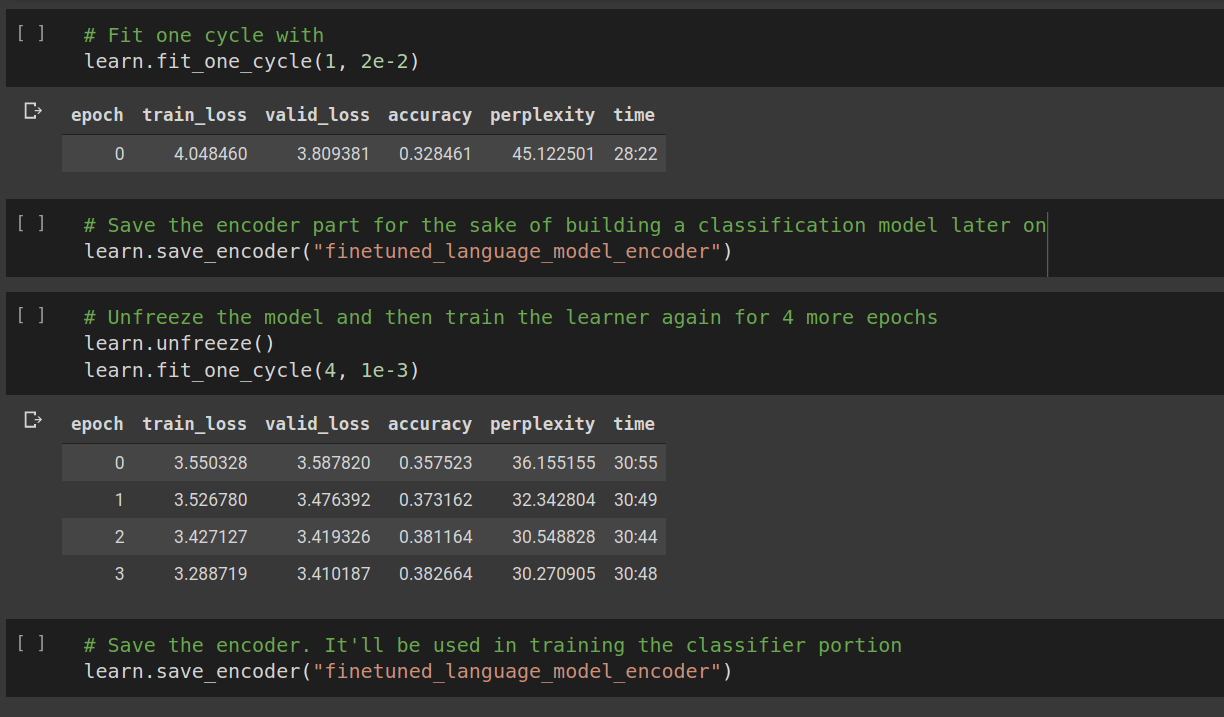
After we have the language model learner, we can fit the learner over several epochs and save the encodings using the save_encoder method. We can see that the language model can on an average predict with a 38% accuracy what the next word would be given the current sequence of words which is pretty decent for this dataset.
有了语言模型学习者之后,我们可以使学习者适应几个时期,并使用save_encoder方法保存编码。 我们可以看到,语言模型平均可以以38%的准确度预测下一个单词将被赋予当前单词序列的当前顺序,这对于该数据集而言相当不错。
Once we have this ready, now we can move to the next step of creating a classifier to identify the probabilities for different labels of the comment_text.
一旦准备好了,现在我们可以进入创建分类器的下一步,以标识comment_text的不同标签的概率。
fastai v2中的分类模型 (Classification Model in fastai v2)
Before we move to creating a classification model, there’s some bit of preprocessing that we need to perform in order to build a proper dataloader. At the time of writing this post, there’s an issue with the DataBlocks API for text which avoids it from inferring all the dependent variables properly, hence we have to resort to this method.
在开始创建分类模型之前,需要进行一些预处理以构建适当的数据加载器。 在撰写本文时,DataBlocks API的文本存在一个问题,该问题避免了它正确地推断所有因变量的情况,因此我们必须诉诸此方法。
Basically, we will have to create another column in our dataframe which indicates the presence or absence of individual label using a fixed delimiter. So, if a comment is obscene and toxic, our new column will show obscene;toxic where delimiter is “;”. Also for the rows which don’t have any objectionable text, we will call them sober for now for the sake of giving a label (without any label, fastai won’t create the dataloader).
