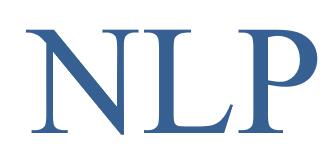
最近一直在分享自然语言推理和文本蕴含的相关论文,并且之前已经分享过三篇,分别是bilateral multi-perspective matching (BiMPM)模型、Enhanced Sequential Inference Model(ESIM)模型和Densely Interactive Inference Network(DIIN)模型。这次分享一下最经典的文本蕴含模型,也就是孪生网络(Siamese Network)。
刘聪NLP:论文阅读笔记:文本蕴含之BiMPMzhuanlan.zhihu.com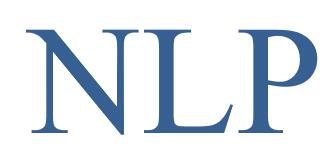
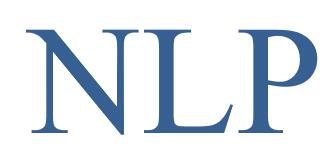
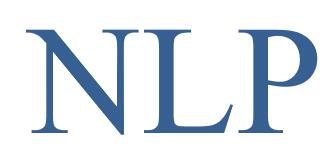
一、背景介绍
孪生网络一开始提出是在图像识别领域(例如人脸识别),来求解两张图片(两张人脸图像)相似度,判断两张图片是否相似。如下图所示1,输入两张图片,将两张图片经过同一个卷积神经网络,得到每张图片的向量表示,最后求解两个向量的编辑距离(可以是余弦距离,欧式距离,等等),根据得到的编辑距离判断两张图片是否相似。
孪生网络原文链接www.cs.utoronto.ca那么从哪里体现出孪生网络的“孪生”二字呢?从图1中,我们可以看到,其实每张图片是经过了各自的卷积神经网络,但是两个卷积神经网络的所有权值(所有参数)都是共享的,这就像一对双胞胎一样,因此我们管这种权值共享的网络称之为孪生网络。而权值共享的目的有两个:(1)减少参数量,减小模型的复杂度;(2)将两个不同空间维度的向量映射到同一个空间维度上,使其数据分布保持一致,在同一空间维度上对不同向量进行编码。
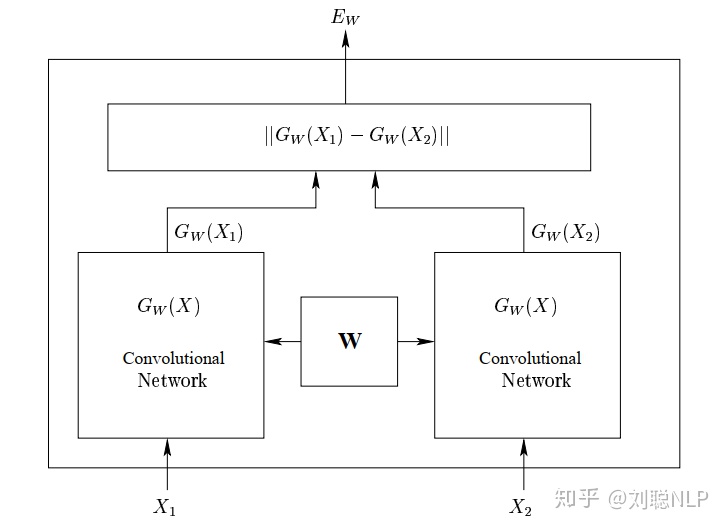
二、孪生网络在文本蕴含中的使用
在机器学习中,很多算法在图像和自然语言处理两个领域是可以互通的(可以相互借鉴的)。根据上一节的描述,我们可以看出,该任务和我们文本蕴含任务很像;都是输入两个不同的特征向量,最后判断两个特征向量之间的关系。如图2所示,我们将孪生网络应用在文本蕴含领域。
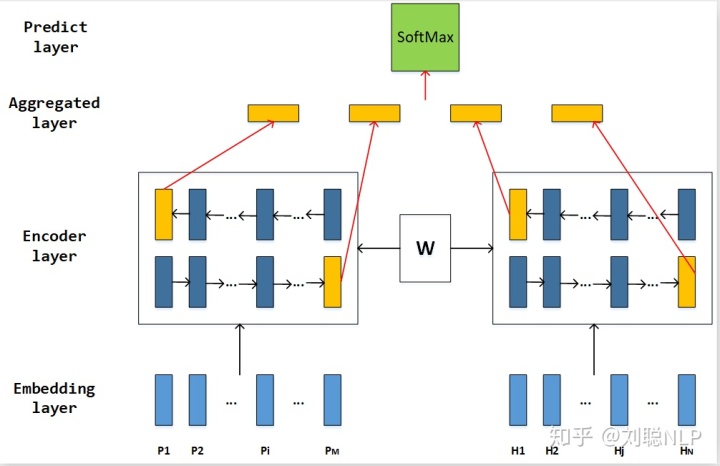
主要包含四层:词表示层(Embedding Layer)、编码层(Encoder Layer)、融合层(Aggregated Layer)和预测层(Predict Layer)。
下面通过代码详细介绍:
首先定义模型固定参数和其输入,其中forward()函数包含上文所提到的四层。
class SIAMESE(object):
def __int__(self, config, word_embedding_matrix=None):
self.word_embedding_dim = config.word_dim
self.rnn_hidden_size = config.rnn_hidden_size
self.fine_tune_embedding = config.fine_tune_embedding
self.num_sentence_words = config.max_length_content
self.learning_rate = config.learning_rate
self.rnn_layers = config.rnn_layers
with tf.variable_scope("input"):
self.sentence_one_word = tf.placeholder(tf.int32, [None, self.num_sentence_words], name="sentence_one_word")
self.sentence_two_word = tf.placeholder(tf.int32, [None, self.num_sentence_words], name="sentence_two_word")
self.y_true = tf.placeholder(tf.int32, [None, 2], name="true_labels")
self.is_train = tf.placeholder(tf.bool, [], name="is_train")
self.dropout_rate = tf.cond(self.is_train, lambda: config.dropout_rate, lambda: 0.0)
self.word_mat = tf.get_variable("word_mat",
initializer=tf.constant(word_embedding_matrix, dtype=tf.float32),
trainabel=self.fine_tune_embedding)
self.forward()
1、词表示层(Embedding Layer)
