收缩数据文件时影响业务
谈生意(Let’s talk business)
Do you know what’s the most valuable resource in the world? Few years back oil was referred as the most valuable resource in the world. But now it’s spot has been taken by data. In today’s information era data has become the single most valuable resource in the world with it’s value increasing with every passing year. For more info read on THE ECONOMIST.
您知道世界上最有价值的资源是什么吗? 几年前,石油被称为世界上最有价值的资源。 但是现在它的位置已经被数据占据了。 在当今的信息时代,数据已变得越来越有价值,它已成为世界上最有价值的单一资源。 欲了解更多信息,请阅读《经济学人》 。
BIG DATA plays a huge role in understanding valuable insights about target demographics and customer preferences and provides a new view into traditional metrics, like sales and marketing information.
BIG DATA在理解有关目标人口统计和客户偏好的宝贵见解方面发挥着巨大作用,并提供了对传统指标(例如销售和营销信息)的新视角。
If you look around yourself today the biggest IT giants be it Google, Facebook, IBM, Microsoft etc, they’re all thriving on data. And with the telecom revolution millions of new users are getting connected to the world of internet every year. Together we create such humongous volume of data that handling it has become a major problem.
如果您今天环顾四周,那么最大的IT巨头,例如Google,Facebook,IBM,Microsoft等,它们都在数据上蒸蒸日上。 随着电信革命的发展,每年都有数以百万计的新用户连接到互联网世界。 我们一起创建了如此庞大的数据量,以至于处理它已成为一个主要问题。
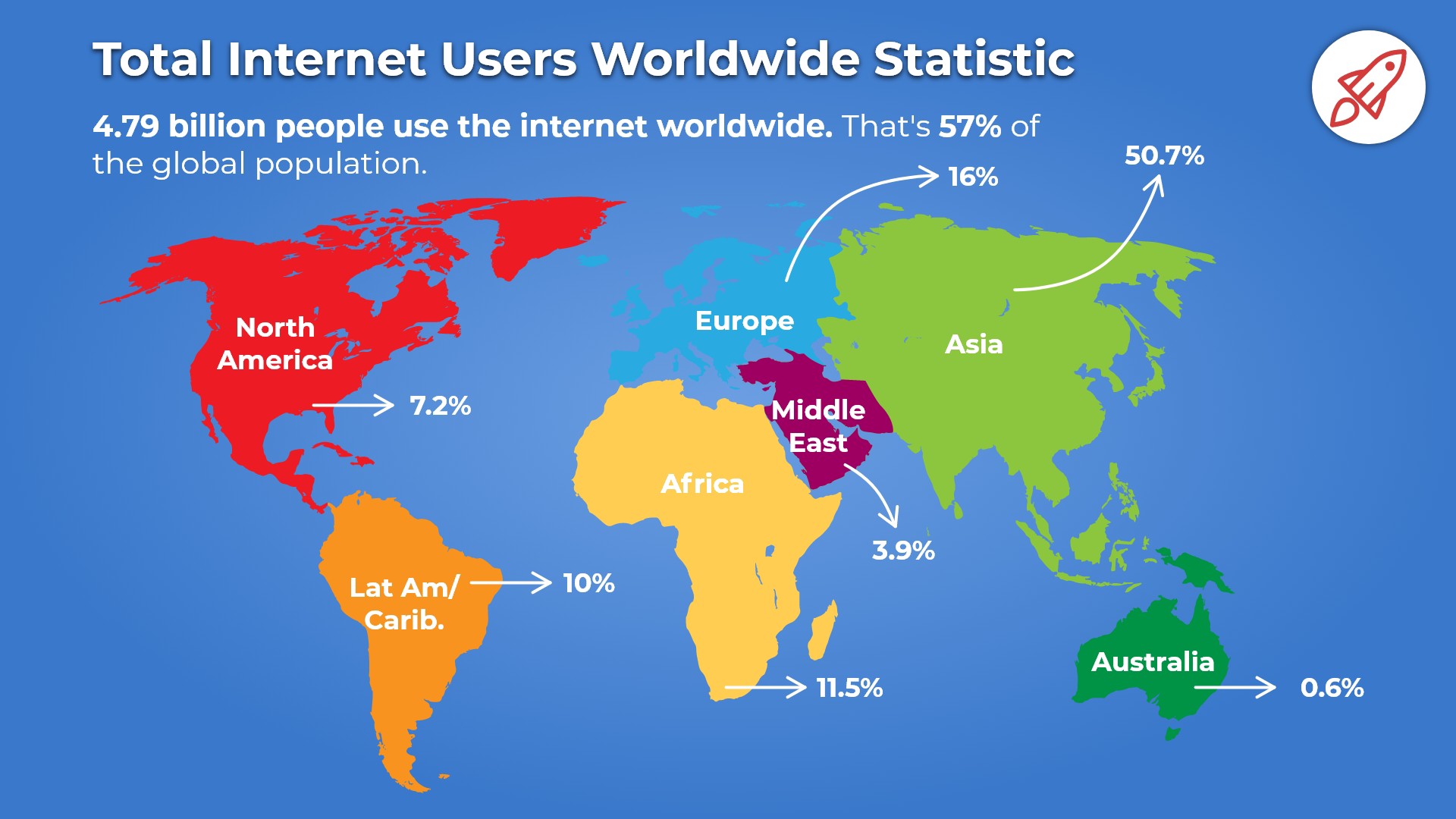
大数据到底是什么? (What exactly is BIG DATA?)
In simple terms it’s massive amount of data being generated on a rapid speed. Many people confuse it with some kind of technology but in fact BIG DATA is a name given to our data problem. The go-to definition: Big data is data that contains greater variety arriving in increasing volumes and with ever-higher velocity. It’s too large and complex for processing by traditional database management tools.