基本上,我们将必须在数据框中创建另一列,以使用固定的定界符指示单个标签的存在与否。 因此,如果评论是淫秽且有毒的,我们的新列将在分隔符为“;”的地方显示淫秽;有毒。 对于没有任何令人反感的文本的行,为了给出标签,我们现在将它们称为清醒的(没有任何标签,fastai不会创建数据加载器)。
# Make a list of columns that would serve as your labels for this task
label_cols = list(train_df.columns[2:])
# Create a column of texts which has a list of all the categories.
# When all the entries are zeros, let's call the txt sober
def get_labels(row):
indcs = np.where(row == 1)[0]
if len(indcs) == 0:
return "sober"
return ";".join([label_cols[x] for x in indcs])
# Get the labels all in a nicely formatted style
labels = train_df[label_cols].apply(lambda row: get_labels(row), axis = 1)
# Add the labels object to our dataframe
train_df["Labels"] = labels

So we can see that there’s a column Labels added which contains a “;” delimited labels field where all our labels are denoted instead of the one-hot encoded format in which they’re provided.
因此,我们可以看到添加了一个标签列,其中包含“;” 分隔标签字段,其中表示了我们所有的标签,而不是提供标签的一键编码格式。
# Create a Dataloader to feed to the model
dls_blk = DataBlock(blocks = (TextBlock.from_df(text_cols = "comment_text", seq_len = 128),
MultiCategoryBlock),
get_x = ColReader(cols = "text"),
get_y = ColReader(cols = "Labels", label_delim = ";"),
splitter = TrainTestSplitter(test_size = 0.2, random_state = 21))
dls_clf = dls_blk.dataloaders(train_df,
bs = 64,
seed = 20)
Now, we create the dataloaders using the datablocks API using “comment_text” field for x and “Labels” field for y respectively. If we would have mentioned the names of 6 columns as a list in the get_y field, it always picks up only two fields; due to this incorrect inference on the dataloader’s part, we have to go through the process of creating a separate label column for getting the dependent variable i.e. y’s values. Next, once we have the dataloader, we can build a classifier model using an LSTM architecture. Also we need to load the language model encodings/embeddings to the classifier once have instantiated it.
现在,我们使用datablocks API创建数据加载器,分别使用x的“ comment_text”字段和y的“ Labels”字段。 如果我们在get_y字段中将6列的名称作为列表提及,则它总是只选择两个字段; 由于数据加载器方面的这种错误推断,我们必须完成创建单独的标签列以获取因变量(即y的值)的过程。 接下来,一旦有了数据加载器,就可以使用LSTM体系结构构建分类器模型。 实例化实例后,我们还需要将语言模型的编码/嵌入物加载到分类器中。
# Create a classifier learner using the dataloader defined above
learn_clf = text_classifier_learner(dls_clf,
# Specify a model architecture for the learner
AWD_LSTM,
# Specify the % in dropout layer for regularization
drop_mult=0.5,
# Specify a metric to evaluate performance while training
metrics = accuracy_multi).to_fp16()
# Load the embeddings from the finetuned language model in our learner object
learn_clf = learn_clf.load_encoder("finetuned_language_model_encoder")
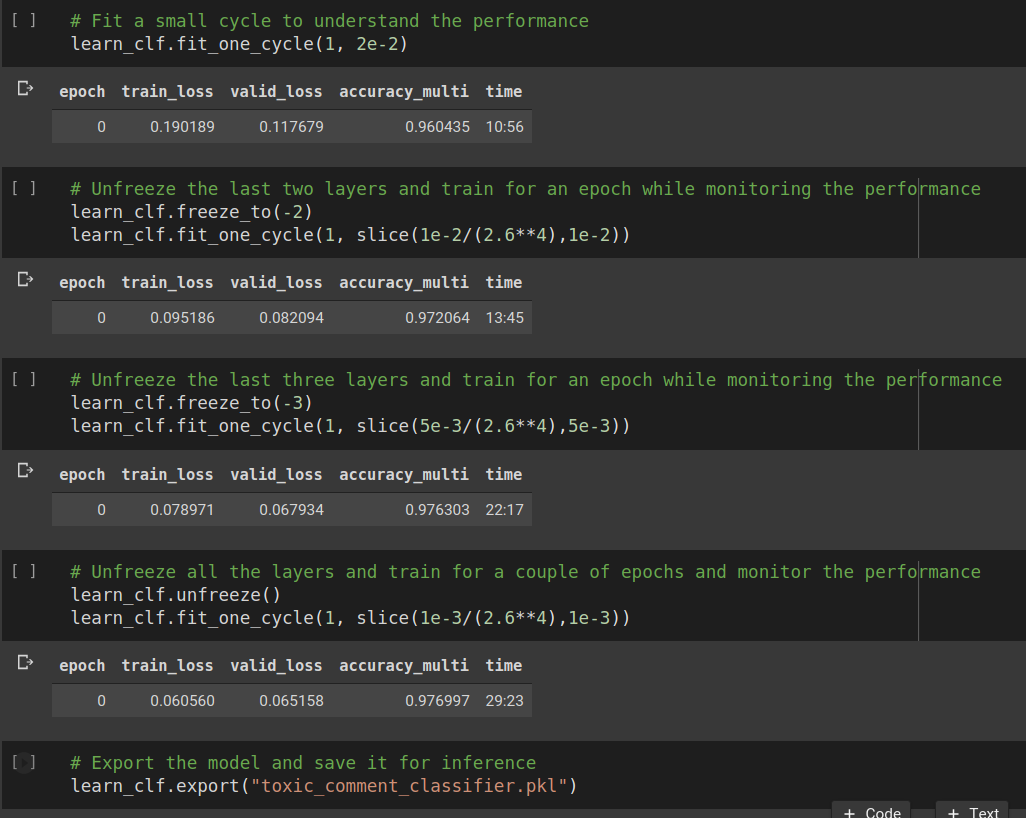
Then we can start training the classifier. Initially, we will keep most of the network except the final FC layer frozen. This means that the back-propagation weight updates will only happen in the penultimate layer. Gradually we will unfreeze the previous layers until eventually we unfreeze the whole network. We do this because if we start with an unfrozen network, it will become difficult for the model to converge quickly to the optimal solution.
