#-*- codeing = utf-8 -*-
#@Time :2021/5/17 10:33
#@Author :Onion
#@File :Convolution.py
#@Software :PyCharm
# 卷积神经网络Demo
# 步骤
# 1:定义输入变量
# 2:定义卷积核变量
# 3:定义卷积操作
# 4:运行卷积操作
import tensorflow as tf
tf.compat.v1.disable_eager_execution()
tf.compat.v1.disable_v2_behavior()
tf.compat.v1.disable_eager_execution()
tf.compat.v1.reset_default_graph()
# 1:定义输入变量
# shape的参数[batch, in_height, in_width, in_channels] [训练时一个batch的图片数量, 图片高度, 图片宽度, 图像通道数]
# 定义3个输入变量来模拟输入图片,分别是5*5大小1个通道,5*5大小2个通道,4*4大小1个通道的模拟数据
input1 = tf.compat.v1.Variable(tf.compat.v1.constant(1.0,shape=[1,5,5,1]))
input2 = tf.compat.v1.Variable(tf.compat.v1.constant(1.0,shape=[1,5,5,2]))
input3 = tf.compat.v1.Variable(tf.compat.v1.constant(1.0,shape=[1,4,4,1]))
# 2:定义卷积核变量
# shape的参数[filter_height, filter_width, in_channels, out_channels] [卷积核的高度,卷积核的宽度,图像通道数,卷积核个数]
# value以2*2个数据为单元,单元数图像通道数*卷积核个数
filter1 = tf.compat.v1.Variable(tf.compat.v1.constant(
Tensorflow(1.15.0)卷积操作
最新推荐文章于 2023-05-12 16:14:52 发布
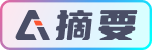