简单来说,就是快速生成大量数据。 许多人将其与某种技术混淆,但实际上BIG DATA是解决我们数据问题的名称。 首选定义:大数据是包含更多种类的数据,这些种类以越来越大的速度和越来越高的速度到达。 对于传统的数据库管理工具而言,它太大且太复杂。
大数据的三大诉求 (The Three Vs of BIG DATA)
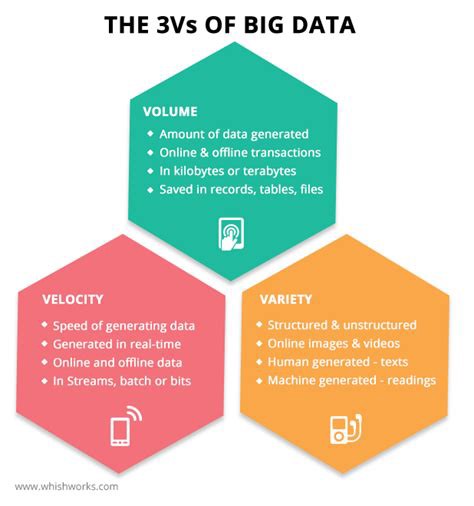
This was introduced by Doug Laney(an analyst at Gartner) in 2001:
这是2001年由Doug Laney(Gartner的分析师)介绍的:
Volume
卷
It refers to the amount of data. It’s generated from multiple sources like social media platforms (such as Facebook, Twitter, Instagram), e-mail service platforms (like G-mail, Outlook.com, Yahoo mail) and so on. For some organizations, this might be tens of terabytes of data. For others, it may be hundreds of petabytes. The problem is it’s so huge that it even dwarfs the largest hardware available for storage(100TB Nimbus Data ExaDrive DC100).
它指的是数据量。 它由多种来源生成,例如社交媒体平台(例如Facebook,Twitter,Instagram),电子邮件服务平台(例如G-mail,Outlook.com,Yahoo邮件)等等。 对于某些组织,这可能是数十兆字节的数据。 对于其他人,可能是数百PB。 问题在于它是如此之大,以至于它甚至使可用于存储的最大硬件( 100TB Nimbus Data ExaDrive DC100 )相形见war 。
2. Velocity
2.速度
Velocity refers to the speed at which the data is generated. Normally, the highest velocity of data streams directly into memory versus being written to disk.
速度是指数据生成的速度。 通常,与直接写入磁盘相比,数据流直接进入内存的速度最高。
3. Variety
3.品种
Variety refers to the many types of data that are available i.e. structured, unstructured and semi-structured. With the rise of big data, data comes in new unstructured data types. Unstructured and semi-structured data types, such as text, audio, and video, require additional preprocessing to derive meaning and support metadata.
