论文题目:《Deep Face Recognition: A Survey》
论文作者:Mei Wang, Weihong Deng
论文链接:http://cn.arxiv.org/pdf/1804.06655.pdf
随着2012年AlexNet赢得了ImageNet挑战赛的冠军后,深度学习技术在各个领域都发挥着重要的作用,极大地提升了许多任务的SOTA。2014年,DeepFace1首次在著名的非受限环境人脸数据集——LFW上取得了与人类相媲美的准确率(DeepFace: 97.35% vs. Human: 97.53%)。因此,本文主要关注深度学习技术在人脸识别领域的应用与发展。
概念和术语
人脸系统一般包括三个部分:
人脸检测(face detection):对于一幅图像,检测其中人脸的位置;
人脸对齐(face alignment):根据人脸关键点,将人脸对齐到一个典型的角度;
人脸识别(face recognition):包括人脸处理、人脸表示和人脸匹配部分。
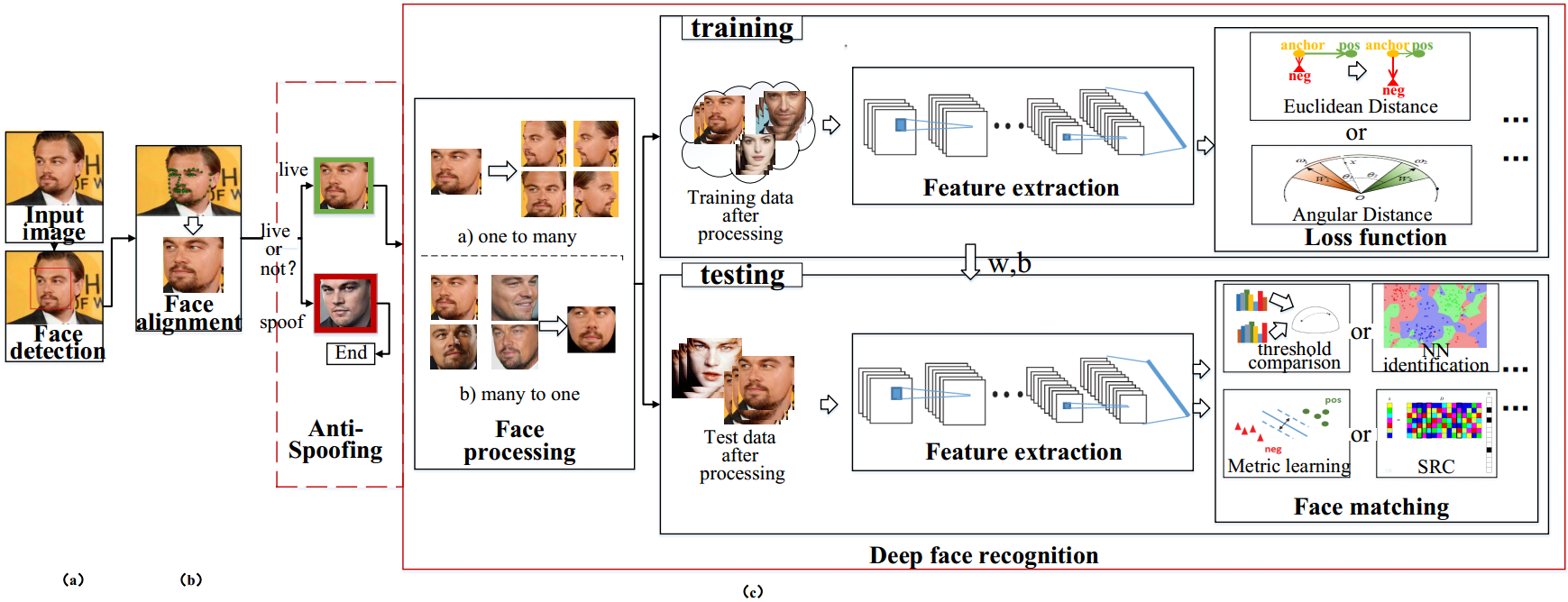
训练集(training set):用于训练系统的人脸集;
注册集(gallery set):提前注册在系统中用于比对的标准人脸集;
测试集(probe set):用于测试的人脸集。
人脸识别任务主要包括:
人脸认证(face identification):为1:N的问题。通过计算测试个体与注册集个体的相似度,判断出当前测试个体的身份。根据测试集中的个体是否出现在注册集中,可分为闭集(closed-set)和开集(open-set)问题。
人脸验证(face verification):为1:1的问题。对测试集和验证集中的个体进行两两比对,判断是否是同一个体。
网络结构
主流结构
在人脸识别问题中,主流的网络结构基本上都借鉴于物体分类问题,一直从AlexNet到SENet。
在2014年,DeepFace2首次使用九层的卷积神经网络,经过3D人脸对齐处理,在LFW上达到了97.35%的准确率。在2015年,FaceNet3在一个很大的私人数据集上训练GoogLeNet,采用triplet loss,得到99.63%的准确率。同年,VGGface4从互联网中收集了一个大的数据集,并在其上训练VGGNet,得到了98.95%的准确率。在2017年,SphereFace5使用64层的ResNet结构,采用angular softmax(A-softmax)loss,得到99.42%的准确率。在2017年末,VGGFace26作为一个新人脸的数据集被引入,同时使用SENet进行训练,在IJB-A和IJB-B上都取得SOTA。
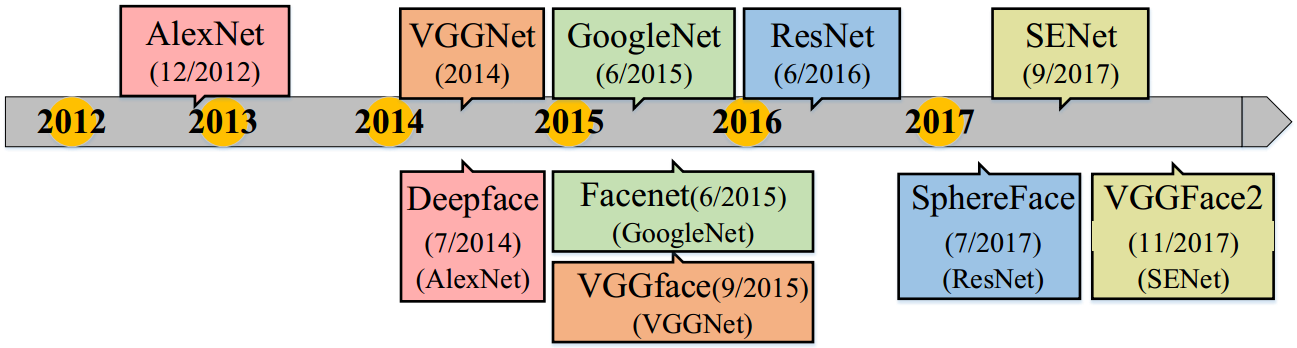
AlexNet
7:AlexNet包括五个卷积层和三个全连接层,并且集成了如ReLU、dropout、数据增强等技术;VGGNet
8:使用3×3卷积核,且每经过2×2的池化后特征图数量加倍,网络深度为16-19层;GoogLeNet
9:提出了inception module,对不同尺度的特征图进行混合;ResNet
10:通过学习残差表示,使得训练更深网络成为可能;SENet
11:提出了Squeeze-and-Excitation操作,通过显式建模channel之间的相互依赖性,自适应地重新校准channel间的特征响应。
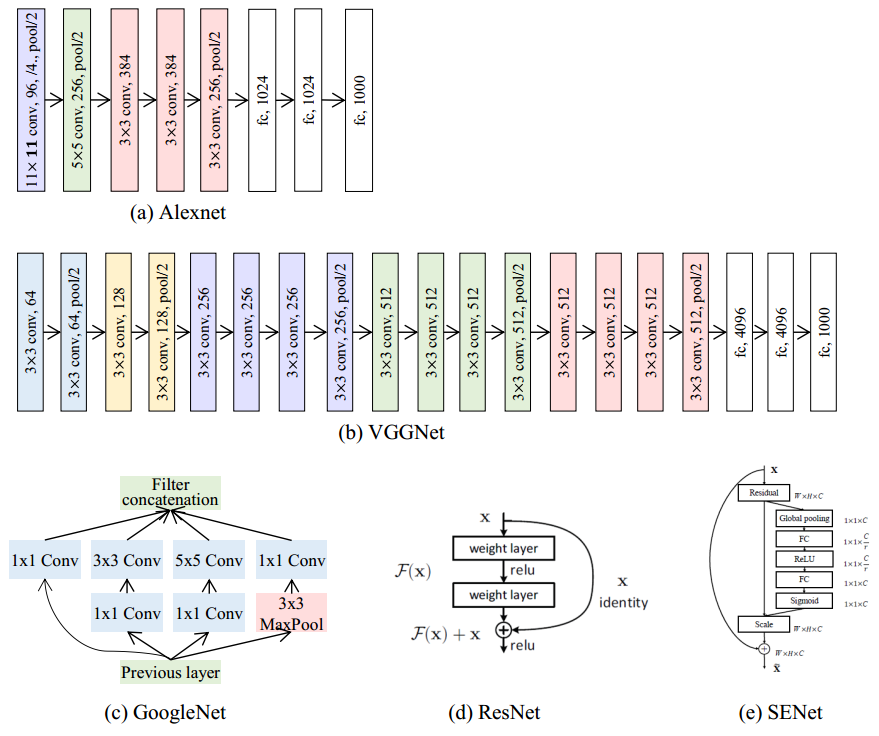
特殊结构
损失函数
在一开始,人们使用和物体分类同样的基于交叉熵的softmax loss,后来发现其不适用于人脸特征的学习,于是开始探索更具有判别性的loss。
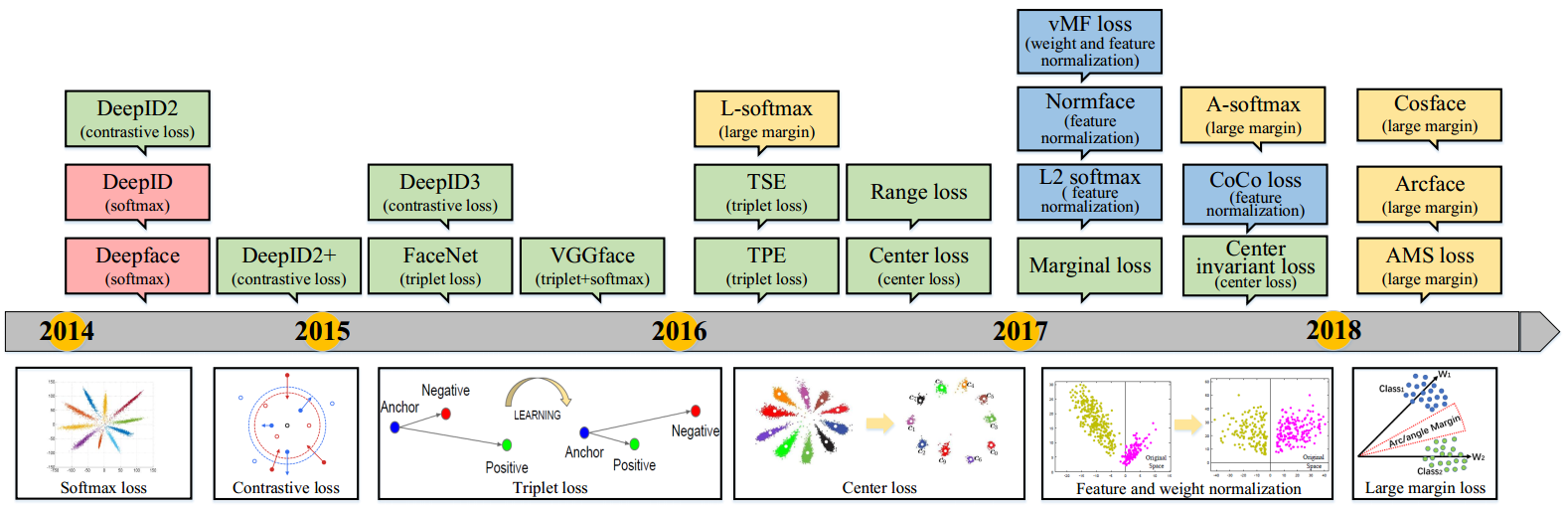
