测试标准 p值
We have all used this is in our stats classes: the null hypothesis is rejected if p<0.05. This short blog is about an explanation of p-value, and how it is connected to the confidence interval and power of a test.
我们都在统计类中使用了此方法:如果p <0.05,则拒绝原假设。 这个简短的博客是关于p值的说明,以及它如何与置信区间和检验功效联系起来。
p-value definition (from stats 101/Wikipedia)
p值定义(来自统计数据101 / Wikipedia)
p-value is a probability of obtaining test results at least as extreme as the results actually observed, under the assumption that the null hypothesis is correct. (difficult to make sense of easily)
p值是在无效假设正确的假设下获得至少与实际观察到的结果一样极端的测试结果的概率。 (难以理解)
Try another method
尝试另一种方法
The null hypothesis is the description of a world we want to check (giving a coupon increases sales or not). In this world, we believe that giving a coupon does not increase sales. We collect samples of data (hoping it is representative of the population) and get our statistic (mean, variance, median, etc). p-value is the probability that the world we wanted to check, throws out the number we got from our sample collection. More explanation in the Figure below.
零假设是对我们要检查的世界的描述(给予优惠券是否会增加销售量)。 在这个世界上,我们相信提供优惠券不会增加销售。 我们收集数据样本(希望它代表总体),并获得统计数据(均值,方差,中位数等)。 p值是我们要检查的世界丢弃从样本集合中获得的数字的概率。 下图中有更多说明。
p-value just tells us if our null hypothesis is true or not so that we can reject it or we fail to reject it. However, we should not be satisfied if p < alpha (generally 0.05) and we should consider Type I and Type II error. Type I error (=alpha) is the probability of rejecting the Null hypothesis when it is true. Type II error is the probability of accepting (or fail to reject) the null hypothesis when it is false.
p值只是告诉我们我们的零假设是否成立,因此我们可以拒绝它或失败。 但是,如果p <alpha(通常为0.05),我们将不满意,我们应该考虑I型和II型误差。 类型I错误(= alpha)是在否定为零时拒绝否定假设的概率。 II型错误是在假假设为假时接受(或未能拒绝)原假设的概率。
Limitations of p-value
p值的局限性
- p-value does not give the probability of how true the null hypothesis was. It just gives a binary decision on if it can be rejected or not p值未给出零假设的真实性的概率。 它只是给出是否可以拒绝的二进制决定
- p-value does not consider how precise the effect is (as it assumes we know the sample size, does not tell much about sample size) p值未考虑效果的精确度(因为它假定我们知道样本量,所以对样本量的了解不多)
Type I error can incur opportunity cost but Type II error could be more harmful. Consider medicine development. Rejecting a drug that could have worked makes the company lose money on its investment. However, accepting a drug that does not work puts peoples’ lives at risk. I remember Type I and Type II error as producers' risk and consumers' risk. Thus the power of a test is also important (power of test = 1-Type II error = probability of rejecting the null hypothesis when it is false). More on the power of a test in Figure 2.
