无人驾驶汽车 (Self-Driving Cars)
“If you recognize that self-driving cars are going to prevent car accidents, AI will be responsible for reducing one of the leading causes of death in the world.” — Mark Zuckerberg
“如果您意识到自动驾驶汽车将预防车祸,那么人工智能将负责减少世界上主要的死亡原因之一。” - 马克·扎克伯格
Whenever we think about the AI world, the auto industry immediately comes to our minds. Self-driving cars is one of the fascinating future which does not seem to be a distant reality. We just sit inside the car and watch a movie while it takes you to its destination.
每当我们想到AI世界时,就会立即想到汽车行业。 自动驾驶汽车是令人着迷的未来之一,这似乎并不是遥不可及的现实。 我们只是坐在车内,一边看电影,一边将您带到目的地。
But is it that easy that cars can drive fully autonomous and pay attention to all contexts in an environment? In the past few years, many papers have been published to predict the possible future trajectories of cars and pedestrians that are socially acceptable.
但是,汽车能够完全自动驾驶并关注环境中的所有环境是否容易呢? 在过去的几年中,已经发表了许多论文来预测社会上可以接受的汽车和行人的未来轨迹。
Question: What will be one of the biggest challenges of self-driving cars?
问题 :自动驾驶汽车的最大挑战是什么?
Answer: Understanding pedestrian behavior and their future trajectory.
答案 :了解行人的行为及其未来轨迹。
Human motion can be described as multimodal i.e. humans have the possibility to move in multiple directions at any given instant of time. And this behavior is one of the biggest challenges of self-driving cars. Since they have to navigate through a human-centric world.
人体运动可以描述为多模式的,即人体可以在任何给定的瞬间沿多个方向移动。 这种行为是自动驾驶汽车的最大挑战之一。 因为他们必须穿越以人为本的世界 。
In this first part, I will discuss three papers in brief whose main aim is to predict future possible trajectories of pedestrians.
在第一部分中,我将简要讨论三篇论文,其主要目的是预测行人的未来可能轨迹。
社交GAN (Social GAN)
This is one of the initial papers that has stated using GAN to predict the possible trajectories of humans.
这是使用GAN预测人类可能轨迹的最初论文之一。
This paper tries to solve the problem by predicting the socially plausible future trajectories of humans that will help self-driving cars in making the right decision.
本文试图通过预测人类在社会上可行的未来轨迹来解决该问题,这将有助于自动驾驶汽车做出正确的决定。
目标: (Aim:)
The paper aims at resolving two major challenges:
本文旨在解决两个主要挑战:
- To have a computationally efficient interaction model among all people in a scene. 在场景中的所有人之间建立计算有效的交互模型。
- To learn and produce multiple trajectories that are socially acceptable. 学习并产生社会上可接受的多种轨迹。
方法 (Method)
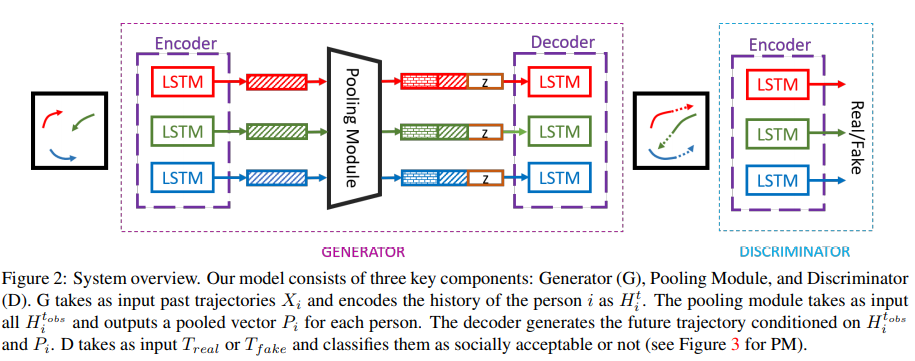
This paper presented a GAN based encoder-decoder network that consists of a LSTM network for each person and a Pooling module that models interactions among them.