多样性是指可用的多种数据类型,即结构化,非结构化和半结构化。 随着大数据的兴起,数据进入了新的非结构化数据类型。 非结构化和半结构化的数据类型,例如文本,音频和视频,需要进行额外的预处理以导出含义并支持元数据。
Apart from these there are few other important characteristics of BIG DATA two of which are:
除了这些以外,BIG DATA的其他重要特征很少,其中两个是:
4. Veracity
4.准确性
How truthful is your data — and how much can you rely on it? Veracity is all about making sure the data you acquire is accurate, which requires processes to keep the bad data from accumulating in your systems.
您的数据有多真实?您可以依靠多少数据? 准确性就是确保您获取的数据准确无误,这需要流程来防止不良数据在您的系统中累积。
5. Value
5.价值
At the end everything folds to the fact that how valuable is your data for your organization’s business. These massive volumes of data can be used to address business problems you wouldn’t have been able to tackle before. Your investment in big data pays off when you analyze and act on your data.
最后,一切都折叠成一个事实,即您的数据对组织业务的价值。 这些海量数据可用于解决您以前无法解决的业务问题。 当您对数据进行分析并采取行动时,您在大数据上的投资将获得回报。
大数据的类型(品种) (Types of BIG DATA (Variety))
Structured Data: As the name suggests the data is structured or formatted in a consistent order that’s easily accessible for analysis.
结构化数据:顾名思义,数据是以一致的顺序进行结构化或格式化的,易于分析。
Unstructured Data: This type of data has no defined structure and hence cannot be stored in traditional relational database or RDBMS. It mainly comes from documents, social media feeds, pictures, videos and sensors.
非结构化数据:这种类型的数据没有定义的结构,因此无法存储在传统的关系数据库或RDBMS中。 它主要来自文档,社交媒体供稿,图片,视频和传感器。
To read about the importance of unstructured data visit forbes.com
要了解非结构化数据的重要性,请访问forbes.com。
3. Semi-structured Data: It’s got flavors of both structured and unstructured data i.e. it has some consistency but doesn’t sticks to a rigid structure. It’s easier to analyze as compared to unstructured data.
