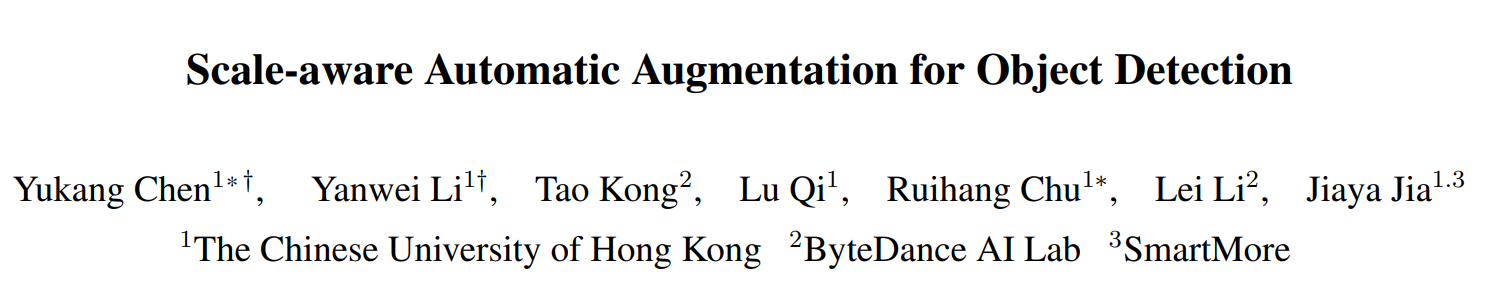
1. Motivation
-
这篇文章主要关注于目标检测中的数据增强。
This paper focuses on data augmentation for object detection.
-
之前的工作,对于如何将尺度适应性融入网络的方法主要来源与网络的结构(FPN)以及数据增强。
-
Previous work handles this challenge which brings the scale adaptation to the network efficiently mainly from two aspects, namely network architecture and data augmentation.
-
Though inspiring performance gain has achieved, these data augmentation strategies usually rely on heavy expert experience.
-
Thus, scale-aware property and efficiency issue are essential to address for searching augmentation in box-level tasks.
2. Contribution
-
In this paper, we propose a new way to automatically learn scale-aware data augmentation strategies for object detection and relevant box-level tasks.
