使用keras进行二分类时,常使用binary_crossentropy作为损失函数。那么它的原理是什么,跟categorical_crossentropy、sparse_categorical_crossentropy有什么区别?在进行文本分类时,如何选择损失函数,有哪些优化损失函数的方式?本文将从原理到实现进行一一介绍。
参考: https:// towardsdatascience.com/ understanding-binary-cross-entropy-log-loss-a-visual-explanation-a3ac6025181a
binary_crossentropy
原理
假设我们想做一个二分类,输入有10个点:
x = [-2.2, -1.4, -0.8, 0.2, 0.4, 0.8, 1.2, 2.2, 2.9, 4.6]
输出有两类,分别为红色、绿色:

我们可以将问题描述成“这个点是绿色的吗?”,或者“这个点是绿色的概率是多少?”。理想情况下,绿点的概率是1.0,而红点的(是绿色的)概率是0.0。从而,绿色就是正样本,红色就是负样本。
如果我们拟合一个模型来执行这种分类,它将预测我们每个点的绿色概率。那么我们如何评估预测概率的好坏呢?这就是损失函数的意义,
Binary CrossEntorpy的计算如下:

其中y是标签(1代表绿色点,0代表红色点),p(y)是所有N个点都是绿色的预测概率。看到这个计算式,发现对于每一个绿点(y=1)它增加了log(p(y))的损失(概率越大,增加的越小),也就是它是绿色的概率。下面我们可视化地看一下这个损失函数。
假设我们训练一个逻辑回归模型来进行分类,那么训练出的函数趋近于一个sigmoid曲线,曲线上每个点表示对于每个x是绿色点的概率:
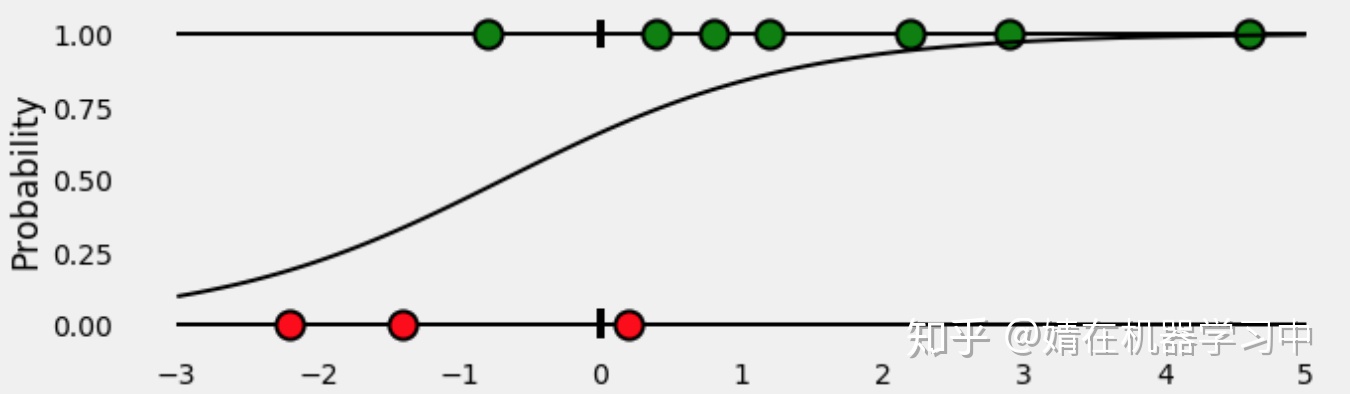
那么对于这些绿色的点,他们预测为绿色的概率是多少呢?实际下面图片中绿色的bar:
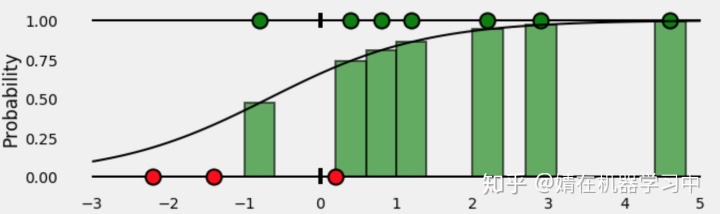
那么红色点预测为红色的概率是多少呢?实际就是下面图片中红色的bar:
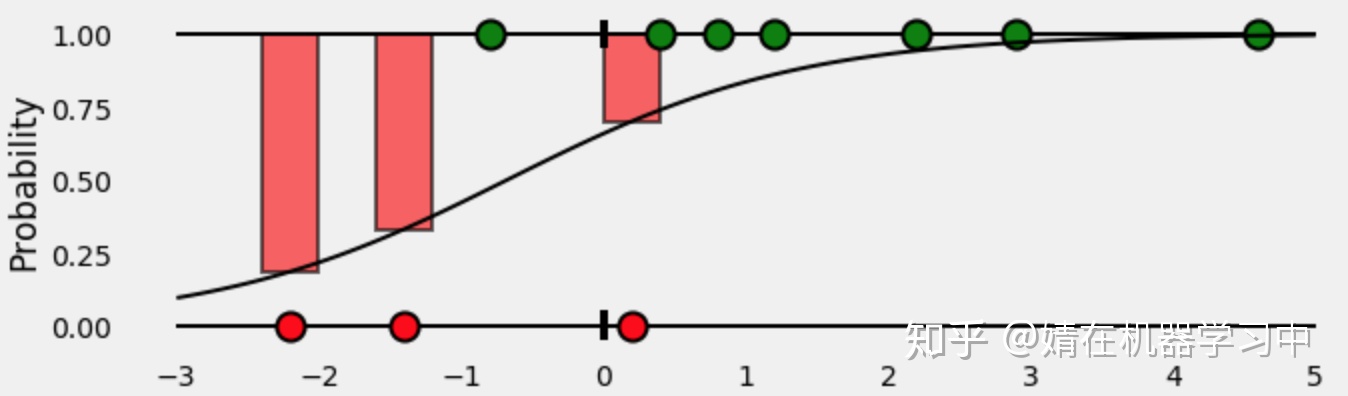