然后,我们可以开始训练分类器。 最初,我们将冻结除最终FC层以外的大多数网络。 这意味着反向传播权重更新将仅在倒数第二层中发生。 逐渐地,我们将解冻先前的层,直到最终我们解冻整个网络。 这样做是因为,如果我们从未冻结的网络开始,模型将很难快速收敛到最佳解决方案。
It can be seen that the accuracy has reached a pretty solid 98% buy the end of the training. Since both train and valid loss both are decreasing, we can ideally train for more epochs and keep going but in the interest of time, we shall consider this a good enough score and start with the inference.
可以看出,在培训结束时,准确性已经达到了非常可靠的98%。 由于训练和有效损失都在减少,因此理想情况下,我们可以训练更多的时期并继续前进,但是为了节省时间,我们将认为这是一个足够好的分数,并从推理开始。
进行推论 (Making inferences)
Now that we have a trained model and we’ve stored it as a pkl, we can use it for making predictions on previously unseen i.e. test data.
现在我们有了训练有素的模型,并将其存储为pkl,我们可以将其用于对以前看不见的数据(即测试数据)进行预测。
# Load the trained learner on GPU for fast predictions
learn_clf = load_learner("toxic_comment_classifier.pkl", cpu = False)
# Create a test dataloader for making predictions
tok_inf_df = tokenize_df(test_df, "comment_text")
inf_dl = learn_clf.dls.test_dl(tok_inf_df[0])
# Predict probabilities for all the classes
all_predictions = learn_clf.get_preds(dl = inf_dl, reorder = False)
probs = all_predictions[0].numpy()
We shall first load the model that we just created and trained on the GPU. (Since we have hundreds of thousands of comment texts, CPU inference will take a lot of time). Next we will tokenize the test_df and then pass it through the same transforms that were used for train and validation data to create a dataloader of test comments for inference.
我们将首先加载刚刚创建并在GPU上训练的模型。 (由于我们有成千上万的注释文本,因此CPU推理将花费大量时间)。 接下来,我们将标记化test_df,然后将其通过用于训练和验证数据的相同转换,以创建用于推理的测试注释的数据加载器。
Next we will use the get_preds method for inference and remember to pass the reorder method to False otherwise there’s a random shuffling of the texts that happens which will lead to incorrect order of predictions at the end.
接下来,我们将使用get_preds方法进行推断,并记住将reorder方法传递给False,否则会随机发生文本混排,这将导致最后的预测顺序不正确。
# IDs for the comment_text
idxs = test_df.loc[inf_dl.get_idxs()].id.reset_index(drop = True)
# The order id in test data
indices = inf_dl.get_idxs()
# Create a Predictions dataframe for submission
predictions = pd.DataFrame(all_predictions[0].numpy(), columns = learn_clf.dls.vocab[1])
predictions["id"] = idxs
predictions["order"] = indices
# Curate the dataframe to match the submission format
predictions = predictions.sort_values(by = ["order"])
predictions = predictions[["id", "toxic", "severe_toxic", "obscene", "threat", "insult", "identity_hate"]].reset_index(drop = True)
display_html(predictions.head().to_html(raw = True))
# Save the dataframe
predictions.to_csv("submissions_toxic.csv", index = False)
Finally, we shall format these predictions in the sample_submissions.csv style. So, after predictions, we get a set of 7 values one for each class and the probability of “sober” class is not needed since it was introduced by us as a placeholder. We remove that and get all the ids in proper order. This is how the final submission looks like.