在这一层中,我们将在句子P和句子Q中的每个词映射成一个d维的向量,这d维的向量由word embedding组成,word embedding来自提前预训练好的词向量。
with tf.variable_scope("embedding_layer"):
word_embedded_sentence_one = tf.nn.embedding_lookup(self.word_mat, self.sentence_one_word)
word_embedded_sentence_two = tf.nn.embedding_lookup(self.word_mat, self.sentence_two_word)
2、编码层(Encoder Layer)
在这一层中,我们对上一层得到的词表示向量进行上下文编码,使用一个双向LSTM(权值共享)分别编码前提句和假设句的词表示向量,得到其上下文表征向量。
由于使用多层动态双向LSTM,因此首先获取每个句子的实际长度;
with tf.variable_scope("get_length_layer"):
self.batch_size = tf.shape(self.sentence_one_word)[0]
self.sentence_one_wordlevel_mask = tf.sign(self.sentence_one_word, name="sentence_one_wordlevel_mask")
self.sentence_two_wordlevel_mask = tf.sign(self.sentence_two_word, name="sentence_two_wordlevel_mask")
self.sentence_one_len = tf.reduce_sum(self.sentence_one_wordlevel_mask,1)
self.sentence_two_len = tf.reduce_sum(self.sentence_two_wordlevel_mask,1)
然后对其编码,得到双向LSTM最后一个time step作为是该句子的句子表征向量;
with tf.variable_scope("sequence_encoder_layer"):
one_fw_outputs, one_bw_outputs = self.my_bilstm(inputs=word_embedded_sentence_one,
dropout=self.dropout_rate,
n_layers=self.rnn_layers,
scope="bilstm",
sequence_length=self.sentence_one_len,
hidden_units=self.rnn_hidden_size)
two_fw_outputs, two_bw_outputs = self.my_bilstm(inputs=word_embedded_sentence_two,
dropout=self.dropout_rate,
n_layers=self.rnn_layers,
scope="bilstm",
sequence_length=self.sentence_two_len,
hidden_units=self.rnn_hidden_size,
reuse=True)
last_one_fw_output = self.last_relevant_output(one_fw_outputs, self.sentence_one_len)
last_one_bw_output = self.last_relevant_output(one_bw_outputs, self.sentence_one_len)
last_one_output = tf.concat([last_one_fw_output, last_one_bw_output], axis=-1)
last_two_fw_output = self.get_last_output(two_fw_outputs, self.sentence_two_len)
last_two_bw_output = self.get_last_output(two_bw_outputs, self.sentence_ontwo_len)
last_two_output = tf.concat([last_two_fw_output, last_two_bw_output], axis=-1)
多层动态双向LSTM代码如下:
def my_bilstn(self, inputs, dropout, n_layers, scope, sequence_length, hidden_size, reuse=None):
with tf.variable_scope("fw"+scope, reuse=reuse):
stacked_rnn_fw = []
for _ in range(n_layers):
fw_cell = LSTMCell(hidden_size)