我们把图片绘制得更好看一下,如下图:
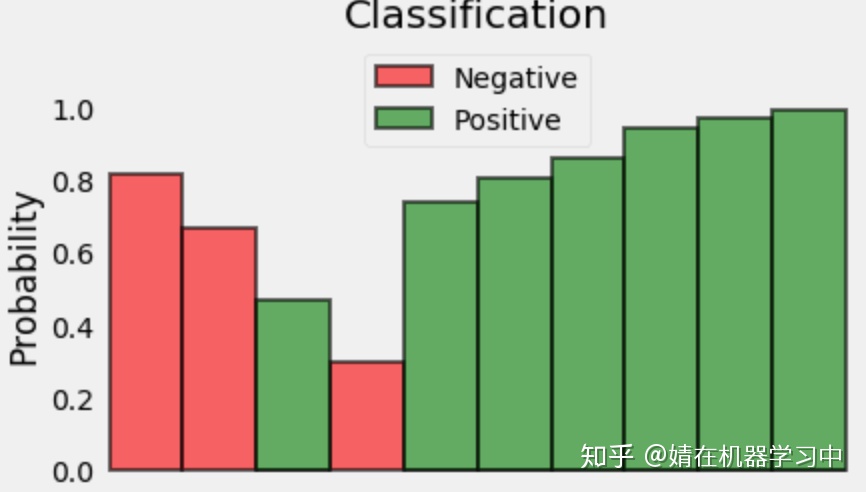
因为我们要计算损失,我们需要惩罚错误的预测。如果与正例相关的概率是1.0,我们需要它的损失为零。相反,如果概率很低,比如0.01,我们需要它的损失是巨大的!取概率的(负)对数非常适合我们的目的(由于0.0和1.0之间的值的对数是负的,我们取负对数来获得正的损失值)。下面这个图展示了当正例的概率逐渐趋近于0时loss的变化:
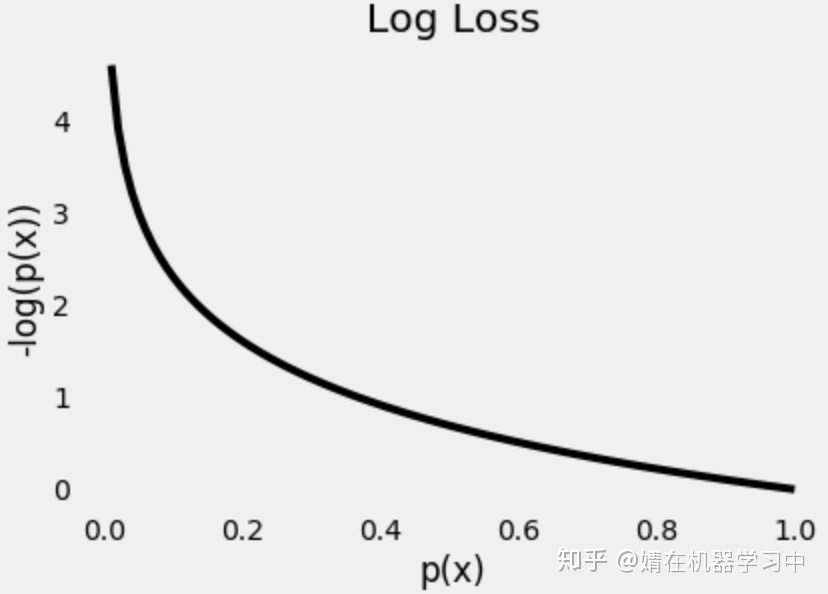
下面这个图表示了,我们使用负对数时每个点的损失,我们计算其平均值,就是binary cross entropy了!
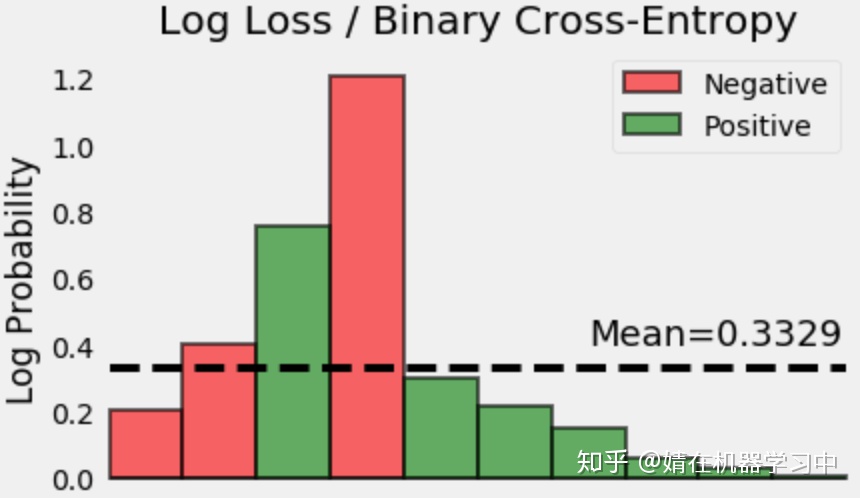
keras实现
tf2.1的bce用法如下:
bce = tf.keras.losses.BinaryCrossentropy()
loss = bce([0., 0., 1., 1.], [1., 1., 1., 0.])
