import numpy as np
import pandas as pd
import matplotlib.pyplot as plt
from matplotlib import gridspec
from sklearn.cluster import OPTICS, cluster_optics_dbscan
from sklearn.preprocessing import normalize, StandardScaler
X = pd.read_csv('Mall_Customers.txt', encoding = 'utf-8')
# Dropping irrelevant columns
drop_features = ['CustomerID', 'Gender']
X = X.drop(drop_features, axis = 1)
# Handling the missing values if any
X.fillna(method ='ffill', inplace = True)
X.head()
# Scaling the data to bring all the attributes to a comparable level
scaler = StandardScaler()
X_scaled = scaler.fit_transform(X)
# Normalizing the data so that the data
# approximately follows a Gaussian distribution
X_normalized = normalize(X_scaled)
# Converting the numpy array into a pandas DataFrame
X_normalized = pd.DataFrame(X_normalized)
# Renaming the columns
X_normalized.columns = X.columns
X_normalized.head()
# Building the OPTICS Clustering model
optics_model = OPTICS(min_samples = 10, xi = 0.05, min_cluster_size = 0.05)
# Training the model
optics_model.fit(X_normalized)
# Producing the labels according to the DBSCAN technique with eps = 0.5
labels1 = cluster_optics_dbscan(reachability = optics_model.reachability_,
core_distances = optics_model
optics聚类(代码)DBSCAN聚类比较
最新推荐文章于 2024-06-13 15:59:09 发布
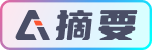