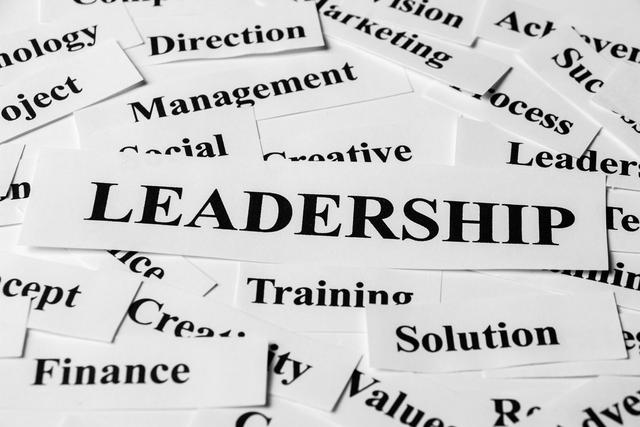
刚刚接触pandas的朋友,想了解数据结构,就一定要认识DataFrame,接下来给大家详细介绍!
初识DataFrame
import numpy as npimport pandas as pd
data = {"name": ["Jack", "Tom", "LiSa"], "age": [20, 21, 18], "city": ["BeiJing", "TianJin", "ShenZhen"]}print(data)print("")frame = pd.DataFrame(data) # 创建DataFrameprint(frame)print("")print(frame.index) # 查看行索引print("")print(frame.columns) # 查看列索引print("")print(frame.values) # 查看值
{'name': ['Jack', 'Tom', 'LiSa'], 'age': [20, 21, 18], 'city': ['BeiJing', 'TianJin', 'ShenZhen']} age city name0 20 BeiJing Jack1 21 TianJin Tom2 18 ShenZhen LiSaRangeIndex(start=0, stop=3, step=1)Index(['age', 'city', 'name'], dtype='object')[[20 'BeiJing' 'Jack'] [21 'TianJin' 'Tom'] [18 'ShenZhen' 'LiSa']]
创建DataFrame
方法一: 由字典创建 字典的key是列索引值可以是
1.列表
2.ndarray
3.Series
# 值是ndarray 注意: 用ndarray创建DataFrame值的个数必须相同 否则报错data2 = {"one": np.random.rand(3), "two": np.random.rand(3) }print(data2)print("")print(pd.DataFrame(data2))
{'one': array([ 0.60720023, 0.30838024, 0.30678266]), 'two': array([ 0.21368784, 0.03797809, 0.41698718])} one two0 0.607200 0.2136881 0.308380 0.0379782 0.306783 0.416987
# 值是Series--带有标签的一维数组 注意: 用Series创建DataFrame值的个数可以不同 少的值用Nan填充data3 = {"one": pd.Series(np.random.rand(4)), "two": pd.Series(np.random.rand(5)) }print(data3)print("")df3 = pd.DataFrame(data3)print(df3)print("")
{'one': 0 0.2176391 0.9216412 0.8988103 0.933510dtype: float64, 'two': 0 0.1327891 0.0999042 0.7234953 0.7191734 0.477456dtype: float64} one two0 0.217639 0.1327891 0.921641 0.0999042 0.898810 0.7234953 0.933510 0.7191734 NaN 0.477456
# 值是Series--带有标签的一维数组 注意: 用Series创建DataFrame值的个数可以不同 少的值用Nan填充data3 = {"one": pd.Series(np.random.rand(4)), "two": pd.Series(np.random.rand(5)) }print(data3)print("")df3 = pd.DataFrame(data3)print(df3)print("")
{'one': 0 0.2176391 0.9216412 0.8988103 0.933510dtype: float64, 'two': 0 0.1327891 0.0999042 0.7234953 0.7191734 0.477456dtype: float64} one two0 0.217639 0.1327891 0.921641 0.0999042 0.898810 0.7234953 0.933510 0.7191734 NaN 0.477456
方法二: 通过二维数组直接创建
data = [{"one": 1, "two": 2}, {"one": 5, "two": 10, "three": 15}] # 每一个字典在DataFrame里就是一行数据print(data)print("")df1 = pd.DataFrame(data)print(df1)print("")df2 = pd.DataFrame(data, index=list("ab"), columns=["one", "two", "three", "four"])print(df2)
[{'one': 1, 'two': 2}, {'one': 5, 'two': 10, 'three': 15}] one three two0 1 NaN 21 5 15.0 10 one two three foura 1 2 NaN NaNb 5 10 15.0 NaN
方法三: 由字典组成的列表创建 DataFrame
# columns为字典的key index为子字典的keydata = {"Jack": {"age":1, "country":"China", "sex":"man"}, "LiSa": {"age":18, "country":"America", "sex":"women"}, "Tom": {"age":20, "country":"English"}}df1 = pd.DataFrame(data)print(df1)print("")# 注意: 这里的index并不能给子字典的key(行索引)重新命名 但可以给子字典的key重新排序 若出现原数组没有的index 那么就填充NaN值df2 = pd.DataFrame(data, index=["sex", "age", "country"])print(df2)print("")df3 = pd.DataFrame(data, index=list("abc"))print(df3)print("")# columns 给列索引重新排序 若出现原数组没有的列索引填充NaN值df4 = pd.DataFrame(data, columns=["Tom", "LiSa", "Jack", "TangMu"])print(df4)
Jack LiSa Tomage 1 18 20country China America Englishsex man women NaN Jack LiSa Tomsex man women NaNage 1 18 20country China America English Jack LiSa Toma NaN NaN NaNb NaN NaN NaNc NaN NaN NaN Tom LiSa Jack TangMuage 20 18 1 NaNcountry English America China NaNsex NaN women man NaN