print('Loss: ', loss.numpy()) # Loss: 11.522857
或者:
model = tf.keras.Model(inputs, outputs)
model.compile('sgd', loss=tf.keras.losses.BinaryCrossentropy())
具体实现如下(tensorflow.python.keras/losses):
class BinaryCrossentropy(LossFunctionWrapper):
def __init__(self, from_logits=False,
label_smoothing=0,
reduction=losses_utils.ReductionV2.AUTO,
name='binary_crossentropy'):
super(BinaryCrossentropy, self).__init__(
binary_crossentropy,
name=name,
reduction=reduction,
from_logits=from_logits,
label_smoothing=label_smoothing)
self.from_logits = from_logits
def binary_crossentropy(y_true, y_pred, from_logits=False, label_smoothing=0):
y_pred = ops.convert_to_tensor_v2(y_pred)
y_true = math_ops.cast(y_true, y_pred.dtype)
label_smoothing = ops.convert_to_tensor_v2(label_smoothing, dtype=K.floatx())
def _smooth_labels():
return y_true * (1.0 - label_smoothing) + 0.5 * label_smoothing
y_true = smart_cond.smart_cond(label_smoothing, _smooth_labels, lambda: y_true)
return K.mean(K.binary_crossentropy(y_true, y_pred, from_logits=from_logits), axis=-1)
在上面代码中,如果from_logits=True,则认为y_predit是tensor(可以认为是[0,1]之间的概率值),使用from_logits=True可以更稳定一些。label_smoothing在[0,1]之间。reduction的默认值是AUTO,表示根据上下文确定;如果是SUM_OVER_BATCH_SIZE表示整个batch的结果相加。
其中K.binary_crossentropy实现如下:
def binary_crossentropy(target, output, from_logits=False):
if from_logits:
return nn.sigmoid_cross_entropy_with_logits(labels=target, logits=output)
if not isinstance(output, (ops.EagerTensor, variables_module.Variable)):
output = _backtrack_identity(output)
if output.op.type == 'Sigmoid':
assert len(output.op.inputs) == 1
output = output.op.inputs[0]
return nn.sigmoid_cross_entropy_with_logits(labels=target, logits=output)
epsilon_ = _constant_to_tensor(epsilon(), output.dtype.base_dtype)
output = clip_ops.clip_by_value(output, epsilon_, 1. - epsilon_)
bce = target * math_ops.log(output + epsilon())
bce += (1 - target) * math_ops.log(1 - output + epsilon())
return -b
sigmoid_cross_entropy_with_logits实现如下:(该函数适用于不同类标签之间相互独立的情况,例如一个图片可以既包含大象也包含狗)
def sigmoid_cross_entropy_with_logits(_sentinel=None, labels=None, logits=None, name=None):
zeros = array_ops.zeros_like(logits, dtype=logits.dtype)
cond = (logits >= zeros)
relu_logits = array_ops.where(cond, logits, zeros)
neg_abs_logits = array_ops.where(cond, -logits, logits)
return math_ops.add(relu_logits - logits * labels, math_ops.log1p(math_ops.exp(neg_abs_logits)), name=name)
对于上面代码,解释如下:
对于x=logits, z=labels,logistic损失定义为
z * -log(sigmoid(x)) + (1 - z) * -log(1 - sigmoid(x))
= z * -log(1 / (1 + exp(-x))) + (1 - z) * -log(exp(-x) / (1 + exp(-x)))
= z * log(1 + exp(-x)) + (1 - z) * (-log(exp(-x)) + log(1 + exp(-x)))
= z * log(1 + exp(-x)) + (1 - z) * (x + log(1 + exp(-x))
= (1 - z) * x + log(1 + exp(-x))
= x - x * z + log(1 + exp(-x))
对于x<0,为了防止exp(-x)溢出:
x - x * z + log(1 + exp(-x))
= log(exp(x)) - x * z + log(1 + exp(-x))
= - x * z + log(1 + exp(x))
为了保证稳定和不溢出,在实现过程中使用了如下等式:
max(x, 0) - x * z + log(1 + exp(-abs(x)))
categorical_crossentropy
原理
CrossEntropy可用于多分类任务,且label且one-hot形式。它的计算式如下:
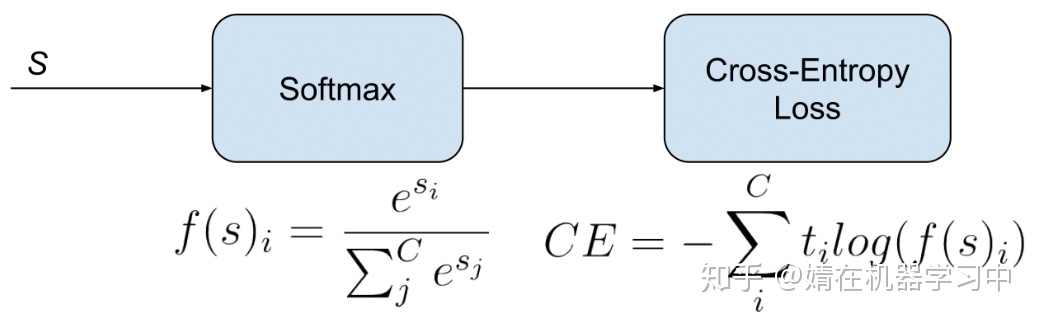
keras实现
tf2.1的ce用法如下:
y_true = [[0, 1, 0], [0, 0, 1]]
y_pred = [[0.05, 0.95, 0], [0.1, 0.8, 0.1]]
cce = tf.keras.losses.CategoricalCrossentropy()