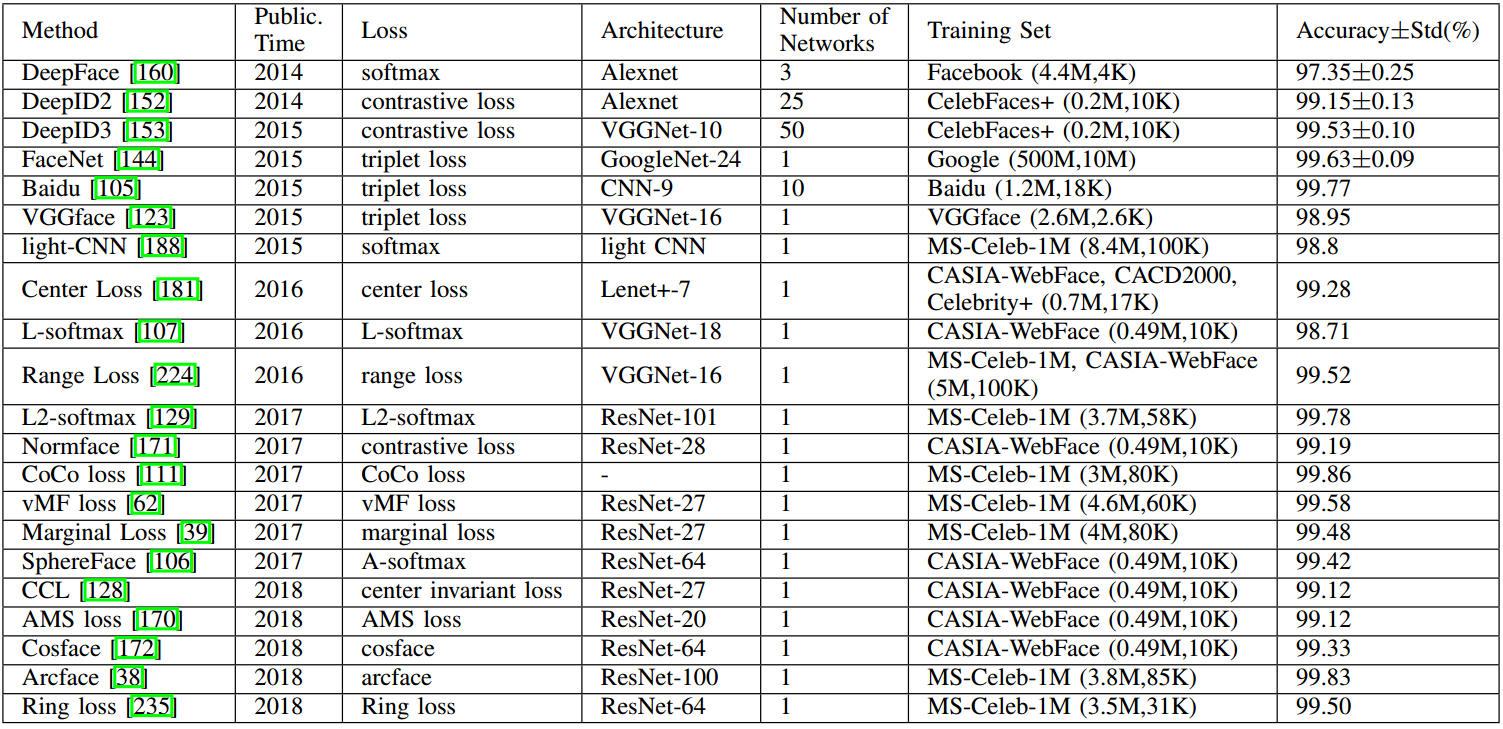
基于欧几里德距离
contrastive loss
相关文献:
- 《Deep learning face representation by joint identification-verification》
- 《Deepid3: Face recognition with very deep neural networks》
DeepID系列使用的loss。
\[ \operatorname { Verif } \left( f _ { i } , f _ { j } , y _ { i j } , \theta _ { v e } \right) = \left\{ \begin{array} { l l } { \frac { 1 } { 2 } \left\| f _ { i } - f _ { j } \right\| _ { 2 } ^ { 2 } } & { \text { if } y _ { i j } = 1 } \\ { \frac { 1 } { 2 } \max \left( 0 , m - \left\| f _ { i } - f _ { j } \right\| _ { 2 } \right) ^ { 2 } } & { \text { if } y _ { i j } = - 1 } \end{array} \right. \]
triplet loss
相关文献:
- 《Facenet: A unified embedding for face recognition and clustering》
\[ \mathcal{L} = \sum _ { i } ^ { N } \left[ \left\| f \left( x _ { i } ^ { a } \right) - f \left( x _ { i } ^ { p } \right) \right\| _ { 2 } ^ { 2 } - \left\| f \left( x _ { i } ^ { a } \right) - f \left( x _ { i } ^ { n } \right) \right\| _ { 2 } ^ { 2 } + \alpha \right] _ { + } \]
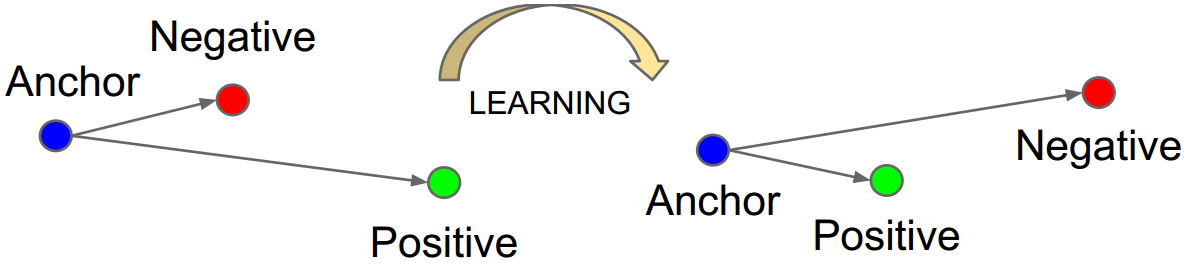
center loss
相关文献:
- 《A Discriminative Feature Learning Approach for Deep Face Recognition》
\[ \begin{aligned} \mathcal { L } & = \mathcal { L } _ { S } + \lambda \mathcal { L } _ { C } \\ & = - \sum _ { i = 1 } ^ { m } \log \frac { e ^ { W _ { y _ { i } } ^ { T } \boldsymbol { x } _ { i } + b _ { y _ { i } } } } { \sum _ { j = 1 } ^ { n } e ^ { W _ { j } ^ { T } \boldsymbol { x } _ { i } + b _ { j } } } + \frac { \lambda } { 2 } \sum _ { i = 1 } ^ { m } \left\| \boldsymbol { x } _ { i } - \boldsymbol { c } _ { y _ { i } } \right\| _ { 2 } ^ { 2 } \end{aligned} \]