3.半结构化数据:它具有结构化和非结构化数据的两种风格,即,虽然具有一定的一致性,但不坚持严格的结构。 与非结构化数据相比,它更易于分析。
Hadoop:大数据工具 (Hadoop: A BIG DATA Tool)
Somewhere around 2005, people began to notice the sheer amount of data users generated through social media, emails, blogs, websites and other online services. Hadoop (an open-source framework created specifically to store and analyze big data sets) was developed that same year.
在2005年左右的某个地方,人们开始注意到通过社交媒体,电子邮件,博客,网站和其他在线服务生成的大量数据用户。 Hadoop (专门创建用于存储和分析大数据集的开源框架)于同年开发。
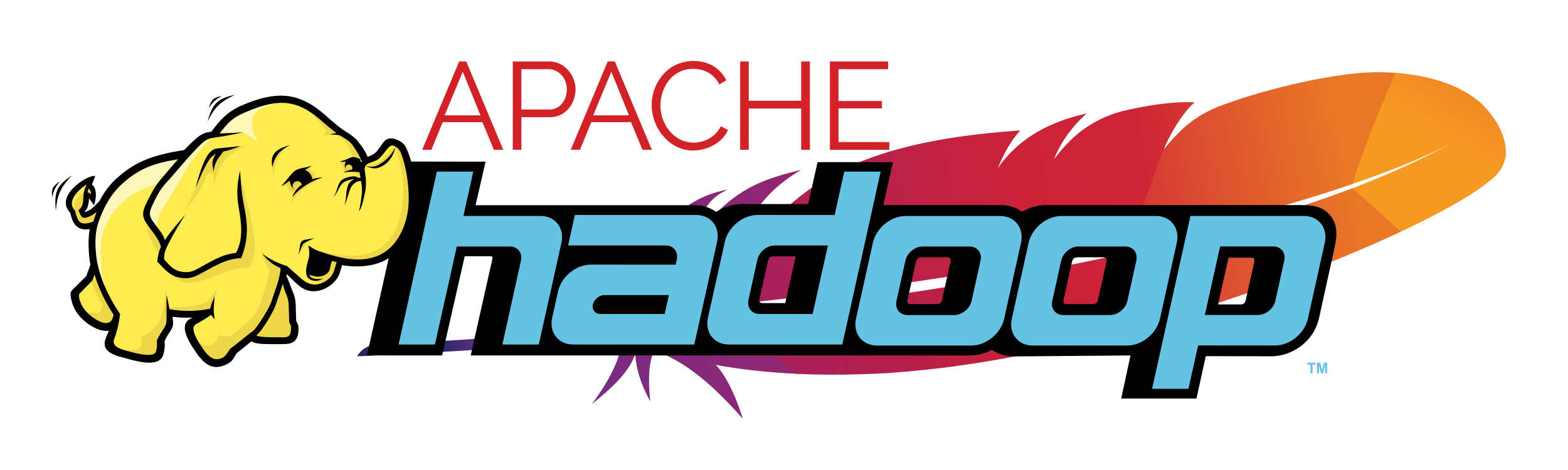
The development of open-source frameworks, such as Hadoop was essential for the growth of big data because they make big data easier to work with and cheaper to store. Since then, the volume of big data has only skyrocketed.
诸如Hadoop之类的开源框架的发展对于大数据的增长至关重要,因为它们使大数据更易于使用且存储成本更低。 从那时起,大数据量才激增。
The massive data collected and generated through the IoT and Machine Learning have added more to this. Cloud computing has opened up further many doors of possibilities for BIG DATA analytics.
通过IoT和机器学习收集和生成的海量数据为这增加了更多。 云计算为大数据分析打开了更多的可能性之门。
分布式存储:大数据量和速度问题的解决方案 (Distributed Storage: A solution to BIG DATA volume and velocity problem)
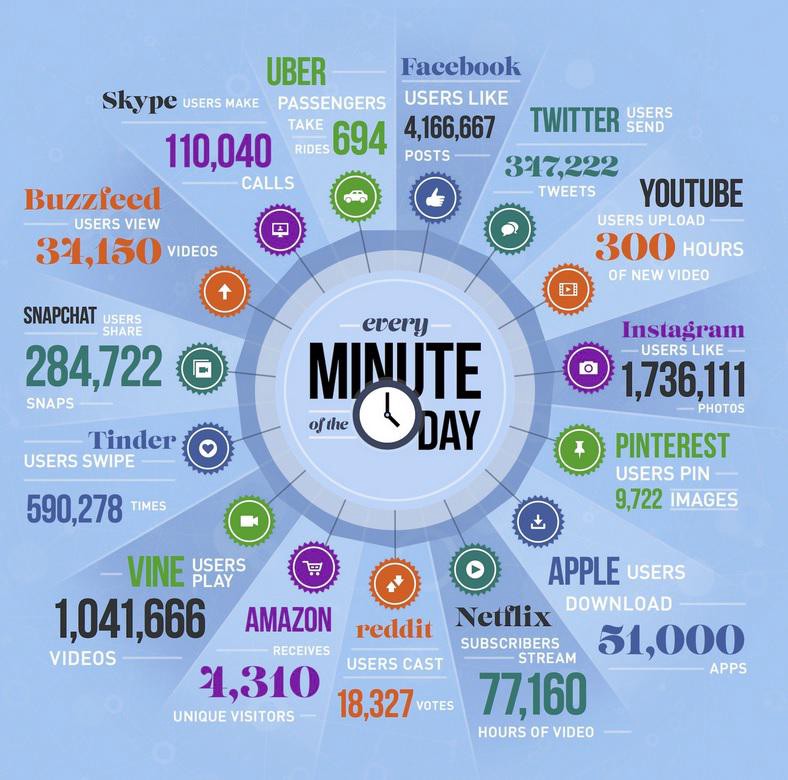
1.7MB of data is created every second by every person during 2020. (Source: Domo)
到2020年,每个人每秒将创建1.7MB数据。 (来源:Domo)
In the last two years alone, the astonishing 90% of the world’s data has been created. (Source: IORG)
仅在过去的两年中,就已经创造了全球90%的惊人数据。 (来源:IORG)
2.5 quintillion bytes of data are produced by humans every day. (Source: Social Media Today)
每天人类产生2.5亿字节的数据。 (来源:今日社交媒体)
463 exabytes of data will be generated each day by humans as of 2025. (Source: Raconteur)
截至2025年,人类每天将产生463艾字节的数据。 (来源:Raconteur)
95 million photos and videos are shared every day on Instagram.
