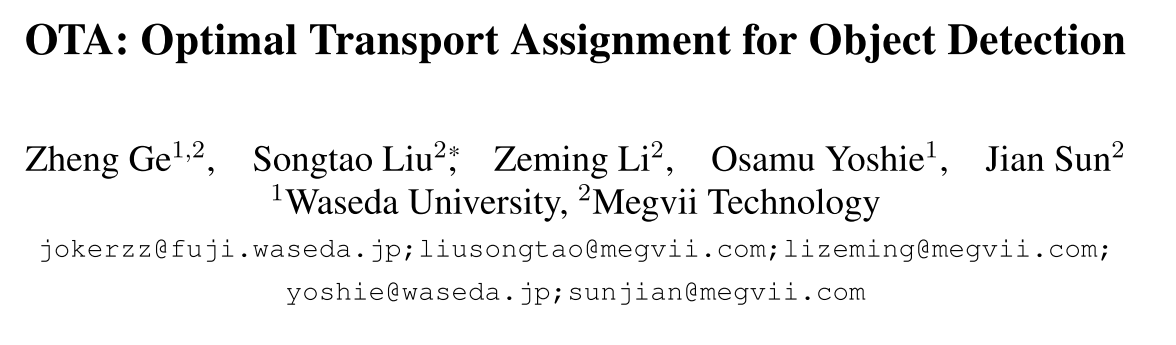
1. Motivation
-
DeTR [3] examines the idea of global optimal matching. But the Hungarian algo- rithm they adopted can only work in a one-to-one assign- ment manner.
-
One-to-Many 的方法。
So far, for the CNN based detectors in one-to-many scenarios, a global optimal assigning strategy remains uncharted.
-
Label Assignment
To train the detector, defining cls and reg targets for each anchor is a necessary procedure, which is called label assignment in object detection.
-
Such static strategies ignore a fact that for objects with different sizes, shapes or occlusion condition, the appropriate posi- tive/negative (pos/neg) division boundaries may vary.
文中认为对于ambiguous anchors的制定是非常重要的。
- Hence the assignment for ambiguous anchors is non-trivial and requires further information beyond the local view.
要将独立的最优分配转化为全局的最优分配。
- Thus a better assigning strategy should get rid of the convention of pursuing optimal assignment for each gt independently and turn to the ideology of global optimum, in other words, finding the global high confidence assignment for all gts in an image.
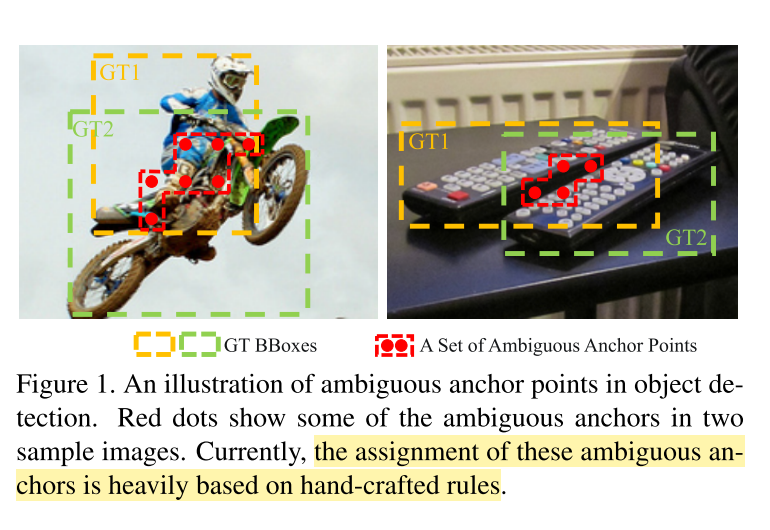
2. Contribution
相比于DETR的one-to–one Label Assignment,本文认为One-to-Many的Lbael Assignment同样可以对训练有帮助,也可以将制定带有global view的labels。
OT将anchor看做demander,将gt看做supplier。每一个gt供应positive label的数量看做为“每一个gt需要多少个positive anchor来完成训练过程,更好的收敛“。
OTA分别要求anchor与gt以及anchor与background pair-wise的loss,其中anchor与gt pair的transportation cost是cls和reg的loss,而anchor与background的pair-wise loss 只需要计算cls loss就好。
-
To achieve the global optimal assigning result under the one-to-many situation, we propose to formulate label as-signment as an Optimal Transport (OT) problem – a special form of Linear Programming (LP) in Optimization Theory.
-
we define each gt as a supplier who supplies a certain number of labels, and define each anchor as a de- mander who needs one unit label.
-
In this context, the number of positive labels each gt supplies can be interpreted as “how many positive anchors that gt needs for better convergence during the training process”.
-
The unit transportation cost between each anchor-gt pair is defined as the weighted summation of their pair-wise cls and reg losses.
-
The cost between background and a certain anchor is defined as their pair-wise classification loss only.