方法四: 由字典组成的字典
# columns为字典的key index为子字典的keydata = {"Jack": {"age":1, "country":"China", "sex":"man"}, "LiSa": {"age":18, "country":"America", "sex":"women"}, "Tom": {"age":20, "country":"English"}}df1 = pd.DataFrame(data)print(df1)print("")# 注意: 这里的index并不能给子字典的key(行索引)重新命名 但可以给子字典的key重新排序 若出现原数组没有的index 那么就填充NaN值df2 = pd.DataFrame(data, index=["sex", "age", "country"])print(df2)print("")df3 = pd.DataFrame(data, index=list("abc"))print(df3)print("")# columns 给列索引重新排序 若出现原数组没有的列索引填充NaN值df4 = pd.DataFrame(data, columns=["Tom", "LiSa", "Jack", "TangMu"])print(df4)
Jack LiSa Tomage 1 18 20country China America Englishsex man women NaN Jack LiSa Tomsex man women NaNage 1 18 20country China America English Jack LiSa Toma NaN NaN NaNb NaN NaN NaNc NaN NaN NaN Tom LiSa Jack TangMuage 20 18 1 NaNcountry English America China NaNsex NaN women man NaN
DataFrame索引
选择行与列
选择列 直接用df["列标签"]
df = pd.DataFrame(np.random.rand(12).reshape(3,4)*100, index = ["one", "two", "three"], columns = ["a", "b", "c", "d"])print(df)print("")print(df["a"], " ", type(df["a"])) # 取一列print("")print(df[["a", "c"]], " ", type(df[["a", "c"]])) # 取多列
a b c done 92.905464 11.630358 19.518051 77.417377two 91.107357 0.641600 4.913662 65.593182three 3.152801 42.324671 14.030304 22.138608one 92.905464two 91.107357three 3.152801Name: a, dtype: float64 a cone 92.905464 19.518051two 91.107357 4.913662three 3.152801 14.030304
选择行 不能通过标签索引 df["one"] 来选择行 要用 df.loc["one"], loc就是针对行来操作的
print(df)print("")print(df.loc["one"], " ", type(df.loc["one"])) # 取一行print("")print(df.loc[["one", "three"]], " ", type(df.loc[["one", "three"]])) # 取不连续的多行print("")
a b c done 92.905464 11.630358 19.518051 77.417377two 91.107357 0.641600 4.913662 65.593182three 3.152801 42.324671 14.030304 22.138608a 92.905464b 11.630358c 19.518051d 77.417377Name: one, dtype: float64 a b c done 92.905464 11.630358 19.518051 77.417377three 3.152801 42.324671 14.030304 22.138608
loc支持切片索引--针对行 并包含末端 df.loc["one": "three"]
df = pd.DataFrame(np.random.rand(16).reshape(4,4)*100, index=["one", "two", "three", "four"], columns=["a", "b", "c", "d"])print(df)print("")print(df.loc["one": "three"])print("") print(df[: 3]) # 切片表示取连续的多行(尽量不用 免得混淆)
a b c done 65.471894 19.137274 31.680635 41.659808two 31.570587 45.575849 37.739644 5.140845three 54.930986 68.232707 17.215544 70.765401four 45.591798 63.274956 74.056045 2.466652 a b c done 65.471894 19.137274 31.680635 41.659808two 31.570587 45.575849 37.739644 5.140845three 54.930986 68.232707 17.215544 70.765401 a b c done 65.471894 19.137274 31.680635 41.659808two 31.570587 45.575849 37.739644 5.140845three 54.930986 68.232707 17.215544 70.765401
iloc也是对行来操作的 只不过把行标签改成了行索引 并且是不包含末端的
print(df)print("")print(df.iloc[0]) # 取一行print("")print(df.iloc[[0,2]]) # 取不连续的多行print("")print(df.iloc[0:3]) # 不包含末端