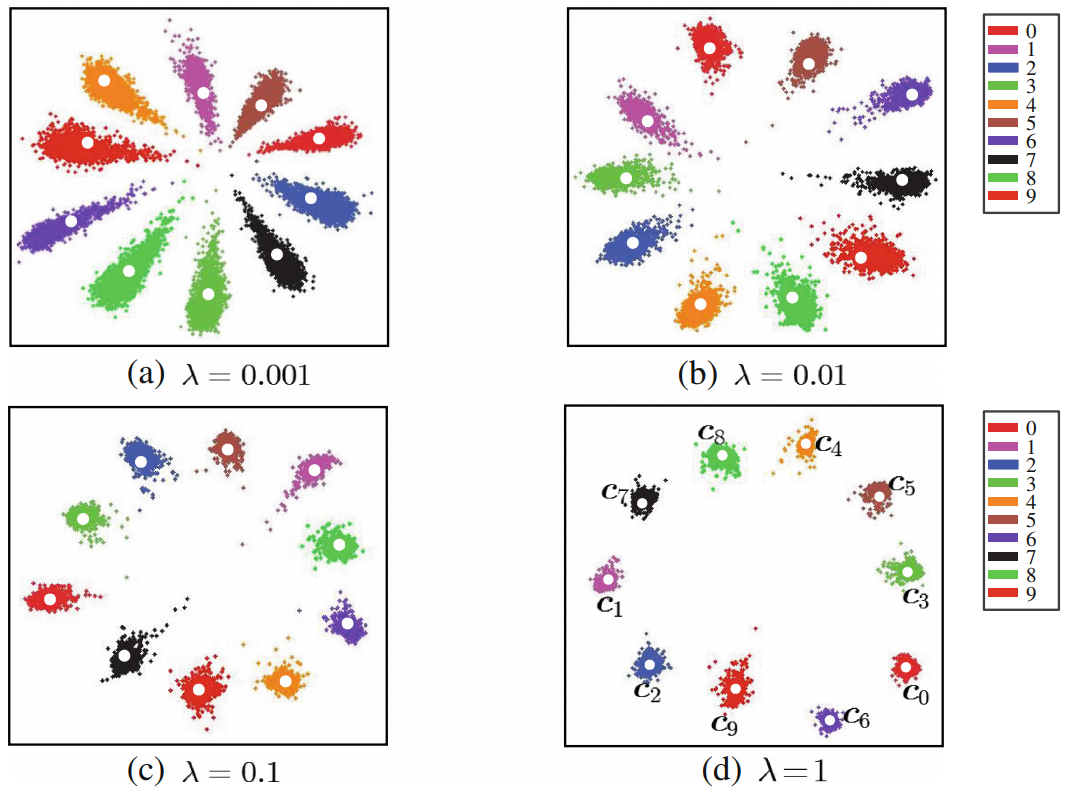
range loss
相关文献:
- 《Range loss for deep face recognition with long-tail》
\[ \mathcal { L } _ { R } = \alpha \mathcal { L } _ { R _ { intra } } + \beta \mathcal { L } _ { R _ { inter } } \]
\[ \mathcal { L } _ { R _ { i n t r a } } = \sum _ { i \subseteq I } \mathcal { L } _ { R _ { i n t r a }}^ { i } = \sum _ { i \subseteq I } \frac { k } { \sum _ { j = 1 } ^ { k } \frac { 1 } { \mathcal { D } _ { j } } } \]
\[ \begin{aligned} \mathcal { L } _ { R _ { \text {inter} } } & = \max \left( m - \mathcal { D } _ { C e n t e r } , 0 \right) \\ & = \max \left( m - \left\| \overline { x } _ { \mathcal { Q } } - \overline { x } _ { \mathcal { R } } \right\| _ { 2 } ^ { 2 } , 0 \right) \end{aligned} \]
\[ \mathcal { L } = \mathcal { L } _ { M } + \lambda \mathcal { L } _ { R } = - \sum _ { i = 1 } ^ { M } \log \frac { e ^ { W _ { y _ { i } } ^ { T } x _ { i } + b _ { v _ { i } } } } { \sum _ { j = 1 } ^ { n } e ^ { W _ { j } ^ { T } x _ { i } + b _ { j } } } + \lambda \mathcal { L } _ { R } \]
center-invariant loss
相关文献:
- 《Deep face recognition with center invariant loss》
\[ \begin{aligned} L = & L _ { s } + \gamma L _ { I } + \lambda L _ { c } \\ = & - \log \left( \frac { e ^ { \mathbf { w } _ { y } ^ { T } \mathbf { x } _ { i } + b _ { y } } } { \sum _ { j = 1 } ^ { m } e ^ { \mathbf { w } _ { j } ^ { T } \mathbf { x } _ { i } + b _ { j } } } \right) + \frac { \gamma } { 4 } \left( \left\| \mathbf { c } _ { y } \right\| _ { 2 } ^ { 2 } - \frac { 1 } { m } \sum _ { k = 1 } ^ { m } \left\| \mathbf { c } _ { k } \right\| _ { 2 } ^ { 2 } \right) ^ { 2 } \\ & + \frac { \lambda } { 2 } \left\| \mathbf { x } _ { i } - \mathbf { c } _ { y } \right\| ^ { 2 } \end{aligned} \]