# Using 'auto'/'sum_over_batch_size' reduction type.
cce(y_true, y_pred).numpy()
# Calling with 'sample_weight'.
cce(y_true, y_pred, sample_weight=tf.constant([0.3, 0.7])).numpy()
# Using 'sum' reduction type.
cce = tf.keras.losses.CategoricalCrossentropy(reduction=tf.keras.losses.Reduction.NONE)
cce(y_true, y_pred).numpy()
# Usage with the `compile` API
model = tf.keras.Model(inputs, outputs)
model.compile('sgd', loss=tf.keras.losses.CategoricalCrossentropy())
具体实现如下(tensorflow.python.keras/losses):
class CategoricalCrossentropy(LossFunctionWrapper):
def __init__(self,
from_logits=False,
label_smoothing=0,
reduction=losses_utils.ReductionV2.AUTO,
name='categorical_crossentropy'):
super(CategoricalCrossentropy, self).__init__(
categorical_crossentropy,
name=name,
reduction=reduction,
from_logits=from_logits,
label_smoothing=label_smoothing)
def categorical_crossentropy(y_true,
y_pred,
from_logits=False,
label_smoothing=0):
y_pred = ops.convert_to_tensor_v2(y_pred)
y_true = math_ops.cast(y_true, y_pred.dtype)
label_smoothing = ops.convert_to_tensor_v2(label_smoothing, dtype=K.floatx())
def _smooth_labels():
num_classes = math_ops.cast(array_ops.shape(y_true)[1], y_pred.dtype)
return y_true * (1.0 - label_smoothing) + (label_smoothing / num_classes)
y_true = smart_cond.smart_cond(label_smoothing, _smooth_labels, lambda: y_true)
return K.categorical_crossentropy(y_true, y_pred, from_logits=from_logits)
其中K.categorical_crossentropy实现如下:
def categorical_crossentropy(target, output, from_logits=False, axis=-1):
if from_logits:
return nn.softmax_cross_entropy_with_logits_v2(
labels=target, logits=output, axis=axis)
if not isinstance(output, (ops.EagerTensor, variables_module.Variable)):
output = _backtrack_identity(output)
if output.op.type == 'Softmax':
output = output.op.inputs[0]
return nn.softmax_cross_entropy_with_logits_v2(
labels=target, logits=output, axis=axis)
# scale preds so that the class probas of each sample sum to 1
output = output / math_ops.reduce_sum(output, axis, True)
# Compute cross entropy from probabilities.
epsilon_ = _constant_to_tensor(epsilon(), output.dtype.base_dtype)
output = clip_ops.clip_by_value(output, epsilon_, 1. - epsilon_)
return -math_ops.reduce_sum(target * math_ops.log(output), axis)
sparse_categorical_crossentropy
原理
跟categorical_crossentropy的区别是其标签不是one-hot,而是integer。比如在categorical_crossentropy是[1,0,0],在sparse_categorical_crossentropy中是3.
