“Things get done only if the data we gather can inform and inspire those in a position to make a difference.” — Mike Schmoker
“只有我们收集的数据能够为人们提供启发和启发,他们才能有所作为。” — 迈克·施默克
Everything has a start and an end, between the initialization and the termination, a process has to take place. Data science is a process that involves numerous steps that enable us to make sense of the data we have. These several steps can turn raw, unorganized, meaningless data into an organized meaningful dataset that tells a story. The number one fact in data is that data is never clean.
一切都有开始和结束,在初始化和终止之间,必须进行一个过程。 数据科学是一个涉及许多步骤的过程,使我们能够理解所拥有的数据。 这几个步骤可以将原始的,无组织的,毫无意义的数据转变为有组织的有意义的数据集,以讲述一个故事。 数据中的第一事实是数据永远不会干净。
Every process always aims at a particular goal. In this case, the data science process always aims at achieving a given goal. For this to take place the following steps have to be followed.
每个过程始终针对特定目标。 在这种情况下,数据科学过程始终旨在实现既定目标。 为此,必须遵循以下步骤。
Set the goal
设定目标
Data scraping
数据抓取
Data cleaning/ cleansing
数据清理/清理
Data exploration
数据探索
Data modeling
资料建模
Data visualization
数据可视化
设定目标。 (Set the goal.)
In every process, a definite goal will always make the process easy to implement and work on. The very first step in the data science process is to identify the problem that requires your solving. With the ultimate goal set, you can identify the kind of data needed in solving the problem.
在每个过程中,都有明确的目标将始终使过程易于实施和进行。 数据科学过程的第一步就是确定需要解决的问题。 通过设定最终目标,您可以确定解决问题所需的数据类型。
An example is if you are trying to understand the causes of climate change the kind of data needed is based on the weather patterns within the past years. It would be unreasonable for the team to gather their data in a financial institution.
例如,如果您试图了解气候变化的原因,则所需的数据类型基于过去几年的天气模式。 对于团队而言,在金融机构中收集数据是不合理的。
The Data Science process is dependent on the ultimate goal set.
数据科学过程取决于最终目标集。
数据交换 (Data Scrapping)
TIt is the process of getting the data. According to techopedia data scraping is defined as a system where a technology extracts data from a particular codebase or program. Data scraping provides results for a variety of uses and automates aspects of data aggregation.
这是获取数据的过程。 根据技术百科,数据抓取被定义为一种系统,其中技术从特定的代码库或程序中提取数据。 数据抓取可为多种用途提供结果,并自动进行数据聚合。
Data scraping, also known as data extraction or web scraping is the process of extracting data from web pages. Scraping tools and software are used to access the Web with the Hypertext Transfer Protocol, collect useful data, and get it extracted as per the requirements. The scrapped information is then saved in a central database or gets downloaded on your hard drive for further uses.
数据抓取(也称为数据提取或Web抓取)是从网页中提取数据的过程。 爬网工具和软件用于通过超文本传输协议访问Web,收集有用的数据,并根据要求将其提取。 然后将报废的信息保存在中央数据库中,或下载到硬盘驱动器上以备将来使用。
The following are tools that will get you started a data scraping process.
以下是可帮助您开始数据抓取过程的工具。
- scraping bee 刮蜂
- Scrapy cra草
- scraper API 刮板API
- Octoparse 八度分析
- Parsel hub Parsel中心
Now that we have scraped the data we need to work on our climate change project. Let us jump on to the next step.
现在我们已经抓取了数据,这是我们开展气候变化项目所需的数据。 让我们跳到下一步。
数据清理/清理。 (Data cleaning/ cleansing.)
This is the third step of the Data Science process it is the most important step when it comes to working with data. Data is always messy and it requires to be cleaned or sorted out. To perform analysis on the data you will need to clean your data to have viable results from the data.
这是数据科学流程的第三步,是处理数据时最重要的一步。 数据总是乱七八糟,需要清理或整理。 要对数据进行分析,您将需要清理数据以从数据中获得可行的结果。
According to Wikipedia Data cleaning is the process of detecting and correcting (or removing) corrupt or inaccurate records from a recordset or a database and refers to identifying incomplete, incorrect, inaccurate, or irrelevant parts of the data and then replacing, modifying, or deleting the dirty or coarse data”
根据Wikipedia的说法,数据清除是从记录集或数据库中检测和纠正(或删除)损坏或不准确的记录的过程,是指识别数据的不完整,不正确,不准确或不相关的部分,然后替换,修改或删除脏数据或粗数据”
Things to look for in the data during the data cleaning process.