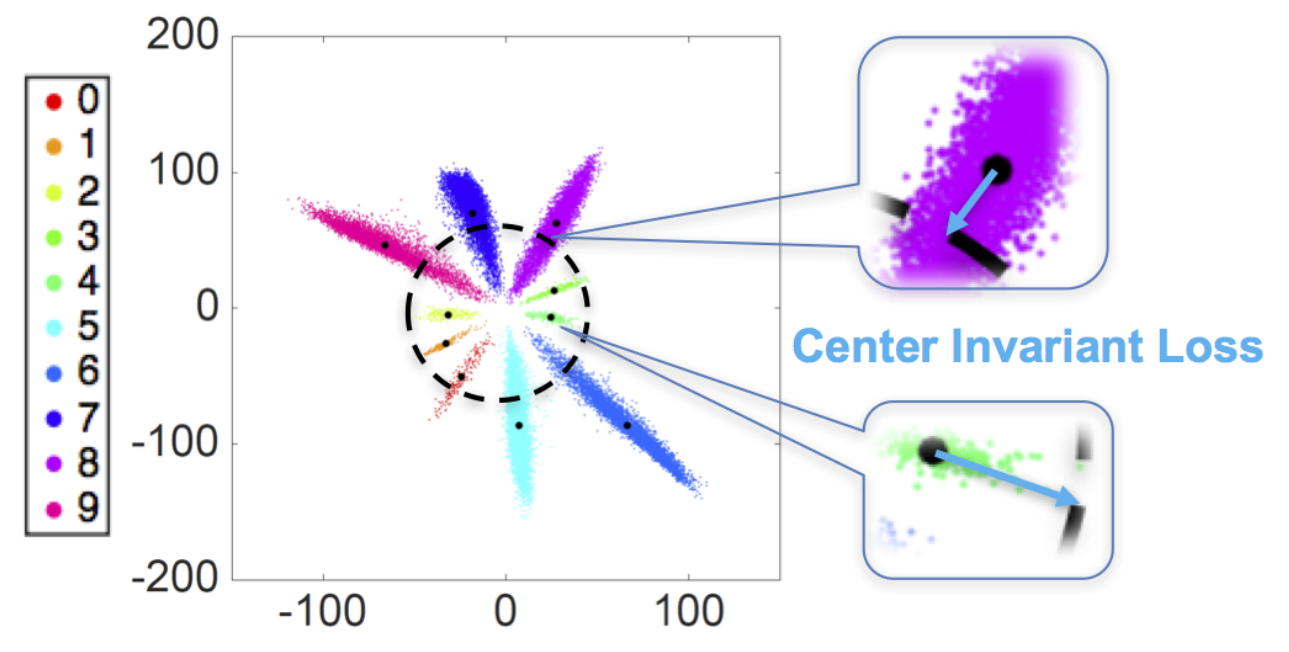
基于角度/余弦间隔
L-Softmax loss
相关文献:
- 《Large-margin softmax loss for convolutional neural networks》
\[ L _ { i } = - \log \left( \frac { e ^ { \left\| \boldsymbol { W } _ { y _ { i } } \right\| \left\| \boldsymbol { x } _ { i } \right\| \psi \left( \theta _ { y _ { i } } \right) } } { e ^ { \left\| \boldsymbol { W } _ { y _ { i } } \right\| \boldsymbol { w } \left( \theta _ { \boldsymbol { y } _ { i } } \right) } + \sum _ { j \neq y _ { i } } e ^ { \left\| \boldsymbol { W } _ { j } \right\| \left\| \boldsymbol { x } _ { i } \right\| \cos \left( \theta _ { j } \right) } } \right) \]
\[ \psi ( \theta ) = ( - 1 ) ^ { k } \cos ( m \theta ) - 2 k , \quad \theta \in \left[ \frac { k \pi } { m } , \frac { ( k + 1 ) \pi } { m } \right] \]
\[ f _ { y _ { i } } = \frac { \lambda \left\| \boldsymbol { W } _ { y _ { i } } \right\| \left\| \boldsymbol { x } _ { i } \right\| \cos \left( \theta _ { y _ { i } } \right) + \left\| \boldsymbol { W } _ { y _ { i } } \right\| \left\| \boldsymbol { x } _ { i } \right\| \psi \left( \theta _ { \boldsymbol { y } _ { i } } \right) } { 1 + \lambda } \]
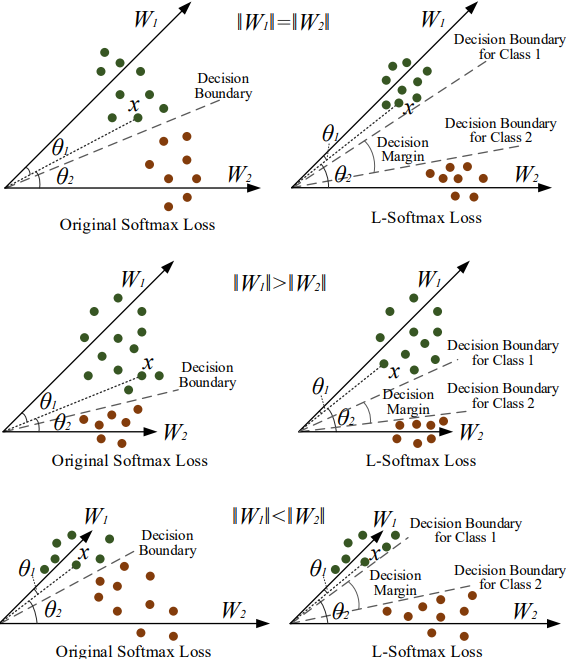
A-Softmax loss
相关文献:
- 《Sphereface: Deep hypersphere embedding for face recognition》
\[ L _ { \mathrm { ang } } = \frac { 1 } { N } \sum _ { i } - \log \left( \frac { e ^ { \left\| \boldsymbol { x } _ { i } \right\| \psi \left( \theta _ { y _ { i } , i } \right) } } { e ^ { \left\| \boldsymbol { x } _ { i } \right\| \psi \left( \theta _ { y _ { i } } , i \right) } + \sum _ { j \neq y _ { i } } e ^ { \left\| \boldsymbol { x } _ { i } \right\| \cos \left( \theta _ { j , i } \right) } } \right) \]