本文提出了一个基于GAN的编解码器网络,该网络由每个人的LSTM网络和对它们之间的交互进行建模的Pooling模块组成。
The whole model (shown in Fig 1.) can be represented by 3 different components:
整个模型(如图1所示)。 可以由3个不同的组件表示:
发电机 (Generator)
The Generator consists of an encoder and a decoder. For each person, the encoder takes input as X_i. It embeds the location of each person and provides it as a fixed-length vector to the LSTM cell at time t.
生成器由编码器和解码器组成。 对于每个人,编码器将输入作为X_i。 它嵌入每个人的位置,并在时间t将其作为固定长度的向量提供给LSTM单元。
The LSTM weights were shared among all the people in a scene that will help with the pooling module to develop interaction among people.
LSTM权重在场景中的所有人之间共享,这将有助于合并模块发展人与人之间的互动。
Unlike prior work, this paper has used 2 following approaches:
与先前的工作不同,本文使用了以下两种方法:
a) For an easy training process during backpropagation, instead of predicting the bivariate Gaussian distribution, the decoder directly produces (x, y) coordinates of the person’s location.
a)为了在反向传播过程中进行简单的训练,解码器无需预测双变量高斯分布,而是直接生成人所在位置的(x,y)坐标。
b) Instead of providing the social context directly as the input of the encoder, they have provided it once as input to the decoder. This led to an increase in speed to 16x times.
b)他们没有将社交环境直接提供为编码器的输入,而是将其一次提供为解码器的输入。 这导致速度提高到16倍。
鉴别器 (Discriminator)
The discriminator consists of an encoder with LSTM layers for each person. The idea of this discriminator is to distinguish the real trajectories with fake ones.
鉴别器由每个人的带有LSTM层的编码器组成。 这种鉴别器的思想是用假的伪造来区分真实的弹道。
Ideally, it should classify the trajectories as “fake” if they are not socially acceptable or possible.
理想情况下,如果轨迹在社会上不可接受或不可能,则应将其归类为“伪造” 。
合并模块 (Pooling Module)
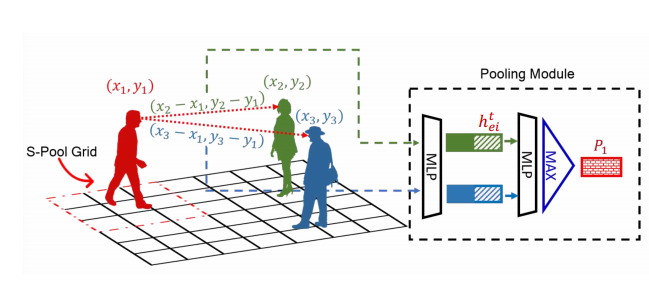
The basic idea of this approach is shown in Fig2. This method computes the relative position of the person 1 (represented in red) and all other people (represented in blue and green). It is then concatenated with hidden states and processed independently through MLP (multi-layer perception).
这种方法的基本思想如图2所示。 此方法计算人员1(以红色表示)与所有其他人(以蓝色和绿色表示)的相对位置。 然后将其与隐藏状态连接起来,并通过MLP(多层感知)进行独立处理。
Eventually, each element is sequentially pooled to compute a person’s 1 pooling vector P1.
最终,每个元素被顺序合并以计算一个人的1个合并向量P1。
This method diminishes the limitation of considering people inside a particular grid (S-Pool Grid in Fig 2.)
这种方法减少了考虑在特定网格(图2中的S-Pool网格 )内部人员的局限性。
损失 (Losses)
3 different losses are used in this paper:
本文使用了3种不同的损耗:
Adversarial loss: This loss is a typical GAN loss that helps in differentiating real and fake trajectories.
对抗性损失 :这种损失是典型的GAN损失,有助于区分真实和伪造的轨迹。
L2 Loss: This loss takes the distance between the predicted and ground-truth trajectory and measures how far the generated samples are from real ones.