I型错误可能会导致机会成本,但II型错误可能会造成更大的危害。 考虑药物开发。 拒绝可能有效的药物会使该公司的投资蒙受损失。 但是,接受无效的药物会使人们的生命处于危险之中。 我记得类型I和类型II错误是生产者风险和消费者风险。 因此,检验的功效也很重要(检验的功效= 1-Type II错误=当其为假时拒绝原假设的概率)。 有关测试功能的更多信息,请参见图2。
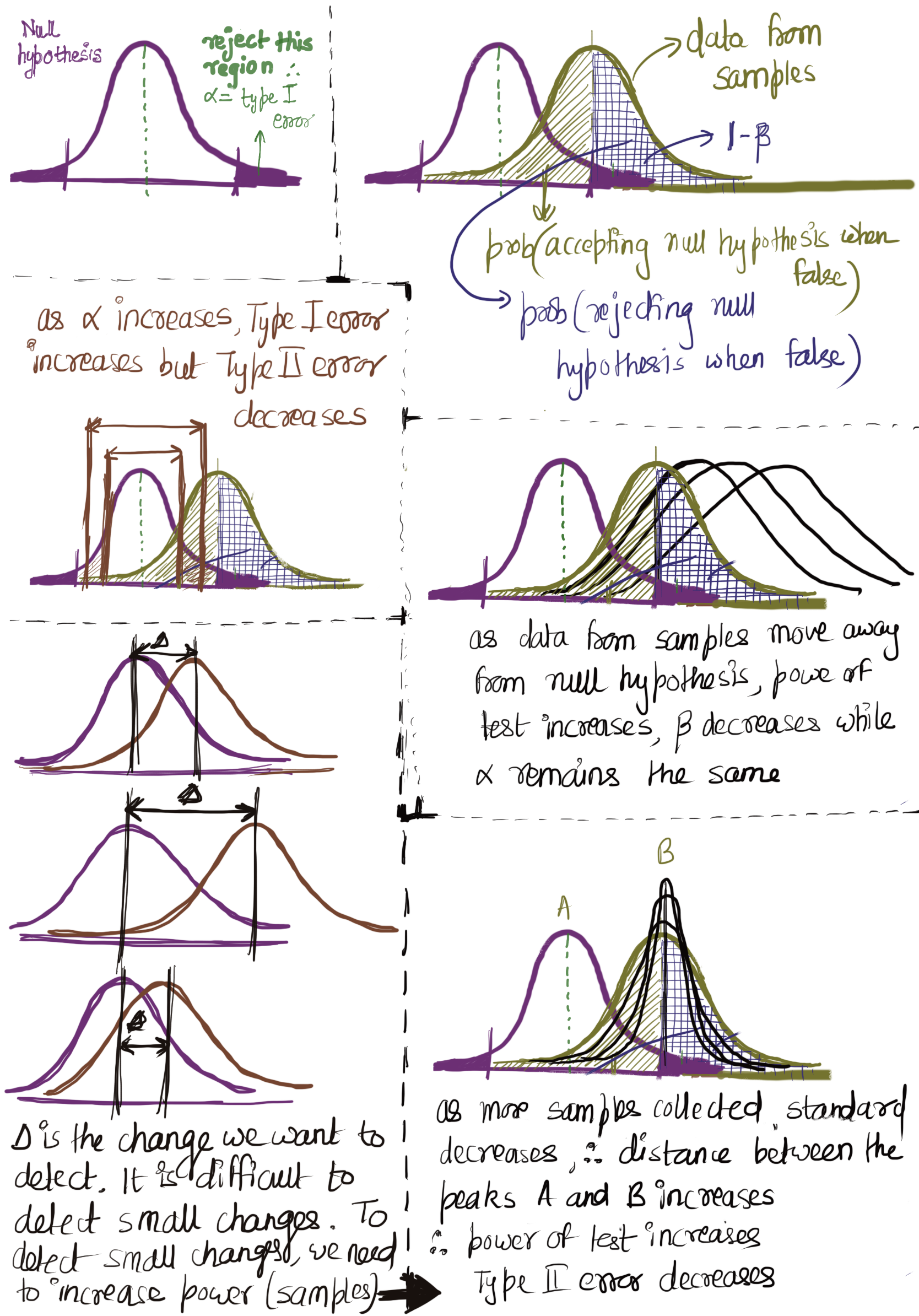
The confidence interval is also calculated from alpha. The confidence interval is interpreted as: (1)if we collect 100 samples (and create a confidence interval for a statistic for each of the sample), the frequency of these confidence intervals which will contain the true value of the statistic (e.g. population mean) tends to be 1-alpha. (2)When we calculate one confidence interval, we say that in future experiments, the true statistic value will lie within that confidence interval (which is equivalent to doing multiple replications now a discussed in (1)).
置信区间也是根据alpha计算得出的。 置信区间解释为:(1)如果我们收集100个样本(并为每个样本创建一个统计的置信区间),则这些置信区间的频率将包含该统计的真实值(例如总体均值) )往往是1- alpha 。 (2)当我们计算一个置信区间时,我们说在将来的实验中,真实的统计值将在该置信区间内(这相当于进行多次复制,这在(1)中已经讨论过)。
翻译自: https://towardsdatascience.com/p-value-and-power-of-a-test-fde61dd8c742
测试标准 p值