\[ \psi \left( \theta _ { y _ { i } , i } \right) = ( - 1 ) ^ { k } \cos \left( m \theta _ { y _ { i } , i } \right) - 2 k \]
\[ \theta _ { y _ { i } , i } \in \left[ \frac { k \pi } { m } , \frac { ( k + 1 ) \pi } { m } \right] \text { and } k \in [ 0 , m - 1 ] \]
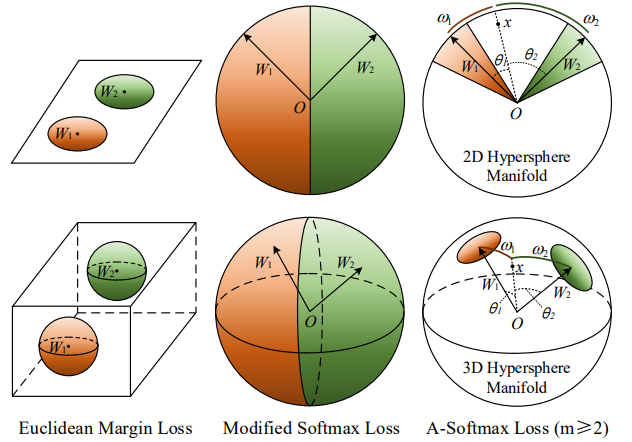
AM-Softmax loss
相关文献:
- 《Additive margin softmax for face verification》
\[ \begin{aligned} \mathcal { L } _ { A M S } & = - \frac { 1 } { n } \sum _ { i = 1 } ^ { n } \log \frac { e ^ { s \cdot \left( \cos \theta _ { y _ { i } } - m \right) } } { e ^ { s \cdot \left( \cos \theta _ { y _ { i } } - m \right) } + \sum _ { j = 1 , j \neq y _ { i } } ^ { c } e ^ { s \cdot c o s \theta _ { j } } } \\ & = - \frac { 1 } { n } \sum _ { i = 1 } ^ { n } \log \frac { e ^ { s \cdot \left( W _ { y _ { i } } ^ { T } f _ { i } - m \right) } } { e ^ { s \cdot \left( W _ { y _ { i } } ^ { T } \boldsymbol { f } _ { i } - m \right) } + \sum _ { j = 1 , j \neq y _ { i } } ^ { c } e ^ { S W _ { j } ^ { T } \boldsymbol { f } _ { i } } } \end{aligned} \]
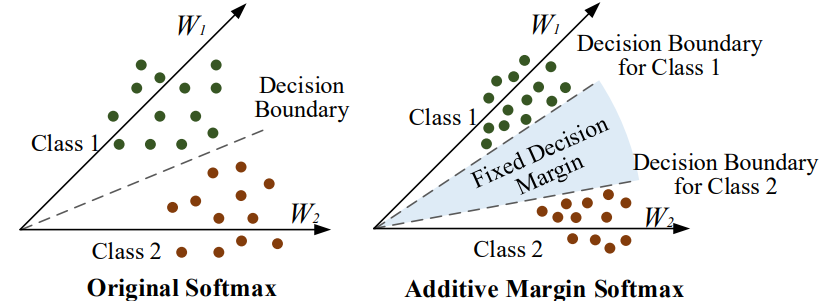
CosFace
相关文献:
- 《Cosface: Large margin cosine loss for deep face recognition》
\[ L _ { l m c } = \frac { 1 } { N } \sum _ { i } - \log \frac { e ^ { s \left( \cos \left( \theta _ { y _ { i } , i } \right) - m \right) } } { e ^ { s \left( \cos \left( \theta _ { y _ { i } } , i \right) - m \right) } + \sum _ { j \neq y _ { i } } e ^ { s \cos \left( \theta _ { j , i } \right) } } \]