每天在Instagram上分享9500万张照片和视频。
By the end of 2020, 44 zettabytes will make up the entire digital universe. (Source: Raconteur)
到2020年底,整个数字世界将构成44 ZB 。 (来源:Raconteur)
Every day, 306.4 billion emails are sent, and 5 million Tweets are made. (Source: Internet Live Stats)
每天发送3064亿封电子邮件,并发送500万条推文。 (来源:Internet Live Stats)
And the list goes on…
而这样的例子不胜枚举…
That’s not even all of the data that we create every day. All this data, it’s the lifeline to the IT giants. But storing such humongous volume of data is extremely challenging. And equally challenging is the velocity problem i.e. the data is generated at a much faster rate than the speed at which it’s stored. This is where Distributed Storage comes into play. It’s basically:
这还不是我们每天创建的所有数据。 所有这些数据,是IT巨头的命脉。 但是存储如此庞大的数据量是极具挑战性的。 同样具有挑战性的是速度问题,即以比存储数据的速度快得多的速度生成数据。 这就是分布式存储发挥作用的地方。 基本上是:
“Storing data on a multitude of standard servers, which behave as one storage system although data is distributed between these servers.”
“将数据存储在许多标准服务器上,这些标准服务器充当一个存储系统,尽管数据分布在这些服务器之间。”
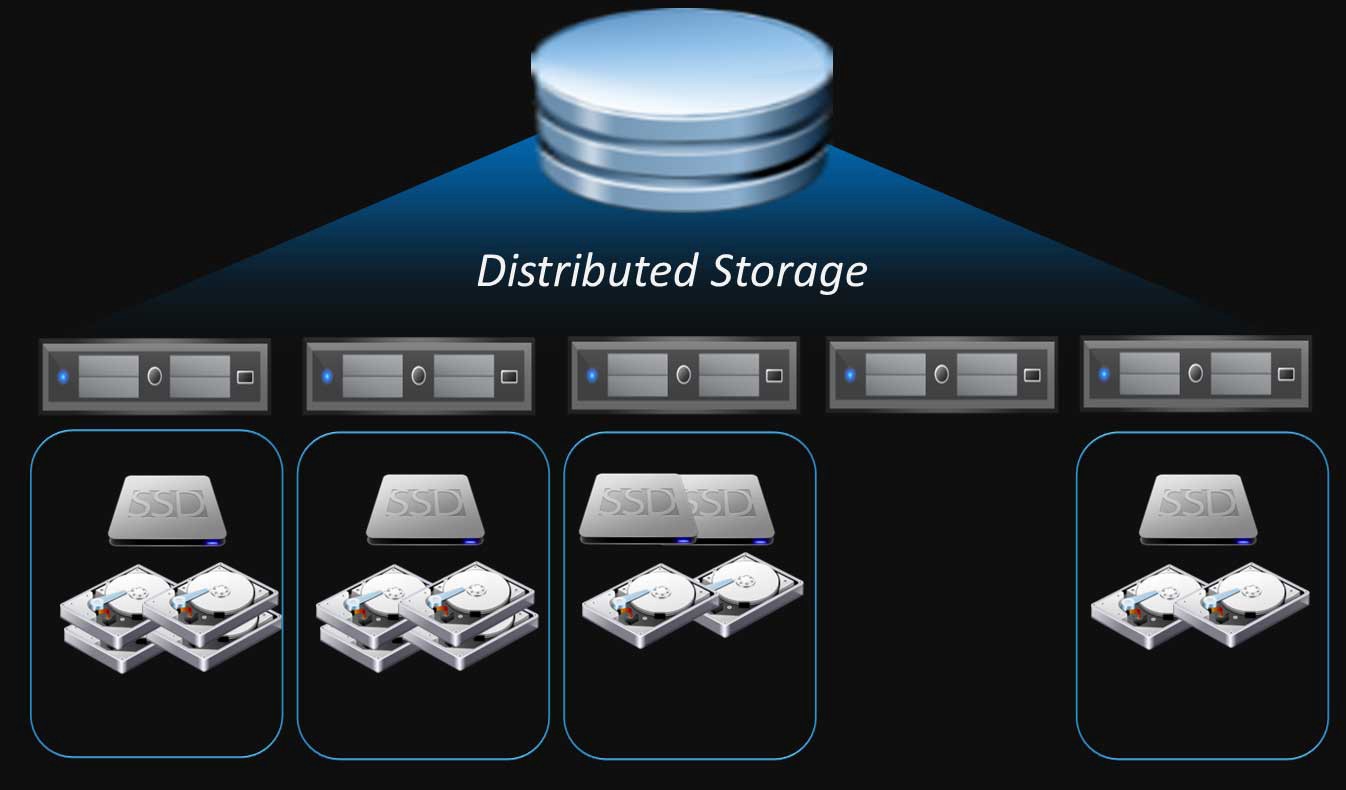
Whatever data comes to the frontend server (Master Node) is sliced equally and sent to the backend servers (Slave Nodes) for storing them. This not only solves the volume problem but also the velocity problem as I/O data transfer takes places in parallel among the slave nodes.