L2损失 :该损失占用了预测轨迹与地面真实轨迹之间的距离,并测量了所生成的样本与真实样本之间的距离。
Variety Loss: This loss helps in generating multiple different trajectories i.e. multimodal trajectories. The idea is very simple, for each input, N different possible outcomes are predicted by randomly sampling ‘z’ from N(0, 1). Eventually, select the best trajectory that has a minimum L2 value.
品种损失 :这种损失有助于产生多种不同的轨迹,即多峰轨迹。 这个想法非常简单,对于每个输入,通过从N(0,1)中随机采样“ z”来预测N个不同的可能结果。 最终,选择具有最小L2值的最佳轨迹。

苏菲:细心的GAN (Sophie: An Attentive GAN)
This paper has extended the work of Social GAN and tried to predict a future path for an agent with the help of both physical and social information.
本文扩展了Social GAN的工作,并尝试借助物理和社交信息来预测代理的未来之路。
Although the aim is still the same as Social GAN, this paper has added scenic information too with the help of images of each frame.
尽管目标仍然与Social GAN相同,但本文还借助每帧图像添加了风景信息。
The network learns two types of attention components:
网络学习两种类型的注意力组件:
Physical Attention: This attention component helps in paying attention and processing the local and global spatial information of the surrounding. As mentioned in the paper: “For example, when reaching a curved path, we focus more on the curve rather than other constraints in the environment”
身体注意 :此注意组件有助于关注并处理周围的局部和全局空间信息。 如论文所述 : “例如,当到达弯曲路径时,我们更多地关注曲线而不是环境中的其他约束”
Social Attention: In this component, the idea is to give more attention to the movement and decisions of other agents in the surrounding environment. For example: “when walking in a corridor, we pay more attention to people in front of us rather than the ones behind us”
社会关注 :在此组件中,其想法是更加关注周围环境中其他代理的移动和决策。 例如:“ 在走廊上行走时,我们会更加关注前方的人,而不是身后的人 ”
方法 (Method)
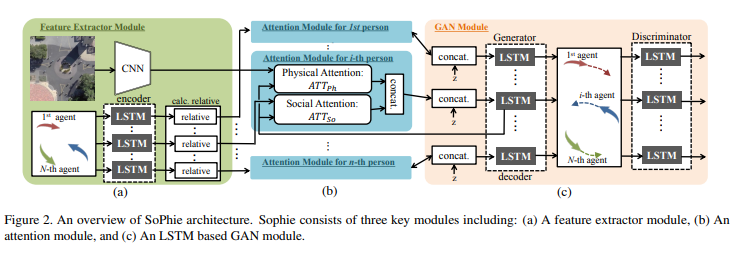
This paper’s proposed approach has been divided into 3 modules (as shown in Fig3.).
本文提出的方法已被划分成3个模块(如图图三)。
特征提取器模块 (Feature Extractor module)
This module extracts features from the input in 2 different forms, first as an image for each frame and second as a state of each agent for each frame at a time ‘t’.
该模块提取物在2组不同的形式输入功能, 首先为每个帧的图像,并且第二 ,因为每个代理在时间t的每个帧的状态。
To extract visual features from the image, they have used VGGnet-19 as a CNN network. The weights of this network are initialized by ImageNet. And to extract features from the past trajectory of all agents, they use a similar approach as Social GAN and use LSTM as an encoder.