\[ \begin{aligned} \text { subject to } \\ W & = \frac { W ^ { * } } { \left\| W ^ { * } \right\| } \\ x & = \frac { x ^ { * } } { \left\| x ^ { * } \right\| } \\ \cos \left( \theta _ { j } , i \right) & = W _ { j } ^ { T } x _ { i } \end{aligned} \]
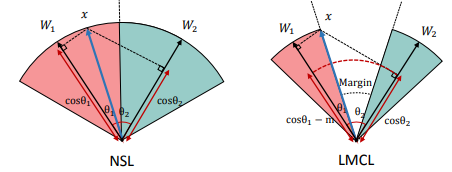
ArcFace
相关文献:
- 《Arcface: Additive angular margin loss for deep face recognition》
\[ L = - \frac { 1 } { N } \sum _ { i = 1 } ^ { N } \log \frac { e ^ { s \left( \cos \left( \theta _ { y _ { i } } + m \right) \right) } } { e ^ { s \left( \cos \left( \theta _ { y _ { i } } + m \right) \right) } + \sum _ { j = 1 , j \neq y _ { i } } ^ { n } e ^ { s \cos \theta _ { j } } } \]
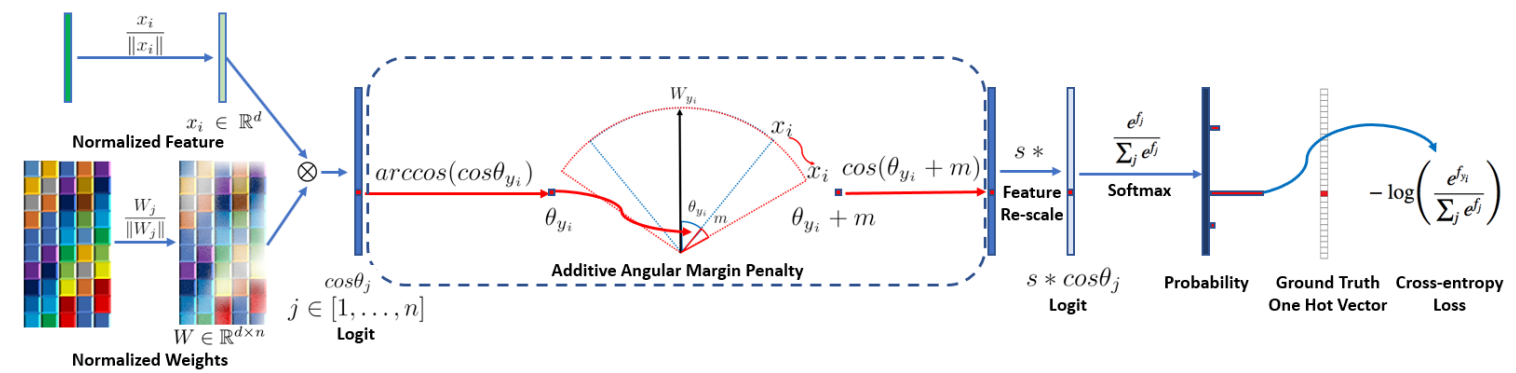
Softmax及其变种
L2-Softmax
相关文献:
- 《L2-constrained softmax loss for discriminative face verification》
\[ \begin{array} { l l } { \text { minimize } } & { - \frac { 1 } { M } \sum _ { i = 1 } ^ { M } \log \frac { e ^ { W _ { y _ { i } } ^ { T } f \left( \mathbf { x } _ { i } \right) + b _ { y _ { i } } } } { \sum _ { j = 1 } ^ { C } e ^ { W _ { j } ^ { T } f \left( \mathbf { x } _ { i } \right) + b _ { j } } } } \\ { \text { subject to } } & { \left\| f \left( \mathbf { x } _ { i } \right) \right\| _ { 2 } = \alpha , \forall i = 1,2 , \ldots M } \end{array} \]
Normface
相关文献:
- 《NormFace: L2 Hypersphere Embedding for Face Verification》
\[ \mathcal { L } _ { S' } = - \frac { 1 } { m } \sum _ { i = 1 } ^ { m } \log \frac { e ^ { s \tilde { W } _ { y _ { i } } ^ { T } \tilde { \mathbf { f } } _ { i } } } { \sum _ { j = 1 } ^ { n } e ^ { s \tilde { W } _ { j } ^ { T } \mathbf { f } _ { i } } } \]