不论到达前端服务器(主节点)的任何数据均被均分,然后发送到后端服务器(从节点)进行存储。 这不仅解决了体积问题,还解决了速度问题,因为I / O数据传输在从属节点之间并行发生。
Let’s take an example to better understand this. Facebook receives nearly 500TB of data each day and the largest available storage is only 100TB in size. So by distributed storage model we’ll club together 5 systems each with hard disks of 100TB with a master node. Now master node will split/slice the data into 5 equal parts and send it to each node. This solves the volume problem. Now, say it takes a minute to store 1GB of data on the hard disk. So, it’ll
让我们举个例子来更好地理解这一点。 Facebook每天接收近500 TB的数据,而最大的可用存储空间仅为100 TB。 因此,通过分布式存储模型,我们将把5个系统与每个100TB硬盘以及一个主节点组合在一起。 现在,主节点会将数据拆分/切片为5个相等的部分,并将其发送到每个节点。 这样解决了体积问题。 现在,假设要花1分钟的时间在硬盘上存储1GB的数据。 所以,它将
Now, say each system takes an hour to store 1TB of data (practically it’ll take days). So, 100TB of data will be stored in 100 hrs and since all 5 systems are running parallelly it’ll take the same time to store 500TB of data. But say, if we double the number of slave nodes then each hard disk will only have to store 50TB of data and it’ll take half the time.
现在,假设每个系统需要一个小时来存储1TB数据(实际上需要几天时间)。 因此,100 TB的数据将在100个小时内存储,并且由于所有5个系统并行运行,因此将需要花费相同的时间来存储500 TB的数据。 但要说的是,如果我们将从属节点的数量加倍,那么每个硬盘将只需要存储50TB的数据,这将花费一半的时间。
This method is not only efficient but also scalable and the best part is that the more you increase the number of slave nodes the faster I/O transfer rate becomes.