a b c done 65.471894 19.137274 31.680635 41.659808two 31.570587 45.575849 37.739644 5.140845three 54.930986 68.232707 17.215544 70.765401four 45.591798 63.274956 74.056045 2.466652a 65.471894b 19.137274c 31.680635d 41.659808Name: one, dtype: float64 a b c done 65.471894 19.137274 31.680635 41.659808three 54.930986 68.232707 17.215544 70.765401 a b c done 65.471894 19.137274 31.680635 41.659808two 31.570587 45.575849 37.739644 5.140845three 54.930986 68.232707 17.215544 70.765401
布尔型索引
df = pd.DataFrame(np.random.rand(16).reshape(4,4)*100, index=["one", "two", "three", "four"], columns=["a", "b", "c", "d"])print(df)print("")d1 = df >50 # d1为布尔型索引print(d1)print("")print(df[d1]) # df根据d1 只返回True的值 False的值对应为NaNprint("")
a b c done 91.503673 74.080822 85.274682 80.788609two 49.670055 42.221393 36.674490 69.272958three 78.349843 68.090150 22.326223 93.984369four 79.057146 77.687246 32.304265 0.567816 a b c done True True True Truetwo False False False Truethree True True False Truefour True True False False a b c done 91.503673 74.080822 85.274682 80.788609two NaN NaN NaN 69.272958three 78.349843 68.090150 NaN 93.984369four 79.057146 77.687246 NaN NaN
选取某一列作为布尔型索引 返回True所在行的所有列 注意: 不能选取多列作为布尔型索引
df = pd.DataFrame(np.random.rand(16).reshape(4,4)*100, index=["one", "two", "three", "four"], columns=["a", "b", "c", "d"], dtype=np.int64)print(df)print("") d2 = df["b"] > 50 print(d2)print("")print(df[d2])
a b c done 27 18 47 61two 26 35 16 78three 80 98 94 41four 85 3 47 90one Falsetwo Falsethree Truefour FalseName: b, dtype: bool a b c dthree 80 98 94 41
选取多列作为布尔型索引 返回True所对应的值 False对应为NaN 没有的列全部填充为NaN
df = pd.DataFrame(np.random.rand(16).reshape(4,4)*100, index=["one", "two", "three", "four"], columns=["a", "b", "c", "d"], dtype=np.int64)print(df)print("")d3 = df[["a", "c"]] > 50print(d3)print("")print(df[d3])
a b c done 49 82 32 39two 78 2 24 84three 6 84 84 69four 21 89 16 77 a cone False Falsetwo True Falsethree False Truefour False False a b c done NaN NaN NaN NaNtwo 78.0 NaN NaN NaNthree NaN NaN 84.0 NaNfour NaN NaN NaN NaN
多重索引
print(df)
a b c done 49 82 32 39two 78 2 24 84three 6 84 84 69four 21 89 16 77
print(df["a"].loc[["one", "three"]]) # 取列再取行print("")print(df[["a", "c"]].iloc[0:3])
one 49three 6Name: a, dtype: int64 a cone 49 32two 78 24three 6 84
print(df.loc[["one", "three"]][["a", "c"]]) # 取行再取列
a cone 49 32three 6 84
print(df > 50)print("")print(df[df>50])print("")print(df[df>50][["a","b"]])
a b c done False True False Falsetwo True False False Truethree False True True Truefour False True False True a b c done NaN 82.0 NaN NaNtwo 78.0 NaN NaN 84.0three NaN 84.0 84.0 69.0four NaN 89.0 NaN 77.0 a bone NaN 82.0two 78.0 NaNthree NaN 84.0four NaN 89.0
DataFrame基本技巧
import numpy as npimport pandas as pd
arr = np.random.rand(16).reshape(8, 2)*10# print(arr)print("")print(len(arr))print("")df = pd.DataFrame(arr, index=[chr(i) for i in range(97, 97+len(arr))], columns=["one", "two"])print(df)
8 one twoa 2.129959 1.827002b 8.631212 0.423903c 6.262012 3.851107d 6.890305 9.543065e 6.883742 3.643955f 2.740878 6.851490g 6.242513 7.402237h 9.226572 3.179664
查看数据
print(df)print("")print(df.head(2)) # 查看头部数据 默认查看5条print("")print(df.tail(3)) # 查看末尾数据 默认查看5条