为了从图像中提取视觉特征,他们将VGGnet-19用作CNN网络。 该网络的权重由ImageNet初始化。 为了从所有特工的过去轨迹中提取特征,他们使用与Social GAN类似的方法,并使用LSTM作为编码器。
To understand the interaction between each agent and capture the influence of each agent trajectory on another agent, the pooling module was used in Social GAN. This paper has mentioned 2 limitations with that method:
为了了解每个代理之间的交互并捕获每个代理轨迹对另一个代理的影响,在Social GAN中使用了合并模块。 本文提到了该方法的两个局限性:
- Max function may discard the important features of the inputs as they might lose their uniqueness 最大功能可能会丢弃输入的重要特征,因为它们可能会失去其唯一性
- After the max operation, all the trajectories are concatenated which may lead to having an identical join feature representation. 在max操作之后,所有轨迹被级联,这可能导致具有相同的联接特征表示。
Because of these limitations, they define an ordering structure. In this, they use sort as permutation invariant function instead of max(used in Social GAN). They sort the agents by calculating the euclidean distance between the target agent and other agents.
由于这些限制,它们定义了排序结构。 在这种情况下,他们使用sort作为置换不变函数,而不是max (在Social GAN中使用)。 他们通过计算目标代理与其他代理之间的欧式距离来对代理进行排序。
注意模块 (Attention module)
With the help of physical or social attention, this module helps in highlighting the important information of the input for the next module.
在身体或社会关注的帮助下,该模块有助于突出显示下一个模块输入的重要信息。
The idea is, as humans pay more attention to certain obstacles or objects in an environment like upcoming turns or people walking towards them, a similar kind of attention needs to be learned.
这个想法是,当人们在即将到来的转弯或人们朝他们走去的环境中更加关注某些障碍或物体时,需要学习类似的注意力。
As mentioned before, this network tends to learn 2 different attention.
如前所述,该网络倾向于学习2种不同的注意力。
In physical attention, hidden states of the LSTM from the GAN module and learned features from the visual context is provided as input. This helps in learning more about the physical constraints like the path is straight or curved, what is the current movement direction, position, and more?
在物理注意方面 ,将GAN模块中LSTM的隐藏状态和可视上下文中的学习功能作为输入提供。 这有助于了解更多关于物理约束的信息,例如路径是直线还是弯曲的,当前的运动方向,位置等等?
In social attention, the LSTM features learned from the feature module together with hidden states of the LSTM from the GAN module are provided as input. This helps in focusing on all agents that are important to predict the further trajectory.
在社会关注中 ,从功能模块中学习到的LSTM功能以及从GAN模块中获得的LSTM隐藏状态都作为输入提供。 这有助于专注于对预测进一步轨迹很重要的所有代理。
GAN模块 (GAN module)
This module takes that highlighted input features to generate a realistic future path for each agent that satisfies all the social and physical norms.
该模块采用突出显示的输入功能,为满足所有社会和身体规范的每个代理生成现实的未来之路。
This GAN module is majorly inspired by the Social GAN with almost no further changes.
此GAN模块主要受Social GAN的启发,几乎没有进一步的变化。
The input to the generator is the selected features from the attention module as well as white noise ‘z’ sampled from a multivariate normal distribution.
生成器的输入是从注意力模块中选择的功能以及从多元正态分布中采样的白噪声“ z” 。
损失 (Losses)
This approach has used 2 losses which are also similar to Social GAN.
该方法使用了2个损失,这也类似于Social GAN。
- Adversarial Loss: This loss helps in learning discrimination between the generated and real samples. 对抗性损失:这种损失有助于了解生成的样本与真实样本之间的区别。
L2 Loss: This loss is similar to “variety loss” used in Social GAN.
L2损失:此损失类似于Social GAN中使用的“ 品种损失 ”。
社交方式 (Social Ways)
In this paper, they are also trying to predict the pedestrian’s trajectories and their interaction. However, they are also aiming to solve one problem from all previous approaches: Mode Collapse.
在本文中 ,他们还试图预测行人的轨迹及其相互作用。 但是,他们还旨在解决以前所有方法中的一个问题: 模式崩溃 。
Mode Collapse is the opposite of multimodality. In this, the generator tries to produce similar samples or the same set of samples leading to similar modes in output.
模式崩溃与多模式相反。 在这种情况下,生成器尝试生成相似的样本或同一组样本,从而导致输出中的相似模式。
To solve the mode collapse problem, this paper uses info-GAN instead of L2 Loss or variety loss.