最后,我们将以sample_submissions.csv样式格式化这些预测。 因此,经过预测,我们为每个类别获得一组7个值,并且不需要“清醒”类别的概率,因为它是由我们作为占位符引入的。 我们将其删除,并以正确的顺序获取所有ID。 这就是最终提交的样子。
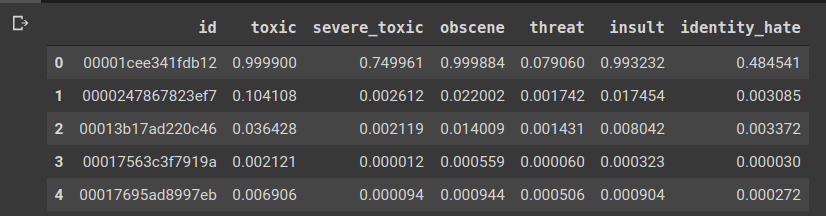
Finally we can submit these predictions using the kaggle API itself. No need to manually go to kaggle and submit the csv file. It could be done simply by this shell command.
最后,我们可以使用kaggle API本身提交这些预测。 无需手动转到kaggle并提交csv文件。 只需使用此shell命令即可完成。
# Submit the predictions to kaggle!kaggle competitions submit -c jigsaw-toxic-comment-classification-challenge -f submissions_toxic.csv -m "First submission for toxic comments classification"
You can change the submissions file name and message as per your own convenience. The final submission score I got is as shown below
您可以根据自己的方便更改提交文件的名称和消息。 我得到的最终提交分数如下所示
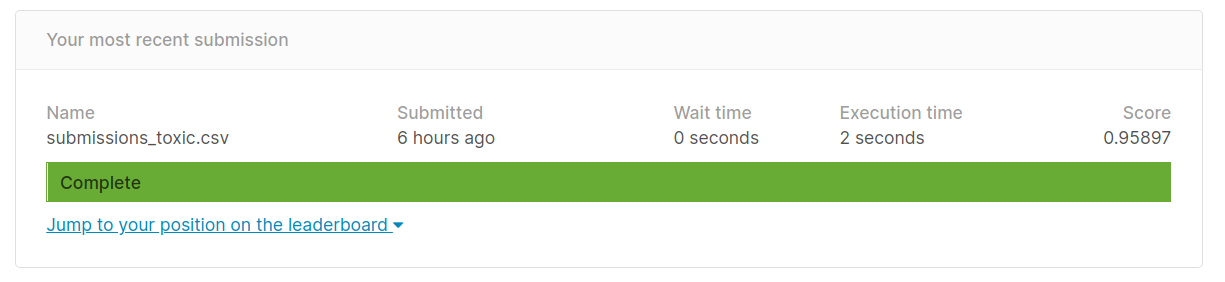
The top score on the leaderboard is around .9885 so our score is somewhat good with such few lines of code and little to no preprocessing. We could’ve removed stopwords, cleaned html tags, tackled punctuation, tuned language model even more or used GloVE or Word2Vec embeddings and went for a complex model like Transformer instead of a simple LSTM. Many people have approached this differently and used some of these techniques to get to such high scores. However, with little effort and using the already implemented fastai library we could get a decent enough score right in our first attempt.
排行榜上的最高分大约为.9885,因此我们的分数在很少的代码行和很少甚至没有预处理的情况下还是不错的。 我们本可以删除更多的停用词,清除html标签,解决标点符号,调整语言模型,或者使用GloVE或Word2Vec嵌入,然后选择像Transformer这样的复杂模型,而不是简单的LSTM。 许多人采用了不同的方法,并使用其中一些技巧来获得如此高的分数。 但是,只需花费很少的精力并使用已经实施的fastai库,我们就可以在第一次尝试中获得足够不错的分数。
On a closing thought, it is worth mentioning that this dataset as annotated by humans may have been mislabelled or there could have been subjective differences between people which is also fair because it’s a very manual and monotonous job. We could aid that process by building a model, then using it to annotate and have humans supervise the annotations to make the process simpler or crowd-source this work to multiple volunteers to get a large corpus of labelled data in a small amount of time. In any case, NLP has become highly instrumental in tackling many language problems in the real world and hope after reading this post, you feel confident to start your journey in the world of text with fastai!
总结一下,值得一提的是,这个由人注释的数据集可能被贴错标签,或者人与人之间可能存在主观差异,这也是公平的,因为这是一项非常手工和单调的工作。 我们可以通过建立模型,然后使用模型进行注释并让人类监督注释来简化该过程,或者将这项工作众包给多个志愿者,以在短时间内获得大量标记数据,从而为该过程提供帮助。 无论如何,NLP已在解决现实世界中的许多语言问题方面发挥了重要作用,希望阅读本文后,您有信心与fastai一起踏入文本世界!
fastai 文本分类