ISLR(7)- 非线性回归分析
多项式回归和阶梯函数
Note Summary:
0.从理想的线性到现实的非线性
1.多项式回归
2.Step Function
3.参考
0. Moving Beyond Linearity
相较于其他模型, 线性模型更易于描述和实现
- 解释性能和推断理论更有优势
However, standard linear regression can have significant limitations in terms of predictive power
- Since the linearity assumption is always an poor approximation
- Recall that Least Squares can be improved by Ridge Regression, LASSO, PCR... to reduce the complexity of the linear model
- reduce the variance of the estimates
Goals Beyond Linearity:
Relax the Linearity assumption while
- still maintaining interpretability as much as possible
Extensions of linear models
- Polynomial Regression(7.1)
- Step Function(7.2)
- Regression Spline(7.4)
- Smoothing Spline(7.5)
- Local Regression(7.6)
- above approaches are for modeling the relationship between a response Y and a single predictor X in a flexible way.
- Generalized Additive Model (GAM)
- above approaches can be seamlessly integrated to model
and several
1. Polynomial Regression
❝ Polynomial Regression extends the linear model by adding extra predictors, obtained by raising each of the original predictors to a power:
- A Cubic regression uses three variables,
, as predictors to
❞
Standard Linear Model to Polynomial
- for large enough 「degree d」, polynomial regression produces an extremely non-linear curve
- the coefficients
are still estimated by Least Squre
- Genearlly, 「
」
Wage & Age Non-Linear Relation
Fitting a degree-4 polynomial using least squares
- the individual coefficients are not of particular interest (black box???)
- Let
be the value of
age
, to predictwage
:
「Variance」
Compute Variance of the fit,
- Variance Estimates for each of the fitted coefficients
from Least Squares
- The Covariances between pairs of coefficient estimates,
- Let
be the 5x5 covariance matrix of the
- Let
- Let
:
- As EACH reference point
, this computation is repeated and get the fitted curve and twice the standard error
The pair of dotted curves at both sides of the fit are (2x) standard error curves
- Since this (2x) quantity corresponds to an approximate 95% CI, for normally distributed error terms
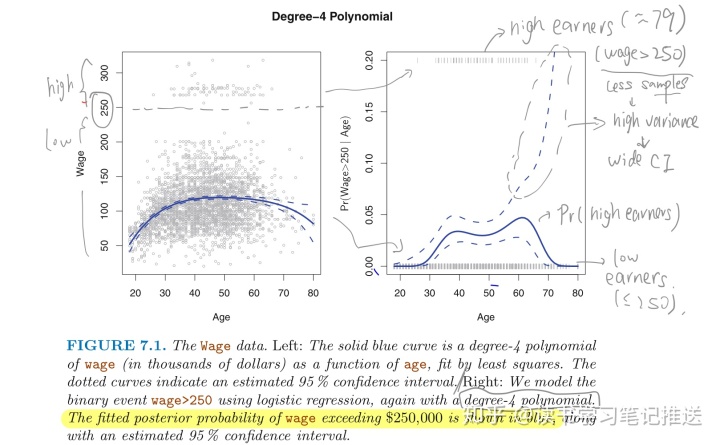
「Logsitic Regression」
We can treat Wage
as a binary variable by splitting it into 「high/low earners」
- logistic regression can be fitted to predict binary response:
Although the sample size is n = 3000, there are only 79 high earners,
- this results in a high variance in the estimated coefficients and therefore fairly wide confidence intervals
2. Step Function
Using polynomial functions in a linear model imposes a 「global structure」 on the non-linear function of X
- use step function to avoid such global structure
❝ Step Function cut the range of a variable into K distinct regions to produce a qualitative variable
- this has the effect of fitting a piecewise constant function in each bin
- and convert a continuous variable into ordered categorical variable
❞
Create cutpoints
Since
- Use Least Squares to fit a linear model by using
as predictors:
-
can be interpreted as the mean value of
for
-
can represent the average increase in the response for
in
relative to
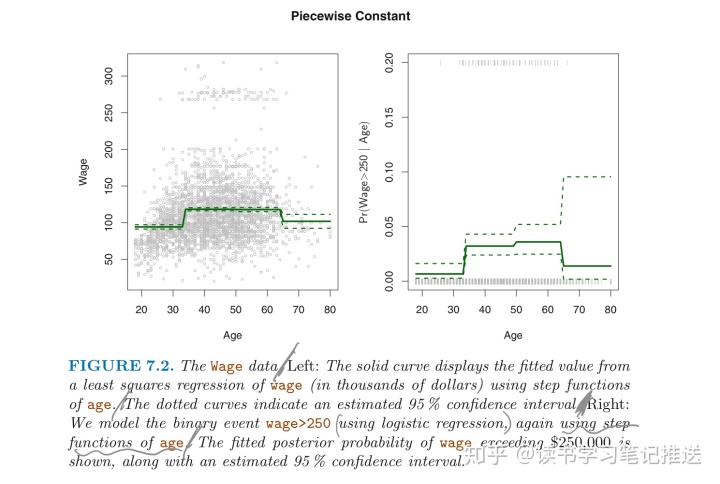
Fit the Logistic Regression Model to predict the probability:
「Disadvantages:」
Unless there are natural breakpoints in the predictors, piecewise-constant functions can 「miss the action」
age
from 20 to 30
「Advantages:」
Step functions are more likely used in biostatistics and epidemiology,
- 5-year age groups are often used to define the bins
3. 参考:
- 《Introduction to Statistical Learning》
- Section 7.1, 7.2
TOGO: (7) Basis Functions and Splines!