在数据清理过程中要在数据中查找的内容。
- Corrupted values. Such as invalid entries. 值损坏。 如无效的条目。
- Timezone differences, perhaps your database doesn’t take into account the different time zones of your users. 时区不同,也许您的数据库没有考虑用户的时区。
- Missing values, there are cases where you will find the null values in a data set. If you decide to work with such data, be sure to get incorrect values. 缺少值,在某些情况下,您会在数据集中找到空值。 如果决定使用此类数据,请确保获取不正确的值。
- Date range errors, in some cases you’ll have data that make no sense at all, such as data registered from before sales started. 日期范围错误,在某些情况下,您将获得毫无意义的数据,例如从销售开始之前注册的数据。
- Repetitive data. 重复数据。
Given that data is never clean, One is always required to sort out the data to eliminate the errors in untidy data. This process will determine the results of the next steps.
鉴于数据永远都不干净,因此始终需要对数据进行分类以消除不整洁数据中的错误。 此过程将确定后续步骤的结果。
数据探索 (Data Exploration)
In simple terms, this is figuring out the relationship in the data. It is getting the general view of the data that will help you in getting the result. It generally refers to the user being able to find his or her way through large amounts of data and gather necessary information.
简单来说,这是在弄清楚数据中的关系。 它正在获得数据的一般视图,这将有助于您获得结果。 它通常指的是用户能够通过大量数据找到自己的方式并收集必要的信息。
Data exploration is an approach that is similar to the data analysis, whereby a data analyst or data scientist uses visual exploration to understand a data-set and the characteristics of the data. These characteristics may include the size of data, completeness of the data, the correctness of the data, the possible relationships among the data elements.
数据探索是一种类似于数据分析的方法,数据分析师或数据科学家使用视觉探索来了解数据集和数据特征。 这些特征可以包括数据的大小,数据的完整性,数据的正确性,数据元素之间的可能关系。
Though might be regarded as similar to Data mining. Let us get the definition of data mining Wikipedia describes data mining as a process of discovering patterns in large data sets involving methods at the intersection of machine learning, statistics, and database systems. Data mining has an overall goal of extracting information (with intelligent methods) from a data set and transforming the information into a comprehensible structure for further use. It is similar to Data exploration.
尽管可能被视为类似于数据挖掘。 让我们得到数据挖掘的定义。维基百科将数据挖掘描述为发现大型数据集中模式的过程,该过程涉及机器学习,统计数据和数据库系统相交处的方法 。 数据挖掘的总体目标是从数据集中提取信息(使用智能方法)并将信息转换为可理解的结构以供进一步使用。 它类似于数据浏览。
Almost to the end.
快要结束了。
资料建模 (Data Modeling)
Data modeling is the representation of data structures in a table. It is a process used to analyze data in order to bring out the relationship between the data. This will help you in defining the data, getting the relationship, ensure consistency and quality of data.
数据建模是表中数据结构的表示。 它是用于分析数据以找出数据之间关系的过程。 这将帮助您定义数据,建立关系,确保数据的一致性和质量。
Data modeling comes in three types.
数据建模分为三种类型。
- Conceptual Data models. This is a model that describes what a system contains. It serves the purpose of defining business concepts and rules. 概念数据模型。 这是一个描述系统包含内容的模型。 它用于定义业务概念和规则的目的。
- Logical Data Models. This is a model that describes how a system should be. It serves the purpose of creating the rules and structures of data. 逻辑数据模型。 此模型描述了系统的状态。 它用于创建数据规则和结构的目的。
- Physical Data Models. This is a model that describes how a system should be implemented its purpose is to implement the database system. 物理数据模型。 该模型描述了应如何实施系统,其目的是实施数据库系统。
Now that we have modeled the data let us now get the meaning out of the data this is where Data visualization comes in this is the last step.
现在我们已经对数据建模了,现在让我们从数据中获取含义,这就是数据可视化的目的,这是最后一步。
数据可视化 (Data visualization)
This is the graphical representation of data. It is a process that involves the use of images to get the relationship in the data. It is the use of visual elements like charts, graphs, timelines, and maps, data visualization is an accessible way to see and understand trends, outliers, correlations, and patterns in the data.ower to discover solutions that have a positive impact in sectors like Medicine, Meteorological, Communication sectors just to mention.