此方法不仅高效,而且可扩展,而且最好的方面是,增加从属节点的数量越多,I / O传输速率就变得越快。
Let’s see thorough few examples how the companies leverage the benefits of BIG DATA ANALYTICS:
让我们看几个完整的例子,这些公司如何利用BIG DATA ANALYTICS的优势:
谷歌搜索 (Google Search)
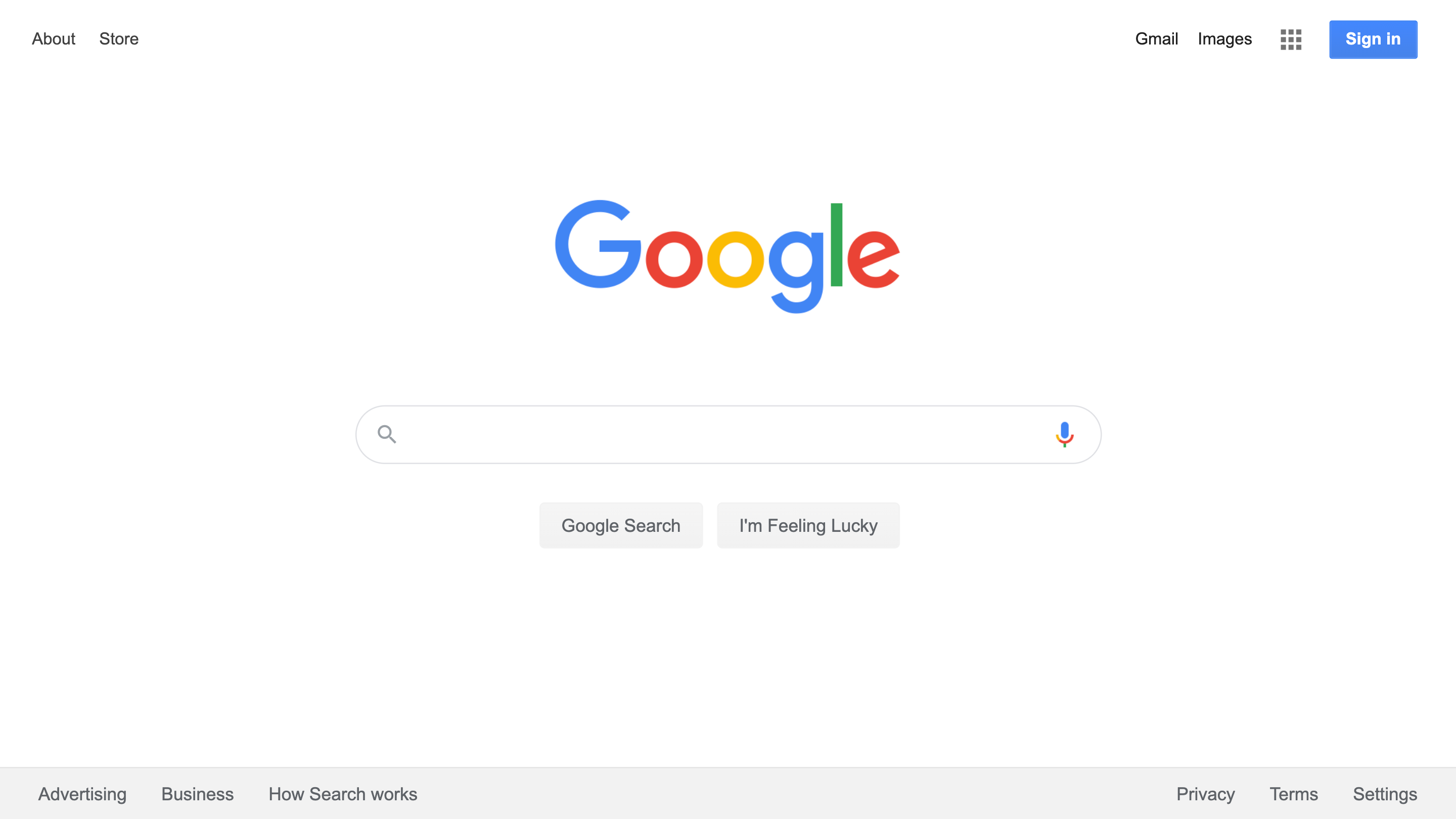
Without a doubt Google is an expert in BIG DATA. Have you ever wondered how Google search gives you search results so fast and accurate considering the fact that there are billions of webpages to scan? The answer is BIG DATA ANALYTICS. Google uses Big Data tools and techniques to understand our requirements based on several parameters like search history, locations, trends etc. Then all this is processed through an algorithm and displayed to the user in sorted manner according to the relevancy.
毫无疑问,Google是BIG DATA的专家。 考虑到有数十亿个网页需要扫描,您是否曾经想过Google搜索如何为您提供如此快速和准确的搜索结果? 答案是大数据分析。 Google使用大数据工具和技术根据几个参数(例如搜索历史,位置,趋势等)来了解我们的要求。然后通过算法对所有这些信息进行处理,并根据相关性以排序的方式显示给用户。
Here’s an infographic by Vertical Measures.