one twoa 2.129959 1.827002b 8.631212 0.423903c 6.262012 3.851107d 6.890305 9.543065e 6.883742 3.643955f 2.740878 6.851490g 6.242513 7.402237h 9.226572 3.179664 one twoa 2.129959 1.827002b 8.631212 0.423903 one twof 2.740878 6.851490g 6.242513 7.402237h 9.226572 3.179664
转置
print(df)
one twoa 2.129959 1.827002b 8.631212 0.423903c 6.262012 3.851107d 6.890305 9.543065e 6.883742 3.643955f 2.740878 6.851490g 6.242513 7.402237h 9.226572 3.179664
print(df.T)
a b c d e f g one 2.129959 8.631212 6.262012 6.890305 6.883742 2.740878 6.242513 two 1.827002 0.423903 3.851107 9.543065 3.643955 6.851490 7.402237 h one 9.226572 two 3.179664
添加与修改
df = pd.DataFrame(np.random.rand(16).reshape(4,4),index=["one", "two", "three", "four"], columns=["a", "b", "c", "d"])print(df)print("")df.loc["five"] = 100 # 增加一行print(df)print("")df["e"] = 10 # 增加一列print(df)print("")df["e"] = 101 # 修改一列print(df)print("")df.loc["five"] = 111 # 修改一行print(df)print("")
a b c done 0.708481 0.285426 0.355058 0.990070two 0.199559 0.733047 0.322982 0.791169three 0.198043 0.801163 0.356082 0.857501four 0.430182 0.020549 0.896011 0.503088 a b c done 0.708481 0.285426 0.355058 0.990070two 0.199559 0.733047 0.322982 0.791169three 0.198043 0.801163 0.356082 0.857501four 0.430182 0.020549 0.896011 0.503088five 100.000000 100.000000 100.000000 100.000000 a b c d eone 0.708481 0.285426 0.355058 0.990070 10two 0.199559 0.733047 0.322982 0.791169 10three 0.198043 0.801163 0.356082 0.857501 10four 0.430182 0.020549 0.896011 0.503088 10five 100.000000 100.000000 100.000000 100.000000 10 a b c d eone 0.708481 0.285426 0.355058 0.990070 101two 0.199559 0.733047 0.322982 0.791169 101three 0.198043 0.801163 0.356082 0.857501 101four 0.430182 0.020549 0.896011 0.503088 101five 100.000000 100.000000 100.000000 100.000000 101 a b c d eone 0.708481 0.285426 0.355058 0.990070 101two 0.199559 0.733047 0.322982 0.791169 101three 0.198043 0.801163 0.356082 0.857501 101four 0.430182 0.020549 0.896011 0.503088 101five 111.000000 111.000000 111.000000 111.000000 111
删除 del(删除行)/drop(删除列 指定axis=1删除行)
df = pd.DataFrame(np.random.rand(16).reshape(4,4),index=["one", "two", "three", "four"], columns=["a", "b", "c", "d"])print(df)print("")del df["a"] # 删除列 改变原数组print(df)
a b c done 0.339979 0.577661 0.108308 0.482164two 0.374043 0.102067 0.660970 0.786986three 0.384832 0.076563 0.529472 0.358780four 0.938592 0.852895 0.466709 0.938307 b c done 0.577661 0.108308 0.482164two 0.102067 0.660970 0.786986three 0.076563 0.529472 0.358780four 0.852895 0.466709 0.938307
df = pd.DataFrame(np.random.rand(16).reshape(4,4),index=["one", "two", "three", "four"], columns=["a", "b", "c", "d"])print(df)print("")d1 = df.drop("one") # 删除行 并返回新的数组 不改变原数组print(d1)print("")print(df)
a b c done 0.205438 0.324132 0.401131 0.368300two 0.471426 0.671785 0.837956 0.097416three 0.888816 0.451950 0.137032 0.568844four 0.524813 0.448306 0.875787 0.479477 a b c dtwo 0.471426 0.671785 0.837956 0.097416three 0.888816 0.451950 0.137032 0.568844four 0.524813 0.448306 0.875787 0.479477 a b c done 0.205438 0.324132 0.401131 0.368300two 0.471426 0.671785 0.837956 0.097416three 0.888816 0.451950 0.137032 0.568844four 0.524813 0.448306 0.875787 0.479477