lstm_fw_cell = DropoutWrapper(fw_cell, output_keep_prob=1-dropout)
stacked_rnn_fw.append(lstm_fw_cell)
lstm_fw_cell_m = tf.nn.rnn_cell.MultiRNNCell(cells=stacked_rnn_fw, state_is_tuple=True)
with tf.variable_scope("bw"+scope, reuse=reuse):
stacked_rnn_bw = []
for _ in range(n_layers):
bw_cell = LSTMCell(hidden_size)
lstm_bw_cell = DropoutWrapper(bw_cell, output_keep_prob=1-dropout)
stacked_rnn_bw.append(lstm_bw_cell)
lstm_bw_cell_m = tf.nn.rnn_cell.MultiRNNCell(cells=stacked_rnn_bw, state_is_tuple=True)
with tf.variable_scope(scope, reuse=reuse):
(fw_outputs, bw_outputs), _ = tf.nn.bidirectional_dynamic_rnn(cell_fw=lstm_fw_cell_m,
cell_bw=lstm_bw_cell_m,
inputs=inputs,
sequence_length=sequence_length,
dtype=tf.float32)
return fw_outputs, bw_outputs
获取动态双向LSTM最后一个time step向量代码如下:
def get_last_output(self, output, sequence_length):
with tf.name_scope("get_last_output"):
batch_size = tf.shape(output)[0]
max_length = tf.shape(output)[-2]
out_size = int(output.get_shape()[-1])
index = tf.range(0, batch_size)*max_length + (sequence_length-1)
flat = tf.reshape(output, [-1, out_size])
last_output = tf.gather(flat, index)
return last_output
3、融合层(Aggregated Layer)和预测层(Predict Layer)
在这一层中,我们将上文得到的两个句子的句子表征向量进行拼接([P,H,P*H,P-H]),然后通过两个全连接层去预测,两个句子是否存在蕴含关系。
with tf.variable_scope("output_layer"):
aggregated_representation = tf.concat([last_one_output, last_two_output, last_one_output*last_two_output, last_one_output-last_two_output], axis=-1)
predict_one_layer = tf.layers.dense(aggregated_representation,
units=aggregated_representation.get_shape().as_list()[1],
activation=tf.nn.tanh,
name="predict_one_layer")
d_predict_one_layer = tf.layers.dropout(predict_one_layer, rate=self.dropout_rate, training=self.is_train, name="predict_one_layer_dropout")
self.predict_two_layer = tf.layers.dense(d_predict_one_layer, units=2, name="predict_two_layer")
self.y_pred = tf.nn.softmax(self.predict_two_layer, name="scores")
self.predictions = tf.arg_max(self.y_pred, axis=1, name="predictions")
三、总结
本人认为,孪生网络的精髓就是两个编码器的参数共享,而孪生网络做文本蕴含应该是文本蕴含的开端;像是BiMPM、DIIN和ESIM,都是孪生网络的改进版,对其编码后的句子向量不是做简单的拼接,而是对其进行注意力提取和句子信息交互,使其结果更好。
以上就是我对孪生网络的理解,如果有不对的地方,请大家见谅并多多指教。