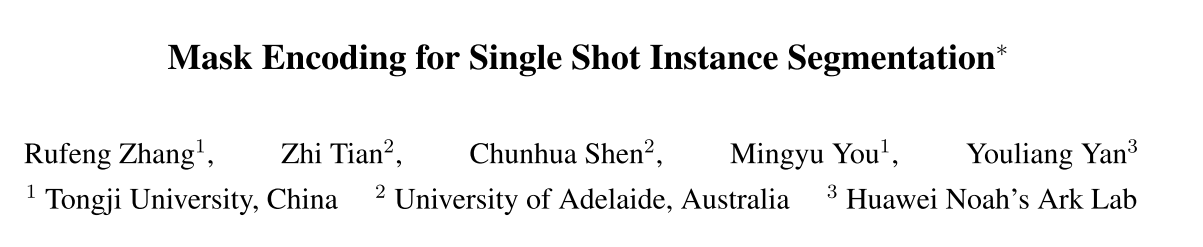
1. Motivation
- 单阶段的分割在mask AP上比不过Mask R-CNN。
one-stage alternatives cannot compete with Mask R-CNN in mask AP.
- 作者提出了一个假设:“Is it possible to predict the object mask in the intrinsic low-dimensional space and still achieve competitive accuracy?" 并给出了肯定的答复。
2. Contribution
- 作者提出了基于FCOS one-stage目标检测网络框架的实例分割网络MEInst。最大的创新点在于在off-line时,通过IPCA的方法将二维的实例mask压缩为一维紧凑的vector。利用了原始mask的redundancy并且更高效的进行重建。
- 实验表明,MEInst的精度比其他的one-stage来的高,和Mask RCNN competitive。
- We propose to encode a two-dimensional instance mask into a compact representation vector. The compressed vector, takes advantages of the redundancy in the original mask and proves to be effective and efficient for reconstruction.
- Encoding can be done with a few dictionary learning methods, including PCA, sparse coding, and auto- encoders. Here we show that even the simplest PCA already suffices for mask encoding.
- With this mask representation, a new framework is introduced for single shot instance segmentation, termed mask encoding based instance segmentation(MEInst), by extending FCOS [29] with a mask branch for mask coefficient regression. Actually, our mask encoding is completely independent of the mechanism of detectors, and it may be easily incorporated into other detectors.
3. Method
3.1 Network Architecture
相比较于原FCOS分支,MEInst添加了 Mask Regression Head,输出为HxWxN,其中N为vector的component(降维之后的维度)。
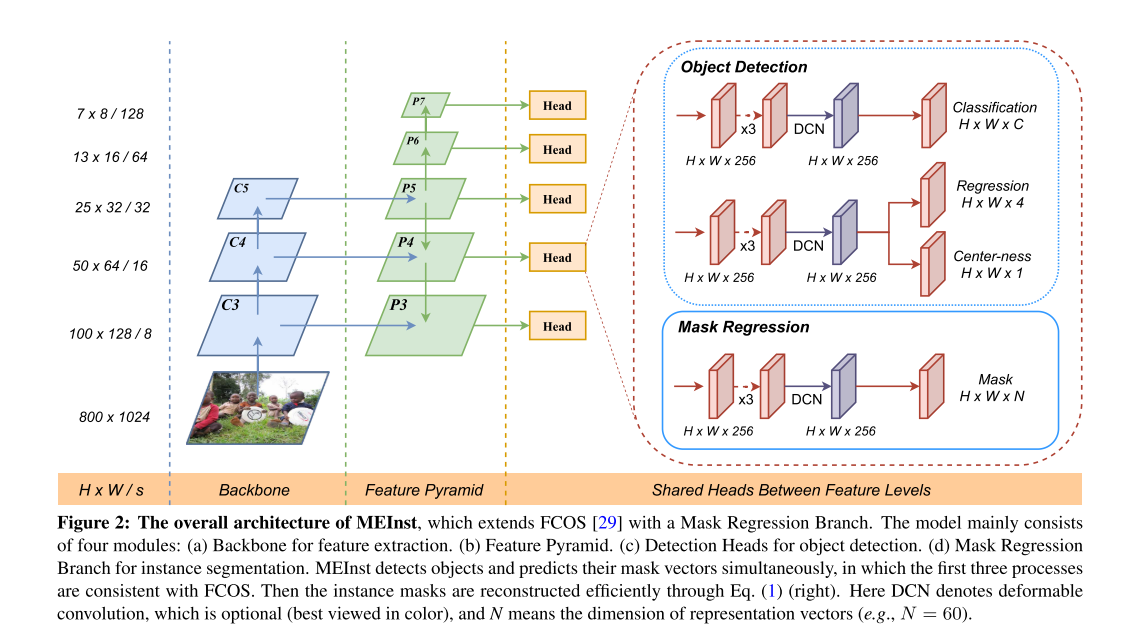
3.2 Mask Encoding
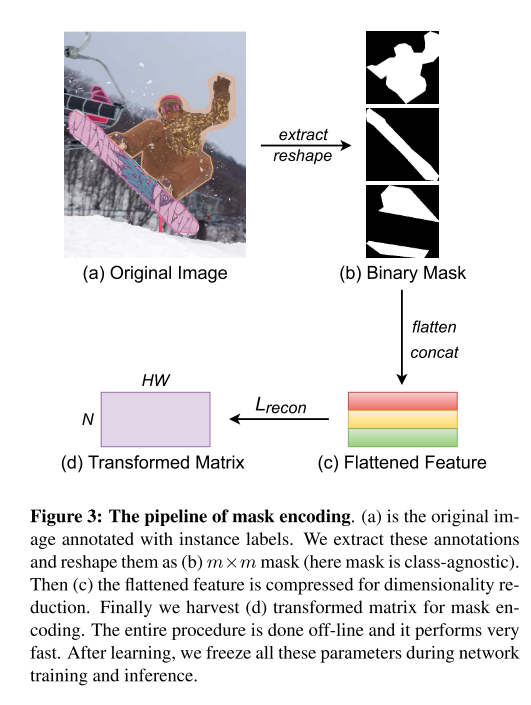
3.2.1 Compact Representation
v ∈ R N v \in R^{N} v∈RN, u ∈ R H