\[ \tilde { \mathbf { x } } = \frac { \mathbf { x } } { \| \mathbf { x } \| _ { 2 } } = \frac { \mathbf { x } } { \sqrt { \sum _ { i } \mathbf { x } _ { i } ^ { 2 } + \epsilon } } \]
CoCo loss
相关文献:
- 《Rethinking feature discrimination and polymerization for large-scale recognition》
\[ \mathcal { L } ^ { C O C O } \left( \boldsymbol { f } ^ { ( i ) } , \boldsymbol { c } _ { k } \right) = - \sum _ { i \in \mathcal { B } , k } t _ { k } ^ { ( i ) } \log p _ { k } ^ { ( i ) } = - \sum _ { i \in \mathcal { B } } \log p _ { l _ { i } } ^ { ( i ) } \]
\[ \hat { \boldsymbol { c } } _ { k } = \frac { \boldsymbol { c } _ { k } } { \left\| \boldsymbol { c } _ { k } \right\| } , \hat { \boldsymbol { f } } ^ { ( i ) } = \frac { \alpha \boldsymbol { f } ^ { ( i ) } } { \left\| \boldsymbol { f } ^ { ( i ) } \right\| } , p _ { k } ^ { ( i ) } = \frac { \exp \left( \hat { \boldsymbol { c } } _ { k } ^ { T } \cdot \hat { \boldsymbol { f } } ^ { ( i ) } \right) } { \sum _ { m } \exp \left( \hat { \boldsymbol { c } } _ { m } ^ { T } \cdot \hat { \boldsymbol { f } } ^ { ( i ) } \right) } \]
Ring loss
相关文献:
- 《Ring loss: Convex feature normalization for face recognition》
\[ L _ { R } = \frac { \lambda } { 2 m } \sum _ { i = 1 } ^ { m } \left( \left\| \mathcal { F } \left( \mathbf { x } _ { i } \right) \right\| _ { 2 } - R \right) ^ { 2 } \]
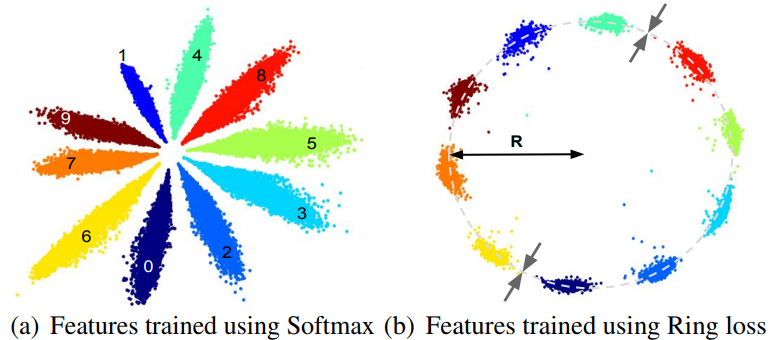
参考文献
Y. Taigman, M. Yang, M. Ranzato, and L. Wolf. Deepface: Closing the gap to human-level performance in face verification. In CVPR, pages 1701–1708, 2014.↩