亚马孙 (Amazon)
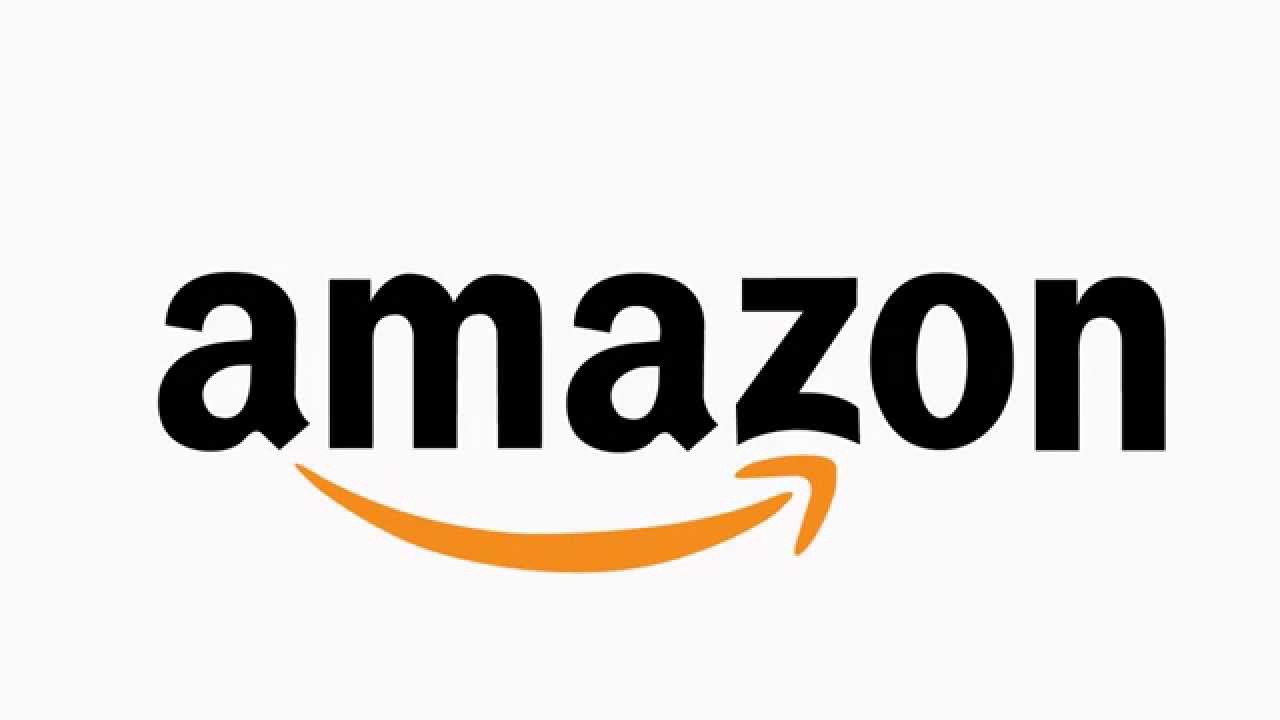
Amazon houses the widest variety of goods and services and has thrived on it. That’s what the arrow in it’s logo represents “everything from A to Z”. But when customers are exposed to such huge range of options they often feel overwhelmed. They get access to more choices but have poor insights and become confused about what to buy and what not to. To tackle this problem amazon uses the BIG DATA that has been collected from customer’s browsing history to fine-tune it’s recommendation engine that provides personalized product recommendations to each customer.
亚马逊拥有种类繁多的商品和服务,并在此基础上蓬勃发展。 这就是徽标中的箭头代表“从A到Z的一切”。 但是,当客户面临如此众多的选择时,他们通常会感到不知所措。 他们可以获得更多选择,但洞察力很差,对购买什么和不购买什么感到困惑。 为了解决此问题,亚马逊使用从客户的浏览历史记录中收集的大数据来微调其推荐引擎,该引擎为每个客户提供个性化的产品推荐。
That’s all!
就这样!
Hope you enjoyed the blog!
希望您喜欢这个博客!
Bye bye… See you soon!
再见……再见!
翻译自: https://medium.com/@ankitkumarakt746/big-data-and-its-impact-on-it-business-6b8750132de4
收缩数据文件时影响业务