df = pd.DataFrame(np.random.rand(16).reshape(4,4),index=["one", "two", "three", "four"], columns=["a", "b", "c", "d"])print(df)print("")d2 = df.drop("a", axis=1) # 删除列 返回新的数组 不会改变原数组print(d2)print("")print(df)
a b c done 0.939552 0.613218 0.357056 0.534264two 0.110583 0.602123 0.990186 0.149132three 0.756016 0.897848 0.176100 0.204789four 0.655573 0.819009 0.094322 0.656406 b c done 0.613218 0.357056 0.534264two 0.602123 0.990186 0.149132three 0.897848 0.176100 0.204789four 0.819009 0.094322 0.656406 a b c done 0.939552 0.613218 0.357056 0.534264two 0.110583 0.602123 0.990186 0.149132three 0.756016 0.897848 0.176100 0.204789four 0.655573 0.819009 0.094322 0.656406
排序
根据指定列的列值排序 同时列值所在的行也会跟着移动 .sort_values(['列'])
# 单列df = pd.DataFrame(np.random.rand(16).reshape(4,4), columns=["a", "b", "c", "d"])print(df)print("")print(df.sort_values(['a'])) # 默认升序 print("")print(df.sort_values(['a'], ascending=False)) # 降序
a b c d0 0.616386 0.416094 0.072445 0.1401671 0.263227 0.079205 0.520708 0.8663162 0.665673 0.836688 0.733966 0.3102293 0.405777 0.090530 0.991211 0.712312 a b c d1 0.263227 0.079205 0.520708 0.8663163 0.405777 0.090530 0.991211 0.7123120 0.616386 0.416094 0.072445 0.1401672 0.665673 0.836688 0.733966 0.310229 a b c d2 0.665673 0.836688 0.733966 0.3102290 0.616386 0.416094 0.072445 0.1401673 0.405777 0.090530 0.991211 0.7123121 0.263227 0.079205 0.520708 0.866316
根据索引排序 .sort_index()
df = pd.DataFrame(np.random.rand(16).reshape(4,4), index=[2,1,3,0], columns=["a", "b", "c", "d"])print(df)print("")print(df.sort_index()) # 默认升序print("")print(df.sort_index(ascending=False)) # 降序
a b c d2 0.669311 0.118176 0.635512 0.2483881 0.752321 0.935779 0.572554 0.2740193 0.701334 0.354684 0.592998 0.4026860 0.548317 0.966295 0.191219 0.307908 a b c d0 0.548317 0.966295 0.191219 0.3079081 0.752321 0.935779 0.572554 0.2740192 0.669311 0.118176 0.635512 0.2483883 0.701334 0.354684 0.592998 0.402686 a b c d3 0.701334 0.354684 0.592998 0.4026862 0.669311 0.118176 0.635512 0.2483881 0.752321 0.935779 0.572554 0.2740190 0.548317 0.966295 0.191219 0.307908
df = pd.DataFrame(np.random.rand(16).reshape(4,4), index=["x", "z", "y", "t"], columns=["a", "b", "c", "d"])print(df)print("")print(df.sort_index()) # 根据字母顺序表排序
a b c dx 0.717421 0.206383 0.757656 0.720580z 0.969988 0.551812 0.210200 0.083031y 0.956637 0.759216 0.350744 0.335287t 0.846718 0.207411 0.936231 0.891330 a b c dt 0.846718 0.207411 0.936231 0.891330x 0.717421 0.206383 0.757656 0.720580y 0.956637 0.759216 0.350744 0.335287z 0.969988 0.551812 0.210200 0.083031
df = pd.DataFrame(np.random.rand(16).reshape(4,4), index=["three", "one", "four", "two"], columns=["a", "b", "c", "d"])print(df)print("")print(df.sort_index()) # 根据单词首字母排序
a b c dthree 0.173818 0.902347 0.106037 0.303450one 0.591793 0.526785 0.101916 0.884698four 0.685250 0.364044 0.932338 0.668774two 0.240763 0.260322 0.722891 0.634825 a b c dfour 0.685250 0.364044 0.932338 0.668774one 0.591793 0.526785 0.101916 0.884698three 0.173818 0.902347 0.106037 0.303450two 0.240763 0.260322 0.722891 0.634825
(1)获取更多优质内容及精彩资讯,可前往:https://www.cda.cn/?seo
(2)了解更多数据领域的优质课程:
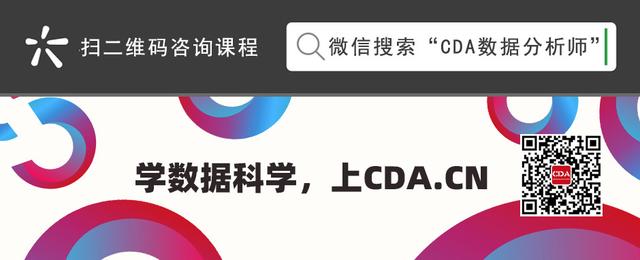