为了解决模式崩溃问题,本文使用info-GAN代替了L2损失或品种损失。
方法 (Method)
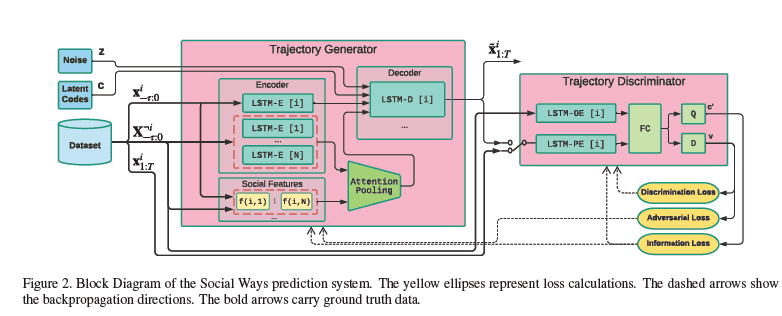
This method comprises of 3 different components:
此方法包含3个不同的组件:
发电机 (Generator)
The generator consists of an encoder-decoder network. Here the past trajectory of each agent was fed into respective LSTM-E (Encoder), which encodes the history of the agent. The output of each LSTM-E was fed into attention pooling as well as a decoder.
生成器由一个编码器-解码器网络组成。 在这里,每个代理的过去轨迹被输入到相应的LSTM-E(编码器)中,该代码对代理的历史进行编码。 每个LSTM-E的输出都被输入到注意力集中以及解码器中。
For decoding, the future trajectories, hidden states from LSTM-E, noise vector ‘z’, latent code ‘c’, and important interacting agent features from the attention pooling are fed into decoder.
对于解码,未来的轨迹,LSTM-E的隐藏状态,噪声矢量“ z”,潜在代码“ c”以及注意力集中的重要交互代理功能都将输入解码器。
The latent code ‘c’ helps in maximizing a lower bound of the mutual information between the distribution of generated output and ‘c’.
潜在代码“ c”有助于最大化生成的输出与“ c”的分布之间的相互信息的下限。
注意池 (Attention Pooling)
This paper uses a similar kind of approach as used in Sophie: an attentive GAN.
本文使用的方法与Sophie中使用的方法类似:殷勤的GAN 。
However, in addition to the euclidean distance between agents (used in Sohpie), 2 more features are being used:
但是,除了代理之间的欧式距离(用于Sohpie)之外,还使用了2个功能:
Bearing angle: “the angle between the velocity vector of agent 1 and vectors joining agents 1 and agent 2.”
方位角 : “代理1的速度矢量与连接代理1和代理2的矢量之间的角度。”
The distance of closest approach: “the smallest distance, 2 agents would reach in the future if both maintain their current velocity.”
最接近的距离 : “最小的距离,如果两个代理都保持当前速度,将来会到达。”
Instead of sorting, the attention weights were obtained by scalar product and softmax operation between hidden states and the above-mentioned three features.
代替排序,注意力权重通过隐藏状态与上述三个特征之间的标量积和softmax操作获得。
鉴别器 (Discriminator)
Discriminator consists of LSTM based encoder with multiple dense layers. Generated trajectories from the generator for each agent and ground-truth trajectories were fed into the discriminator.
鉴别器由具有多个密集层的基于LSTM的编码器组成。 生成器为每个代理生成的轨迹和真实的轨迹被输入到鉴别器中。
As output, the probability that generated trajectories are real is provided.
作为输出,提供了生成的轨迹是真实的概率。
损失 (Losses)
There are 2 losses used in this process:
此过程中使用了2种损失:
Adversarial Loss: This is the normal GAN loss that helps in differentiating between real and fake samples.
对抗性损失 :这是正常的GAN损失,有助于区分真实和伪造样品。
Information Loss: The basic idea of this loss is to maximize mutual information. And that is achieved by minimizing the negative- loss likelihood on salient variable ‘c’.