这是数据的图形表示。 这个过程涉及使用图像来获取数据中的关系。 它使用图表,图形,时间线和地图等可视元素,数据可视化是查看和理解数据中趋势,离群值,相关性和模式的一种便捷方式。发现在行业中产生积极影响的解决方案像医学,气象,通讯等领域就更不用说了。
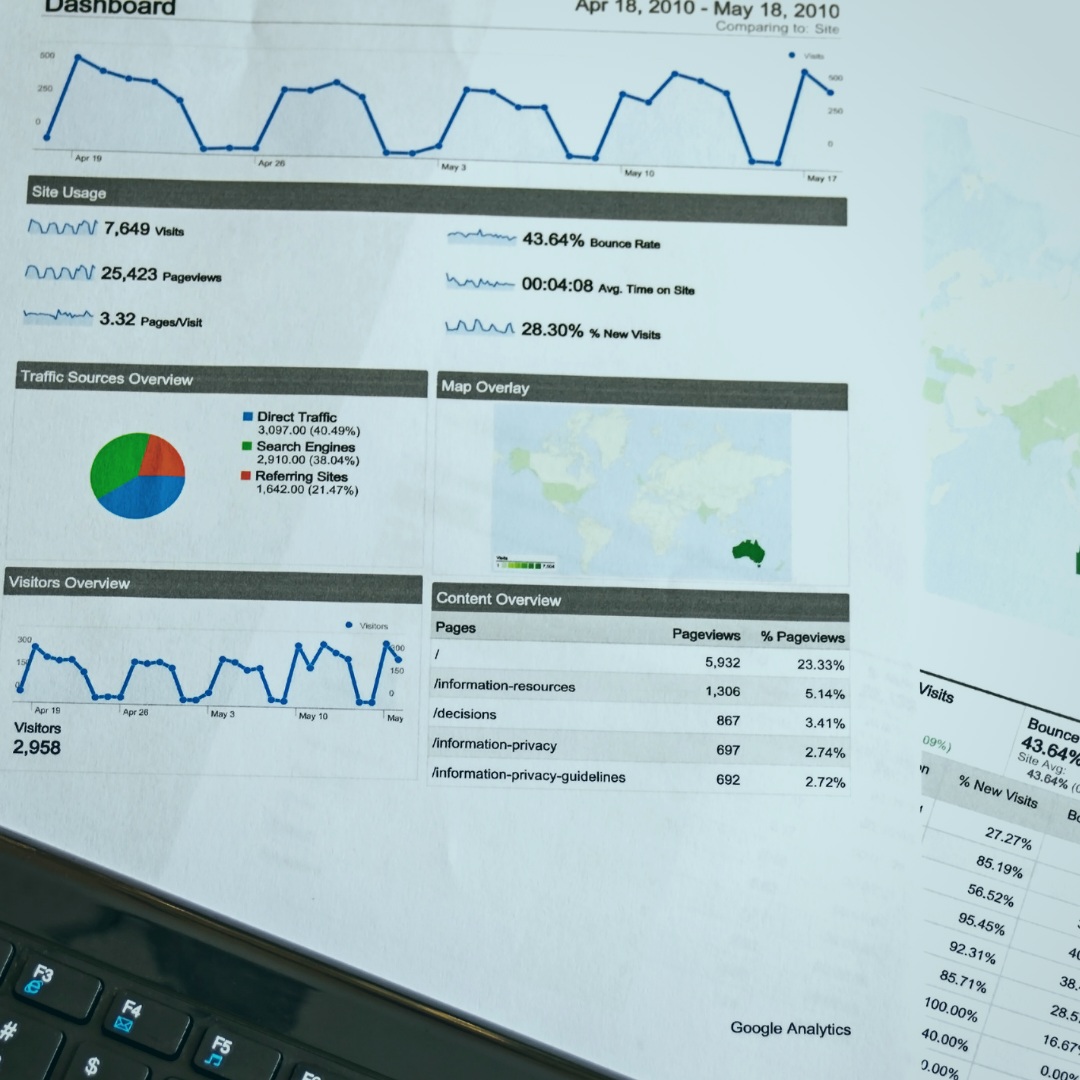
Data visualization will enable you to
数据可视化将使您能够
- Make fast and better decisions based on the observations. 根据观察结果做出更快更好的决策。
- Improved insights. With visualization capturing the relationship in the data is. 改善见解。 通过可视化捕获数据中的关系。
- Discover patterns faster 更快发现图案
- Discover relationships in the data set. 发现数据集中的关系。
- Ask relevant questions. 提出相关问题。
Being able to make sense of the data marks the end of the Data Science Process.
能够理解数据标志着数据科学过程的结束。
摘要 (Summary)
The data science process is a number of steps that aims at getting the meaning out of the data and help us to solve problems. In this case
数据科学过程是许多步骤,旨在从数据中获取含义并帮助我们解决问题。 在这种情况下
“The goal is to turn data into information, and information into insight.” — Carly Fiorina
“目标是将数据转化为信息,并将信息转化为洞察力。” — 卡莉·菲奥莉娜 ( Carly Fiorina)
Having to go through the data science process is a path of discovery that will change the world. With data we have the power to discover solutions that have a positive impact in sectors like Medicine, Meteorological, Communication sectors just to mention.
必须经历数据科学过程是一条将改变世界的发现之路。 借助数据,我们有能力发现对医疗,气象,通信等行业产生积极影响的解决方案,仅此而已。
Hope you liked our article leave a comment a like if you liked our article.
如果您喜欢我们的文章,希望您喜欢我们的文章。
#happylearning #keeplearning
#快乐学习#keeplearning
翻译自: https://medium.com/mldotcareers/the-data-science-process-c38fa1b9f9c1