代码
import numpy as np
import matplotlib.pyplot as plt
from sklearn.tree import DecisionTreeRegressor
if __name__ == "__main__":
N = 400
x = np.random.rand(N) * 8 - 4
x.sort()
print('====================')
y1 = 16 * np.sin(x) ** 3 + np.random.randn(N)
y2 = 13 * np.cos(x) - 5 * np.cos(2*x) - 2 * np.cos(3*x) - np.cos(4*x) + 0.1* np.random.randn(N)
np.set_printoptions(suppress=True)
y = np.vstack((y1, y2)).T
print(y)
print('Data = \n', np.vstack((x, y1, y2)).T)
print('=================')
x = x.reshape(-1, 1)
deep = 8
reg = DecisionTreeRegressor(criterion='mse', max_depth=deep)
dt = reg.fit(x, y)
x_test = np.linspace(-4, 4, num=1000).reshape(-1, 1)
print(x_test)
y_hat = dt.predict(x_test)
print(y_hat)
plt.scatter(y[:, 0], y[:, 1], c='r', marker='s', s=60, label='Actual')
plt.scatter(y_hat[:, 0], y_hat[:, 1], c='g', marker='o', edgecolors='g', s=30, label='Depth=%d' % deep, alpha=0.6)
plt.legend(loc='upper left')
plt.xlabel('y1')
plt.ylabel('y2')
plt.grid()
plt.show()
运行结果
[[ 6.63145077 -8.74451466]
[ 6.63145077 -8.74451466]
[ 6.63145077 -8.74451466]
...
[-6.04569282 -8.88572268]
[-6.04569282 -8.88572268]
[-6.04569282 -8.88572268]]
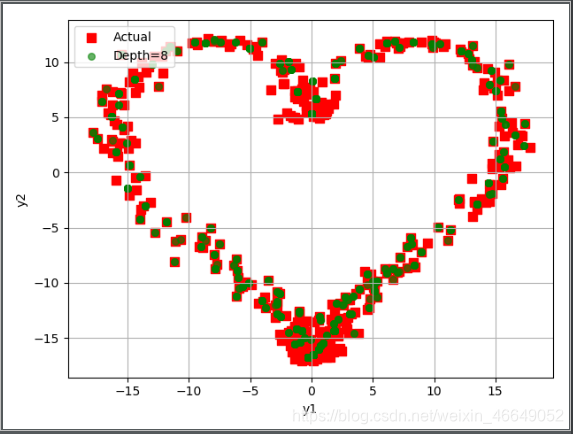