结果 (Results)
All three papers have tried to learn from previous approaches and have gained some new insights.
所有这三篇论文都试图向以前的方法学习,并获得了一些新见解。
There are 2 metrics that are used to evaluate this application:
有两个用于评估此应用程序的指标:
Average Displacement Error (ADE): It is the average L2 distance on all predicted timesteps between the generated trajectory and ground-truth trajectory.
平均位移误差(ADE) :它是在生成的轨迹和地面真实轨迹之间的所有预测时间步长上的平均L2距离。
Final Displacement Error(FDE): This is the smallest distance between the generated trajectory and ground-truth trajectory at the final predicted timestep.
最终位移误差(FDE) :这是在最终预测时间步中生成的轨迹与地面真实轨迹之间的最小距离。
There are 5 datasets, which are used as benchmarking this application. All these approaches have been tested on all these datasets and they have provided valuable comparable results.
有5个数据集,用作基准测试此应用程序。 所有这些方法都在所有这些数据集上进行了测试,它们提供了有价值的可比结果。
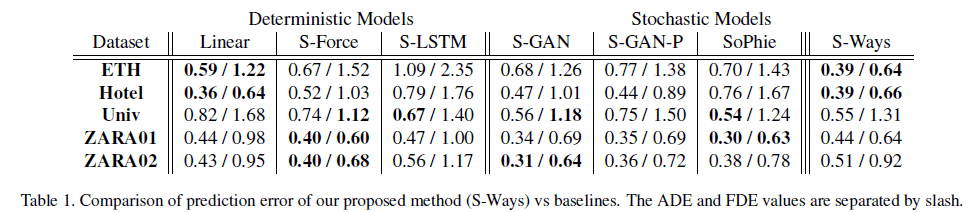
From Fig5. , it can be said that all three approaches show promising results on some datasets. However, I think with hyperparameter tuning and little adjustments, results may shuffle too. I believe all three have certain advantages and could be used to further research in this area.
从图5。 ,可以说这三种方法在某些数据集上都显示出令人鼓舞的结果。 但是,我认为通过超参数调整和少量调整,结果也可能会混乱。 我相信这三者都具有一定的优势,可以用于该领域的进一步研究。
结论 (Conclusion)
The problem of modeling human motion prediction in a scene along with human-human interaction is challenging yet vital for self-driving cars. Without modeling this behavior, it is impossible that self-driving cars could be fully operational.
在场景中对人的运动预测进行建模以及人与人之间的交互作用的问题是具有挑战性的,但对于自动驾驶汽车而言却至关重要。 如果不对此行为进行建模,那么自动驾驶汽车就不可能完全运转。
How to model human-human interaction is the major difference between the above-mentioned approaches. From the theory and suggested methods, I believe attention on distance and the bearing angle between 2 agents is one the most crucial ways to move forward.
如何模拟人与人之间的互动是上述方法之间的主要区别。 从理论和建议的方法来看,我认为关注距离和两个代理之间的方位角是前进的最关键方法之一。
But it is completely my perspective. There could be multiple ways that this could be implemented and enhanced. And we will see that in Part 2 too.
但这完全是我的观点。 可能有多种方法可以实现和增强它。 我们还将在第2部分中看到这一点。
With self-driving cars as the focus, I would continue with more approaches in Part 2 with a focus on the trajectory prediction of cars.
以自动驾驶汽车为重点,我将在第2部分中继续介绍更多方法,重点是汽车的轨迹预测。
I am happy for any further discussion on this paper and in this area. You can leave a comment here or reach out to me on my LinkedIn profile.
对于本文和该领域的任何进一步讨论,我感到很高兴。 您可以在此处发表评论,也可以通过我的LinkedIn个人资料与我联系。
翻译自: https://towardsdatascience.com/trajectory-prediction-self-driving-cars-ai-40a7c6eecb4c