-
OTA also achieves the SOTA performance among one-stage detectors on a crowded pedestrian detection dataset named CrowdHu- man [35], showing OTA’s generalization ability on different detection benchmarks
3.Method
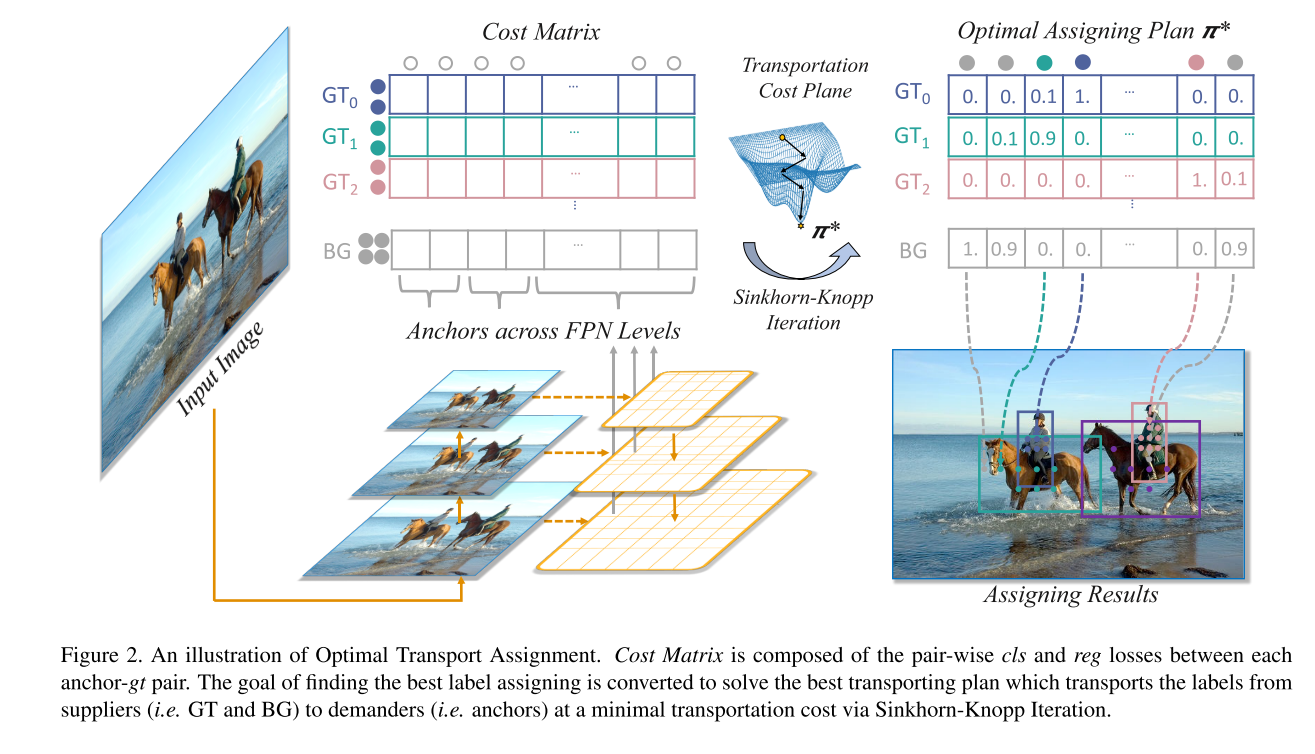
3.1. Optimal Transport
- Transporting cost for each unit of good from supplier i to demander j is denoted by c i j c_{ij} cij
- We thus address this issue by a fast iterative solution, named Sinkhorn-Knopp
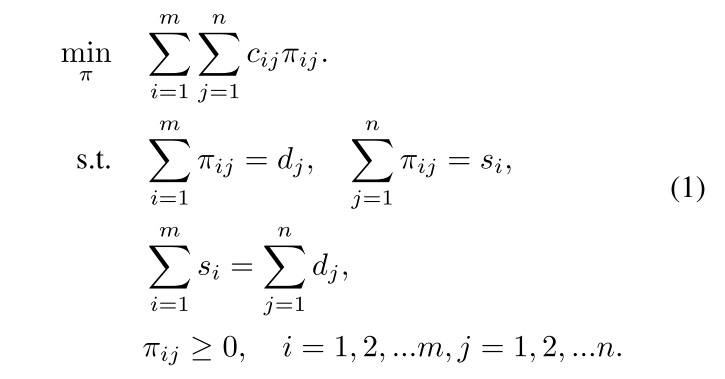
3.2 OT for Label Assignment
m gt targets and n anchors. 根据one-to-many的关系,一个supplier有多个unit(一个unit对应一个demander),一个demander(anchor)值对应一个supplier(gt)。
- we view each gt as a supplier who holds k units of positive labels ( s i = k s_i=k si=k, i = 1, 2, …, m)。
- each anchor as a demander who needs one unit of label(i.e. d j = 1 d_j = 1 dj=1, j= 1,2,…, n)。
c f g c^{fg} cfg前景cost的公式如下所示:(one unit)
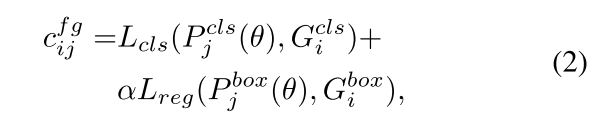
其中,Lcls和Lreg分别是cross entropy loss 和 IoU Loss(也可以被其他常用损失函数取代)。α是平衡参数。
对于 c b g c^{bg} cbg背景cost的公式如下所示:

negative labels的数量为 n − m × k n-m\times k n−m×k。 m × k m \times k m×k表示gt共有的所有units,而n表示anchor的个数,由于每一个unit就对应一个anchor(demander)。因此剩余的数量就要分配为negative labels。
c b g ∈ R