-
We first introduce scale-awareness to the search space from two image-levels and box-levels.
For image-level augmentations, zoom-in and -out operations are included with their probabilities and zooming ratios for search.
For box-level augmentations, the augmenting areas are generalized with a new searchable parameter, i.e., area ratio.
3. Method
The designed scale-aware search space contains both image-level and box-level augmentations.
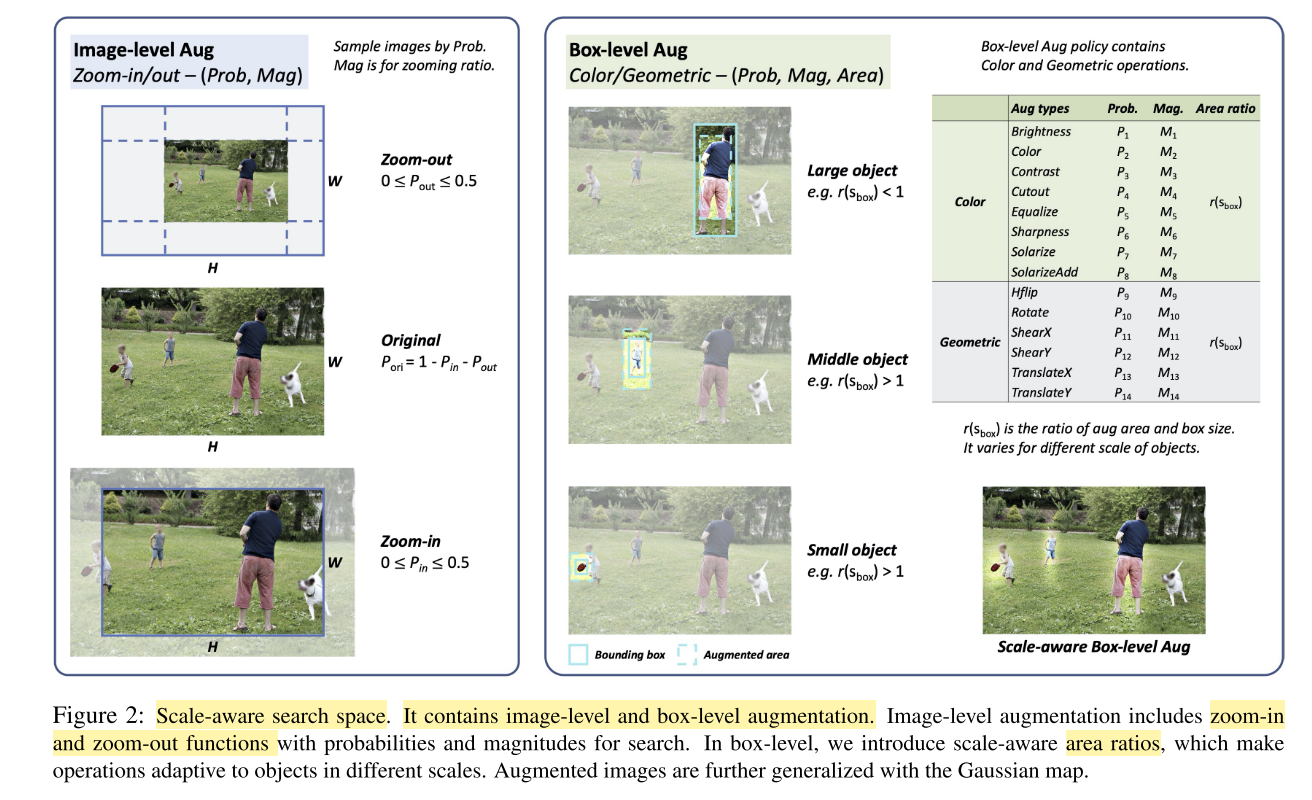
3.1 Scale-aware Search Space
3.1.1 Image-Level augmentations

3.1.2 Box-Level augmentations
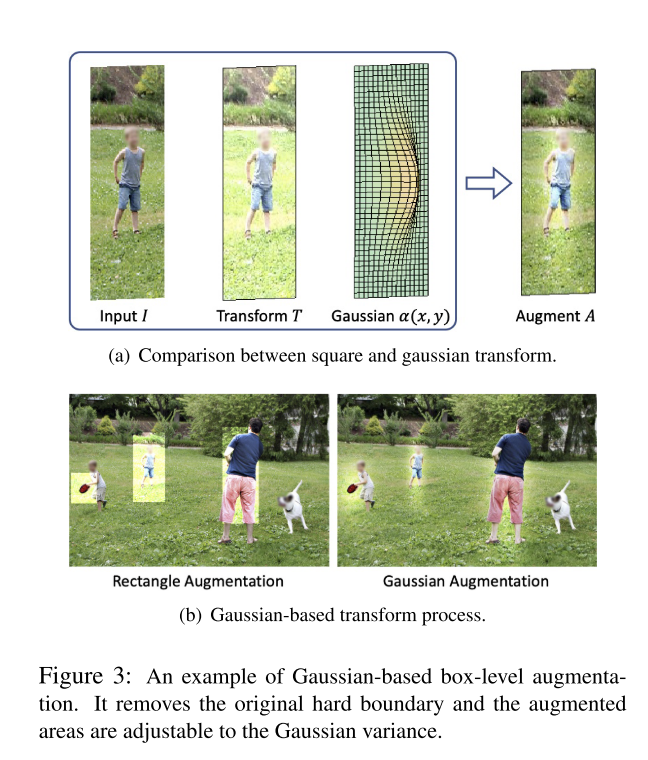
引入可学习的参数 area-ratio
- Different from [51], the proposed approach further