Y. Taigman, M. Yang, M. Ranzato, and L. Wolf. Deepface: Closing the gap to human-level performance in face verification. In CVPR, pages 1701–1708, 2014.↩
F. Schroff, D. Kalenichenko, and J. Philbin. Facenet: A unified embedding for face recognition and clustering. In CVPR, pages 815–823, 2015.↩
O. M. Parkhi, A. Vedaldi, A. Zisserman, et al. Deep face recognition. In BMVC, volume 1, page 6, 2015.↩
W. Liu, Y. Wen, Z. Yu, M. Li, B. Raj, and L. Song. Sphereface: Deep hypersphere embedding for face recognition. In CVPR, volume 1, 2017.↩
Q. Cao, L. Shen, W. Xie, O. M. Parkhi, and A. Zisserman. Vggface2: A dataset for recognising faces across pose and age. arXiv preprint arXiv:1710.08092, 2017.↩
A. Krizhevsky, I. Sutskever, and G. E. Hinton. Imagenet classification with deep convolutional neural networks. In NIPS, pages 1097–1105, 2012.↩
K. Simonyan and A. Zisserman. Very deep convolutional networks for large-scale image recognition. arXiv preprint arXiv:1409.1556, 2014.↩
C. Szegedy, W. Liu, Y. Jia, P. Sermanet, S. Reed, D. Anguelov, D. Erhan, V. Vanhoucke, A. Rabinovich, et al. Going deeper with convolutions. In CVPR, 2015.↩
K. He, X. Zhang, S. Ren, and J. Sun. Deep residual learning for image recognition. In CVPR, pages 770–778, 2016.↩
J. Hu, L. Shen, and G. Sun. Squeeze-and-excitation networks. arXiv preprint arXiv:1709.01507, 2017.↩
X. Wu, R. He, Z. Sun, and T. Tan. A light cnn for deep face representation with noisy labels. arXiv preprint arXiv:1511.02683, 2015.↩
A. R. Chowdhury, T.-Y. Lin, S. Maji, and E. Learned-Miller. One-to-many face recognition with bilinear cnns. In WACV, pages 1–9. IEEE, 2016.↩