keras实现
tf2.1中使用方法如下:
y_true = [1, 2]
y_pred = [[0.05, 0.95, 0], [0.1, 0.8, 0.1]]
loss = tf.keras.losses.sparse_categorical_crossentropy(y_true, y_pred)
其他技巧
focal loss
参考: https:// ldzhangyx.github.io/201 8/11/16/focal-loss/
Focal Loss的出现是为了解决训练集正负样本极度不平衡的情况,通过reshape标准交叉熵损失解决类别不均衡(Class Imbalance),这样它就能降低容易分类的样例的比重(Well-classified Examples)。这个方法专注训练在Hard Examples的稀疏集合上,能够防止大量的Easy Negatives在训练中压倒训练器。其公式为:

其中参数为0的时候,Focal Loss退化为交叉熵CE。当这个参数不同时,对loss的影响如下:
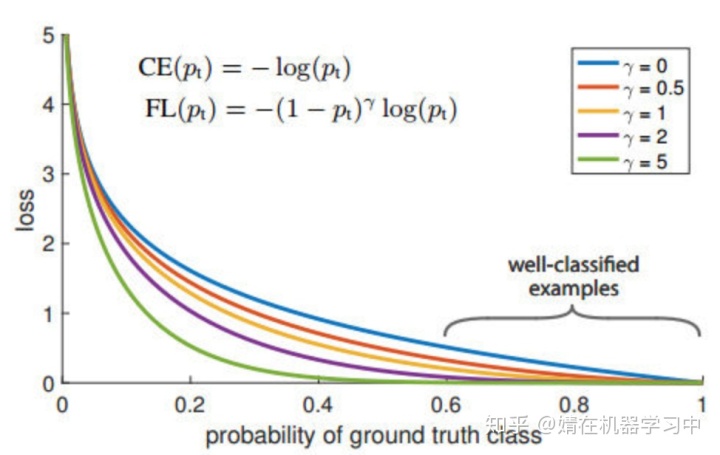
p_t越大,FL越小,其对总体loss所做的贡献就越小;反过来说,p_t越小(小于0.5的情况也就是被误分类),越能反映在总体loss上。
Focal Loss的tensorflow api:https://www.tensorflow.org/addons/api_docs/python/tfa/losses/SigmoidFocalCrossEntropy
label smooth
参考: https:// towardsdatascience.com/ what-is-label-smoothing-108debd7ef06
在使用深度学习模型进行分类任务时,我们通常会遇到以下问题:overfit和over confidence。Overfit问题得到了很好的研究,可以通过earlystop、dropout、正则化等方法来解决。另一方面,我们over confidence的工具较少。标签平滑是一种正则化技术,解决了这两个问题。
Label Smooth将y_hot和均匀分布的混合来代替一个hot编码的标签向量y_hot:

K是标签类的数目,α是一个决定平滑的超参数。如果α= 0,我们获得最初的一个原始的y_hot编码。如果α= 1,我们得到均匀分布。
当损失函数为交叉熵时,使用标签平滑,模型将softmax函数应用于倒数第二层的logit向量z,计算其输出概率p。在这种情况下,交叉熵损失函数相对于logit的梯度为:

其中y是标签分布,并且:
- 梯度下降会使p尽可能接近y
- 梯度在-1和1之间有界
一个标准的ont-hot希望有更大的logit gaps输入到里面。直观地说,较大的logit gap加上有界的梯度会使模型的自适应性降低,并且对其预测过于自信。相反,平滑的标签鼓励小的logit差距,可以得到更好的模型校准,并防止过度自信的预测。
下面我们使用一个例子说明:假设我们有K = 3类,我们的标签属于第一类。令[a, b, c]为logit向量。如果我们不使用标签平滑,那么标签向量就是一个one-hot向量[1,0,0]。我们的模型将a≫b和a≫c。例如,应用softmax分对数向量(10,0,0)给(0.9999,0,0)的4位小数。
如果我们使用标签的平滑与α= 0.1,平滑标签向量≈(0.9333,0.0333,0.0333)。logit向量[3.3322,0,0]在softmax之后将经过平滑处理的标签向量近似为小数点后4位,并且它的差距更小。这就是为什么我们称平滑标签为一种正则化技术,因为它可以防止最大的logit变得比其他的更大。
更形象地说,对于label_smoothing=0.2,则意味着标签0的概率是0.1,标签1的概率是0.9